Automatic International Classification of Diseases Coding via Note-Code Interaction Network with Denoising Mechanism.
Journal of computational biology : a journal of computational molecular cell biology(2023)
摘要
Clinical notes are comprehensive files containing explicit information about a patient's visit. However, accurately assigning medical codes from clinical documents can be a persistent challenge due to the complexity of clinical data and the vast range of medical codes. Moreover, the large volume of medical records, the noisy medical records, and the uneven quality of coders all negatively impact the quality of the final codes. Deep learning technology has recently been integrated into automatic International Classification of Diseases (ICD) coding tasks to improve accuracy. Nevertheless, the imbalanced class problem, the complexness of code associations, and the noise in lengthy records still restrict the advancement of ICD coding tasks in deep learning. Thus, we present the Note-code Interaction Denoising Network (NIDN) that employs the self-attention mechanism to pull critical semantic features in electronic medical records (EMRs). Our model utilizes the label attention mechanism for retaining code-specific text expression. We introduce Clinical Classifications Software coding for multitask learning, capturing the functional relationships of medical coding to oblige in model prediction. To minimize the impact of noise on model prediction and improve the label distribution imbalance, a denoising module is introduced to filter noise. Our practical consequences indicate that the model NIDN exceeds competitive models on a third version of Medical Information Mart for Intensive Care data set.
更多查看译文
关键词
diseases coding,automatic international classification,note-code
AI 理解论文
溯源树
样例
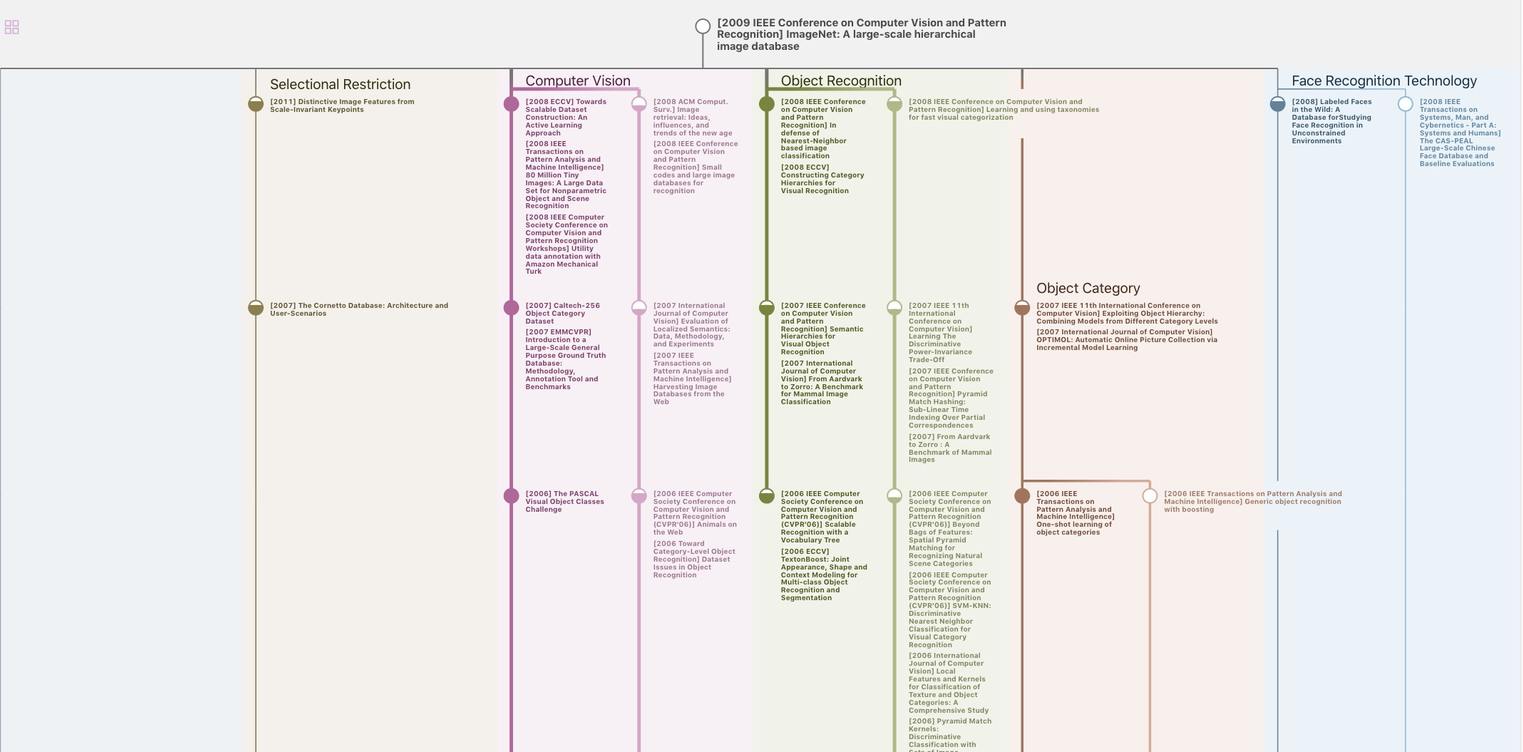
生成溯源树,研究论文发展脉络
Chat Paper
正在生成论文摘要