Popularity-based Aggregation and Bundle-specific Ranking for Bundle Recommendation
2023 6th International Conference on Artificial Intelligence and Big Data (ICAIBD)(2023)
摘要
Bundle Recommendation aims to recommend a bundle of items to users, which can greatly improve the recommendation efficiency of service providers and satisfy users’ diverse needs. In recent methods, the adoption of graph neural network and contrastive learning has made great progress. However, they ignore the contribution difference of items in a bundle. In addition, they use the conventional BPR(Bayesian Personalized Ranking) to create a user-specific ranking for a set of bundles, but neglect to rank users for each bundle. In this work, we propose a model named PABR(short for Popularity-based Aggregation and Bundle-specific Ranking for Bundle Recommendation). PABR adopts weighted aggregation based on the popularity of items and performs Bayesian Personalized Ranking from user-specific perspective and bundle-specific perspective. Experiments on three real-world datasets demonstrate that our method achieves improvement compared with baselines.
更多查看译文
关键词
bundle recommendation,weighted aggregation,contrastive learning,graph neural network
AI 理解论文
溯源树
样例
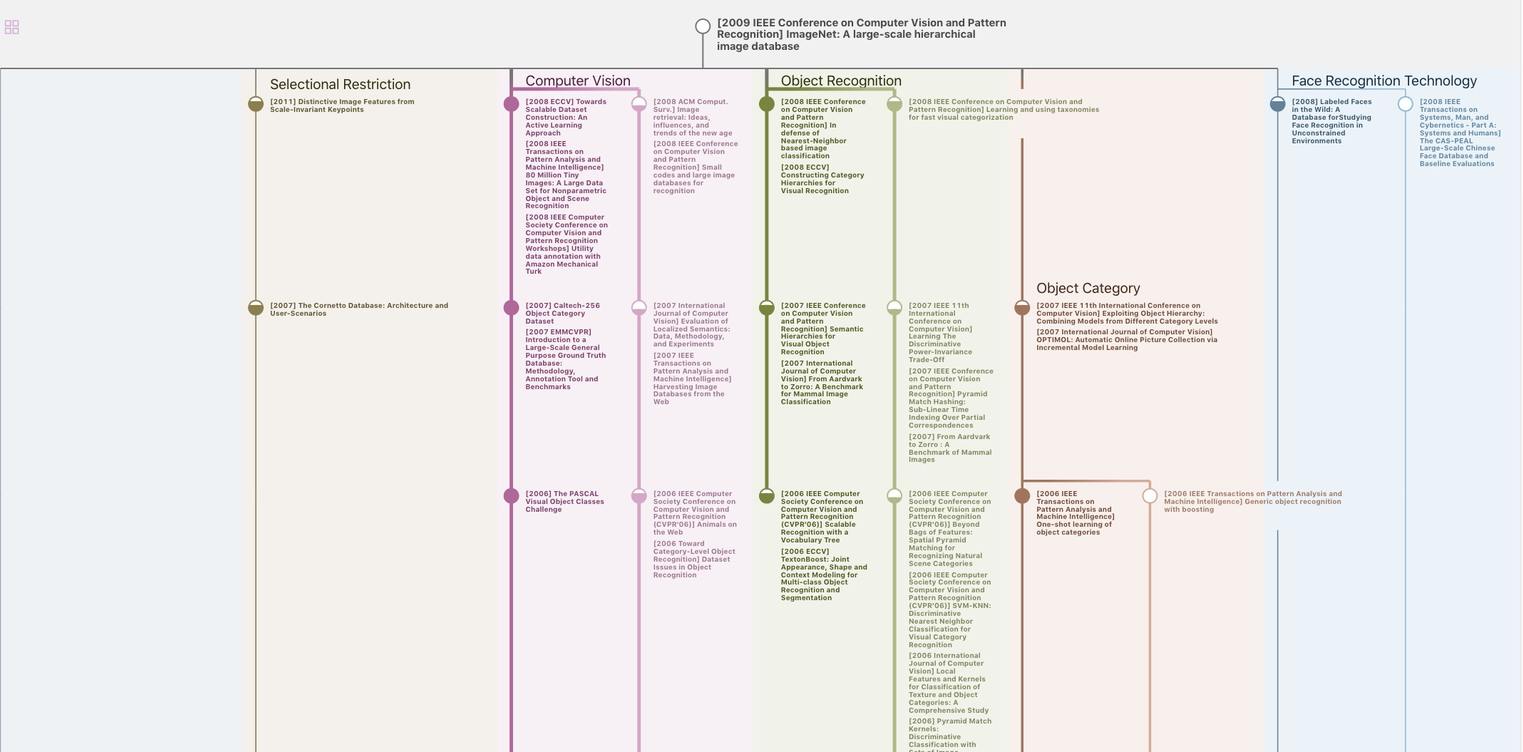
生成溯源树,研究论文发展脉络
Chat Paper
正在生成论文摘要