CompTLL-UNet: Compressed Domain Text-Line Localization in Challenging Handwritten Documents Using Deep Feature Learning from JPEG Coefficients.
CoRR(2023)
摘要
Automatic localization of text-lines in handwritten documents is still an open and challenging research problem. Various writing issues such as uneven spacing between the lines, oscillating and touching text, and the presence of skew become much more challenging when the case of complex handwritten document images are considered for segmentation directly in their respective compressed representation. This is because, the conventional way of processing compressed documents is through decompression, but here in this paper, we propose an idea that employs deep feature learning directly from the JPEG compressed coefficients without full decompression to accomplish text-line localization in the JPEG compressed domain. A modified U-Net architecture known as Compressed Text-Line Localization Network (CompTLL-UNet) is designed to accomplish it. The model is trained and tested with JPEG compressed version of benchmark datasets including ICDAR2017 (cBAD) and ICDAR2019 (cBAD), reporting the state-of-the-art performance with reduced storage and computational costs in the JPEG compressed domain.
更多查看译文
关键词
handwritten documents,deep feature learning,comptll-unet,text-line
AI 理解论文
溯源树
样例
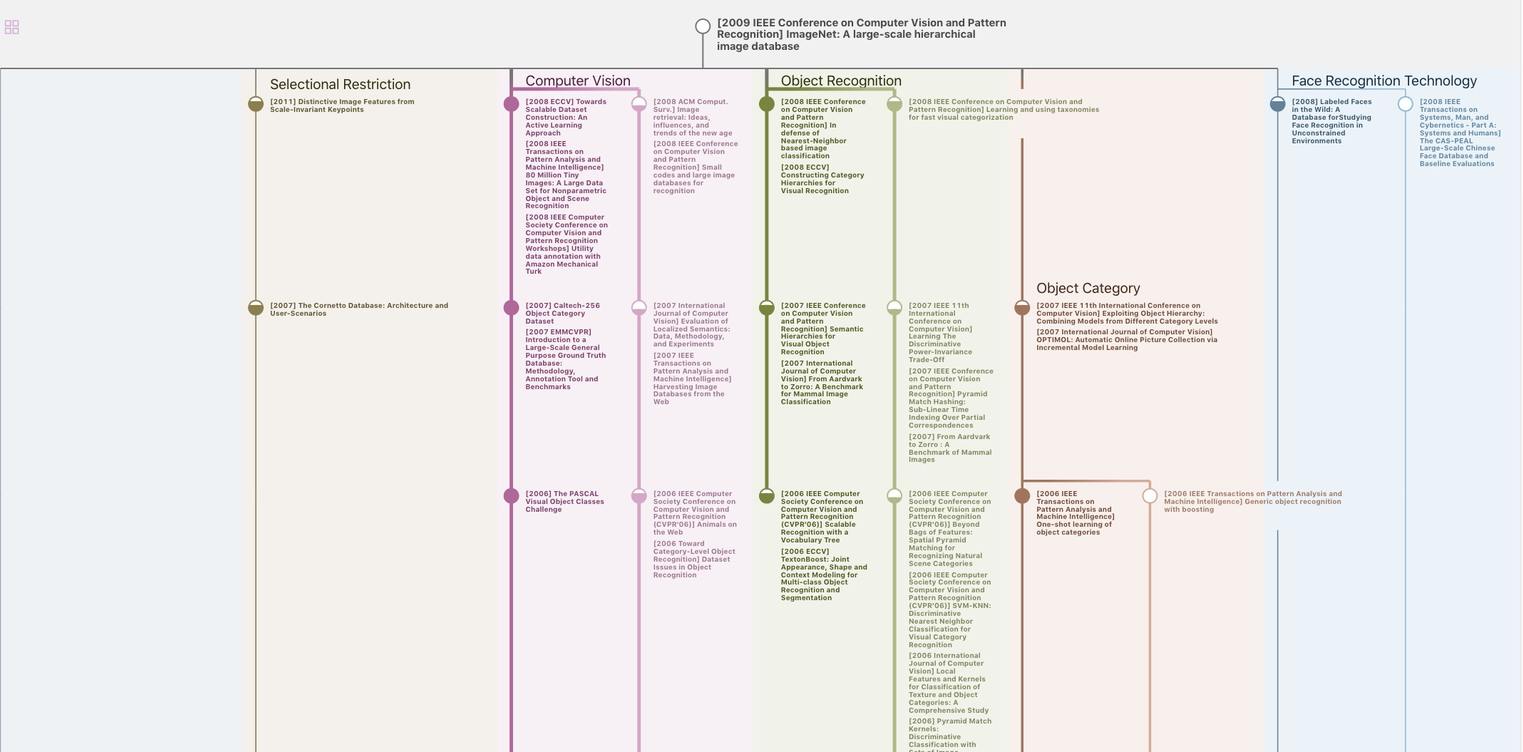
生成溯源树,研究论文发展脉络
Chat Paper
正在生成论文摘要