Hyperspectral Image Classification Combining Superpixel Principal Component Analysis Dimensionality Reduction with Extended Random Walk Probability Optimization
Laser & Optoelectronics Progress(2023)
摘要
A hyperspectral image classification method based on joint hyperpixel dimension reduction and category posterior probability optimization is proposed to address the issues of inadequate utilization of spatial information and decline in classification accuracy under small- and medium-sized training samples. First, the entropy rate hyperpixel segmentation algorithm, based on the spatial texture structure of hyperspectral images was used to adaptively detect homogeneous hyperpixel regions, and principal component analysis was applied to each region individually to mine the hyperpixel mixture features that can represent the spatial -spectral information of images. Then, the initial category probability vector of each pixel was calculated using the mixed features provided in the support vector machine, and the extended random walk algorithm was used to optimize the initial category using the image space neighborhood information. Finally, the classification result was calculated based on the maximum classification probability of each pixel. Experiments were performed on three general hyperspectral datasets, including Indian Pines, Pavia University, and Salinas, and compared with the other six methods. Even with a small number of training samples, the experimental results show that the proposed method's overall classification accuracy is 98. 29%, 97. 29%, and 99. 72%, respectively, which is superior to the classification results of the comparison methods.
更多查看译文
关键词
image processing,hyperspectral image classification,superpixel dimensionality reduction,extended random walk,support vector machine
AI 理解论文
溯源树
样例
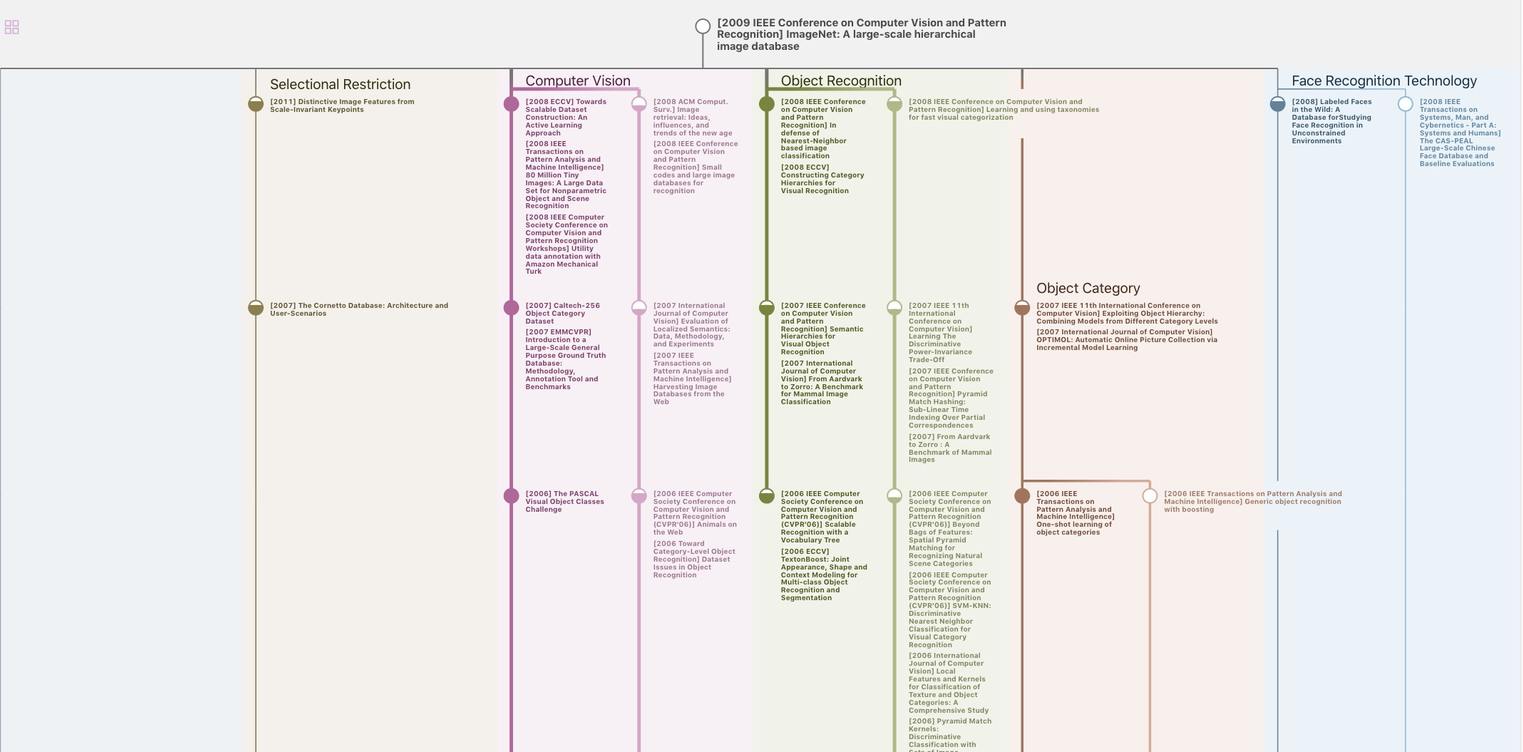
生成溯源树,研究论文发展脉络
Chat Paper
正在生成论文摘要