A Self-Supervised Learning-Based Channel Estimation for IRS-Aided Communication Without Ground Truth
IEEE Transactions on Wireless Communications(2023)
摘要
Deep learning (DL) is an emerging paradigm for accurate channel estimation for intelligent reflecting surface (IRS)-aided wireless communication systems. It has been proven to be a promising way to achieve better channel estimation performance for the IRS-aided wireless communication system than traditional methods (e.g., least-square algorithm). However, existing DL-based methods rely on ground truth (labels of the true channels) which is difficult to obtain in real networks. In this paper, we propose a self-supervised learning (SSL) method for the IRS channel estimation problem. No ground truth channel is needed in the training, while a simple and novel self-supervised denoising formula without a clean reference signal is presented. Particularly, in the training phase, the self-supervised signal and the input are the received signal vector and its noisy version, respectively. While in the inference phase the input is the estimated channel by using the least-square method and the output is the refined channel estimation. That is, our neural network-based channel estimation algorithm is not reciprocal for training and testing. We demonstrate that the proposed SSL solution has good convergence performance and generalization ability through numerical simulations. Interestingly, we find a “double descent” phenomenon in the learning curve during the test phase, i.e., when we gradually increase the number of training epochs, the performance first gets better, then becomes worse, and further gets better again. Besides, we propose to analyze SSL using the loss landscape and centered kernel alignment method. The results show that the self-supervised model has a similar loss landscape and representational similarity to the supervised model. We explored the effects of different signal-to-noise ratios (SNRs), different neural network sizes, and different training data volumes on our algorithm through numerical simulations. Extensive numerical simulation results show that our SSL algorithm is still competitive without ground truth. We also show that the developed scheme exhibits robustness to SNR ratio mismatch.
更多查看译文
关键词
Index Terms-Channel estimation, self-supervised learning, IRS, deep neural network, double descent
AI 理解论文
溯源树
样例
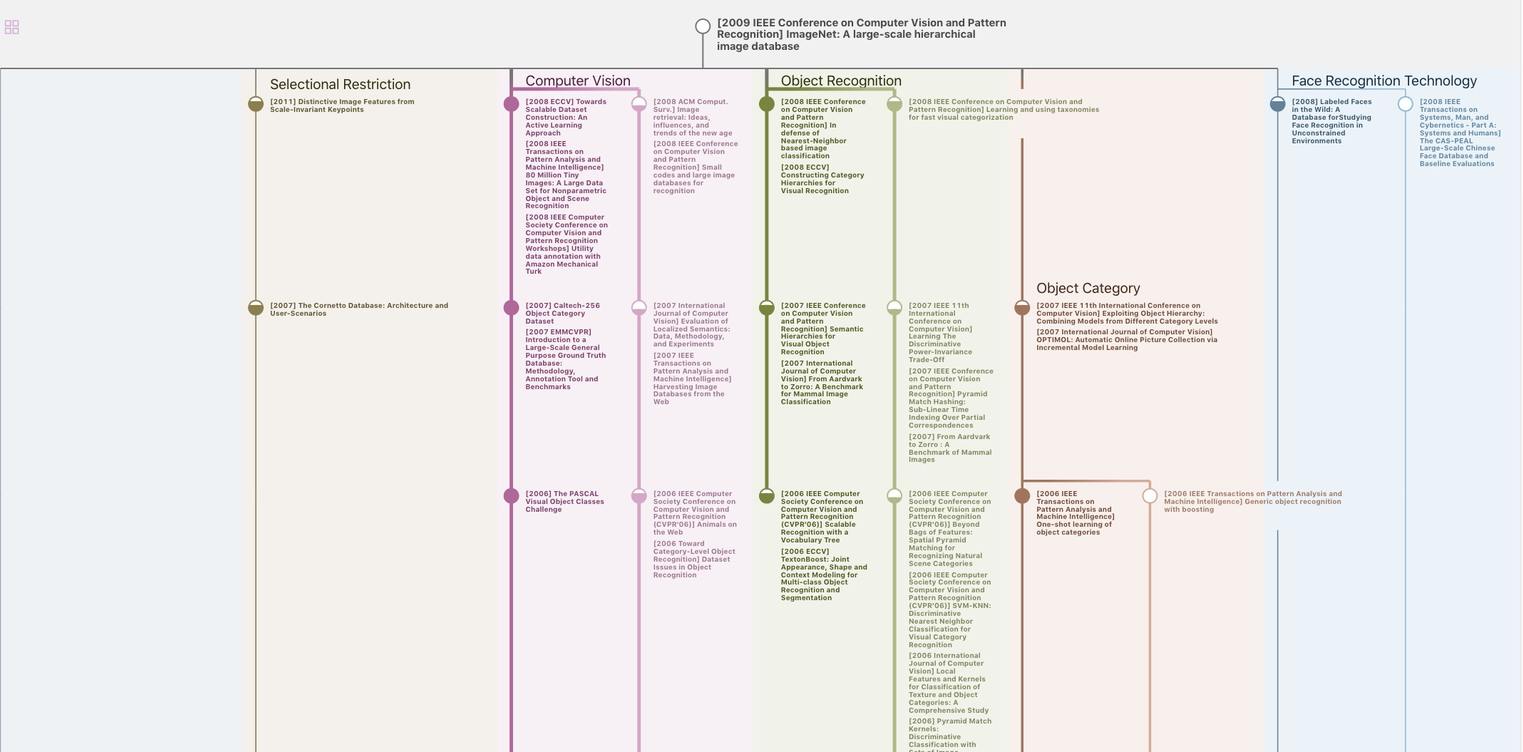
生成溯源树,研究论文发展脉络
Chat Paper
正在生成论文摘要