MUE-CoT: multi-scale uncertainty entropy-aware co-training framework for left atrial segmentation.
Physics in medicine and biology(2023)
摘要
Objective.Accurate left atrial segmentation is the basis of the recognition and clinical analysis of atrial fibrillation. Supervised learning has achieved some competitive segmentation results, but the high annotation cost often limits its performance. Semi-supervised learning is implemented from limited labeled data and a large amount of unlabeled data and shows good potential in solving practical medical problems.Approach. In this study, we proposed a collaborative training framework for multi-scale uncertain entropy perception (MUE-CoT) and achieved efficient left atrial segmentation from a small amount of labeled data. Based on the pyramid feature network, learning is implemented from unlabeled data by minimizing the pyramid prediction difference. In addition, novel loss constraints are proposed for co-training in the study. The diversity loss is defined as a soft constraint so as to accelerate the convergence and a novel multi-scale uncertainty entropy calculation method and a consistency regularization term are proposed to measure the consistency between prediction results. The quality of pseudo-labels cannot be guaranteed in the pre-training period, so a confidence-dependent empirical Gaussian function is proposed to weight the pseudo-supervised loss.Main results.The experimental results of a publicly available dataset and an in-house clinical dataset proved that our method outperformed existing semi-supervised methods. For the two datasets with a labeled ratio of 5%, the Dice similarity coefficient scores were 84.94% ± 4.31 and 81.24% ± 2.4, the HD95values were 4.63 mm ± 2.13 and 3.94 mm ± 2.72, and the Jaccard similarity coefficient scores were 74.00% ± 6.20 and 68.49% ± 3.39, respectively.Significance.The proposed model effectively addresses the challenges of limited data samples and high costs associated with manual annotation in the medical field, leading to enhanced segmentation accuracy.
更多查看译文
关键词
left atrial image segmentation, uncertainty, co-training, entropy
AI 理解论文
溯源树
样例
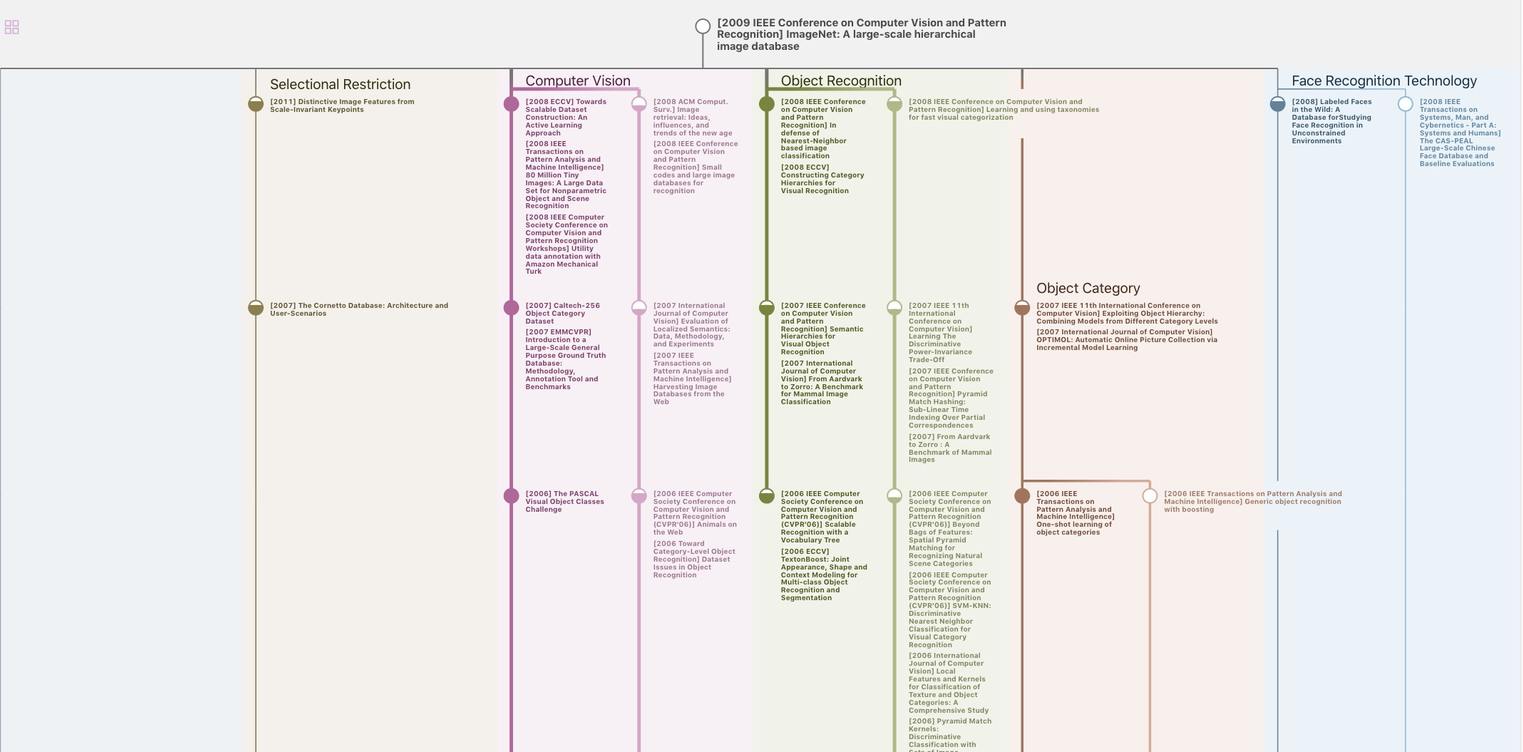
生成溯源树,研究论文发展脉络
Chat Paper
正在生成论文摘要