MATH: A Deep Learning Approach in QSAR for Estrogen Receptor Alpha Inhibitors.
Molecules (Basel, Switzerland)(2023)
摘要
Breast cancer ranks as the second leading cause of death among women, but early screening and self-awareness can help prevent it. Hormone therapy drugs that target estrogen levels offer potential treatments. However, conventional drug discovery entails extensive, costly processes. This study presents a framework for analyzing the quantitative structure-activity relationship (QSAR) of estrogen receptor alpha inhibitors. Our approach utilizes supervised learning, integrating self-attention Transformer and molecular graph information, to predict estrogen receptor alpha inhibitors. We established five classification models for predicting these inhibitors in breast cancer. Among these models, our proposed MATH model achieved remarkable precision, recall, F1 score, and specificity, with values of 0.952, 0.972, 0.960, and 0.922, respectively, alongside an ROC AUC of 0.977. MATH exhibited robust performance, suggesting its potential to assist pharmaceutical and health researchers in identifying candidate compounds for estrogen alpha inhibitors and guiding drug discovery pathways.
更多查看译文
关键词
qsar,estrogen receptor,deep learning approach,inhibitors
AI 理解论文
溯源树
样例
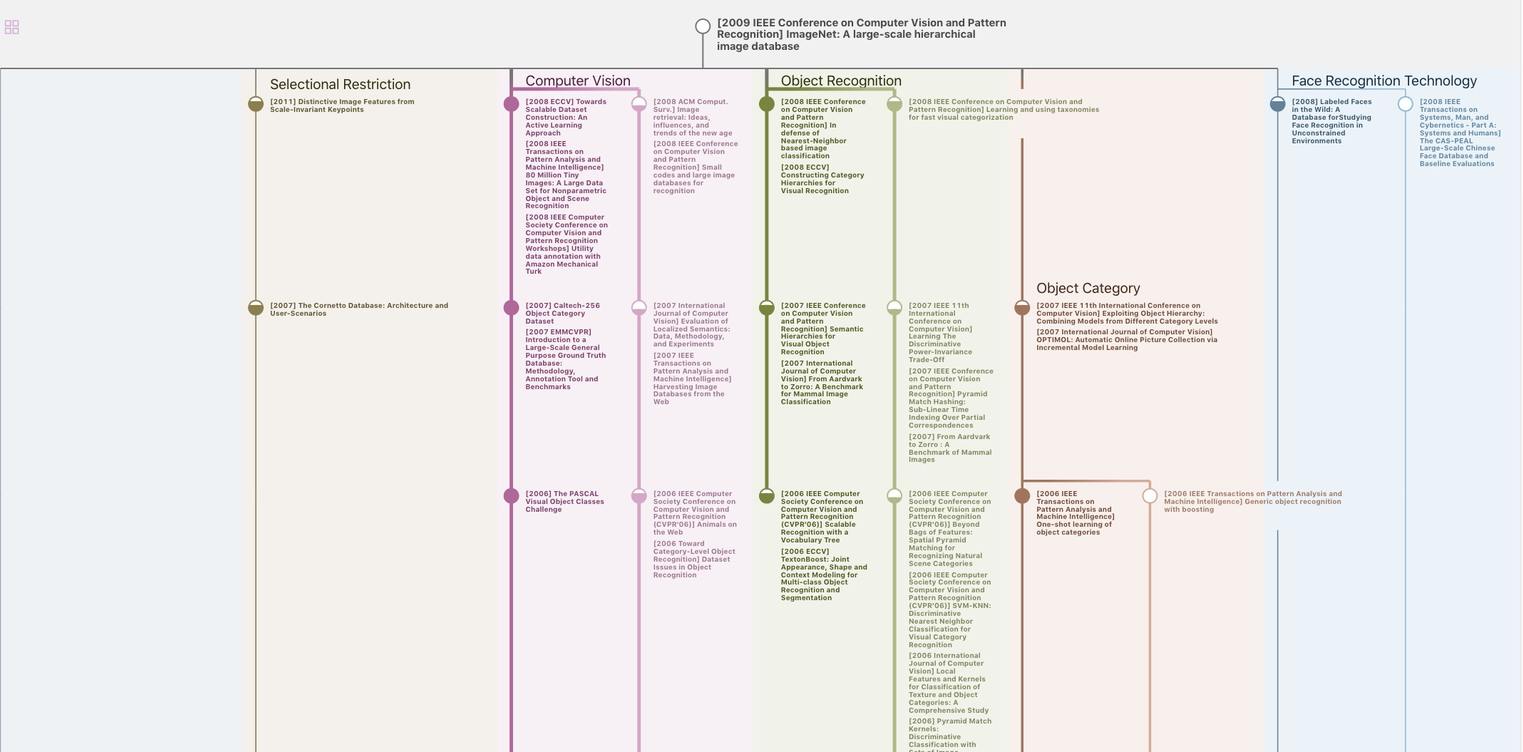
生成溯源树,研究论文发展脉络
Chat Paper
正在生成论文摘要