Resource Allocation of NOMA Communication Systems for Federated Learning
IEEE Communications Letters(2023)
摘要
Federated learning is a new communication and computing concept that allows naturally distributed data sets (e.g., as in data acquisition sensors) to be used to train global models, and therefore successfully addresses privacy, power and bandwidth limitations in wireless networks. In this letter, we study the communications problem of latency minimization of a multi-user wireless network used to train a decentralized machine learning model. To facilitate low latency, the wireless stations (WSs) employ non-orthogonal multiple access (NOMA) for simultaneous transmission of local model parameters to the base station, subject to the users' maximum CPU frequency, maximum transmit power, and maximum available energy. The proposed resource allocation scheme guarantees fair resource sharing among WSs by enforcing only a single WS to spend the maximum allowable energy or transmit at maximum power, whereas the rest of the WSs transmit at lower power and spend less energy. The closed-form analytical solution for the optimal values of resource allocation parameters is used for efficient online implementation of the proposed scheme with low computational complexity.
更多查看译文
关键词
computational complexity. Index Terms-Wireless federated learning,non-orthogonal multiple access,latency minimization
AI 理解论文
溯源树
样例
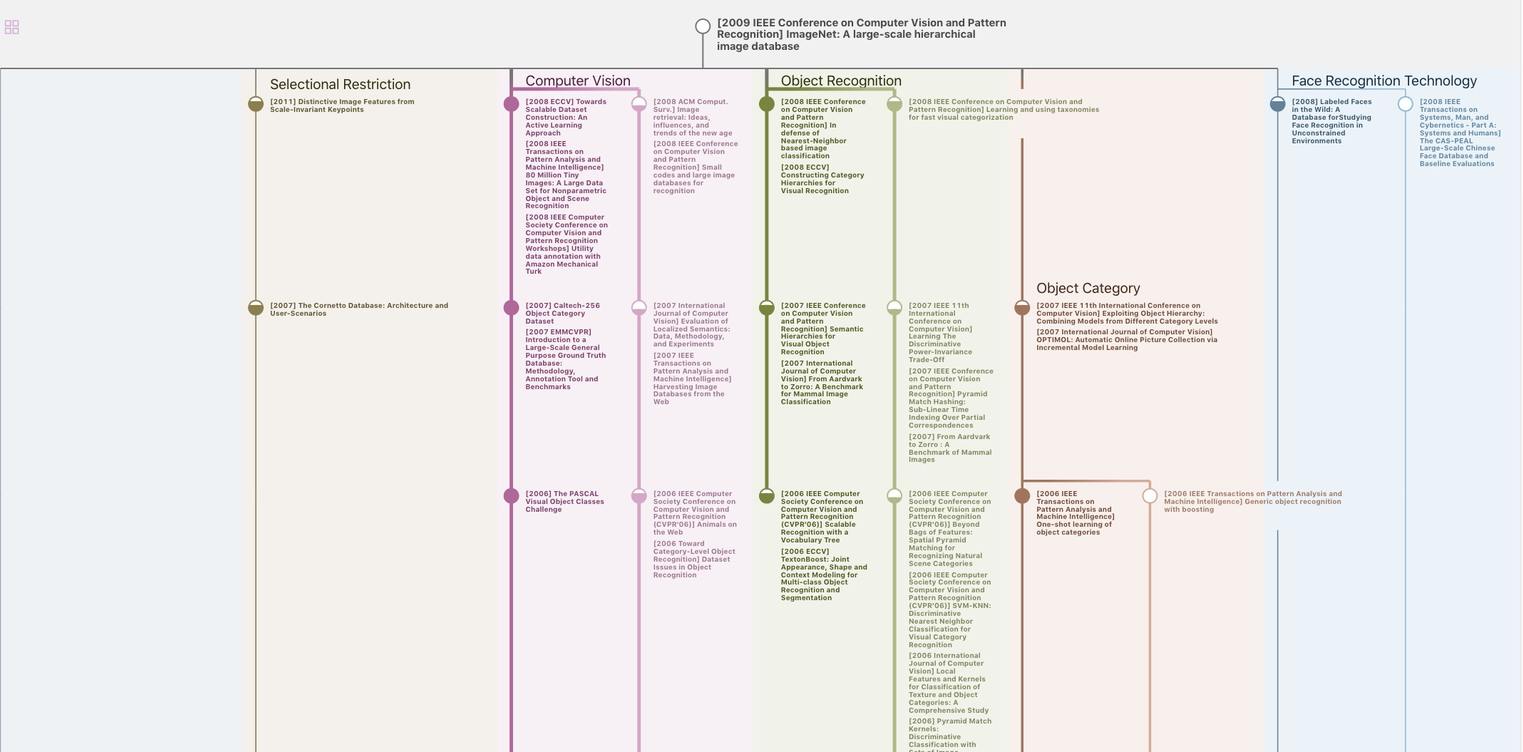
生成溯源树,研究论文发展脉络
Chat Paper
正在生成论文摘要