Learning to Locate: Adaptive Fingerprint-Based Localization With Few-Shot Relation Learning in Dynamic Indoor Environments
IEEE Transactions on Wireless Communications(2023)
摘要
WiFi fingerprint-based localization has been intensive studies as a promising technology of ubiquitous location-based services. Two main concerns for its wide spread applications are to tackle with the cumbersome efforts of site survey and to combat vulnerable environment changes. To address these issues comprehensively, we propose a novel approach on adaptive fingerprint-based localization with less site survey, named as LESS, by exploring a new paradigm of radio map construction and adaptation with few-shot relation learning. Firstly, we extend sparsely collected fingerprints with the fingerprint augmentation method which produces new related data and derives their location information based on local proximity property in a low-dimensional manifold space. Then, LESS designs deep relation networks to learn not only the appropriate features but also a transferable deep-distance metric for modeling the fundamental relationships of the neighborhood fingerprints. Finally, once trained, LESS can quickly establish the neighborhood relationships among new fingerprints in the changed surroundings to realize adaptive location estimations, even without the network updating. The extensive experimental results demonstrate that LESS can achieve an attractive trade-off between the system overhead and the location performance with the superiorities over others in dynamic indoor environments.
更多查看译文
关键词
Index Terms-Indoor localization, fingerprinting, data aug-mentation, few-shot learning
AI 理解论文
溯源树
样例
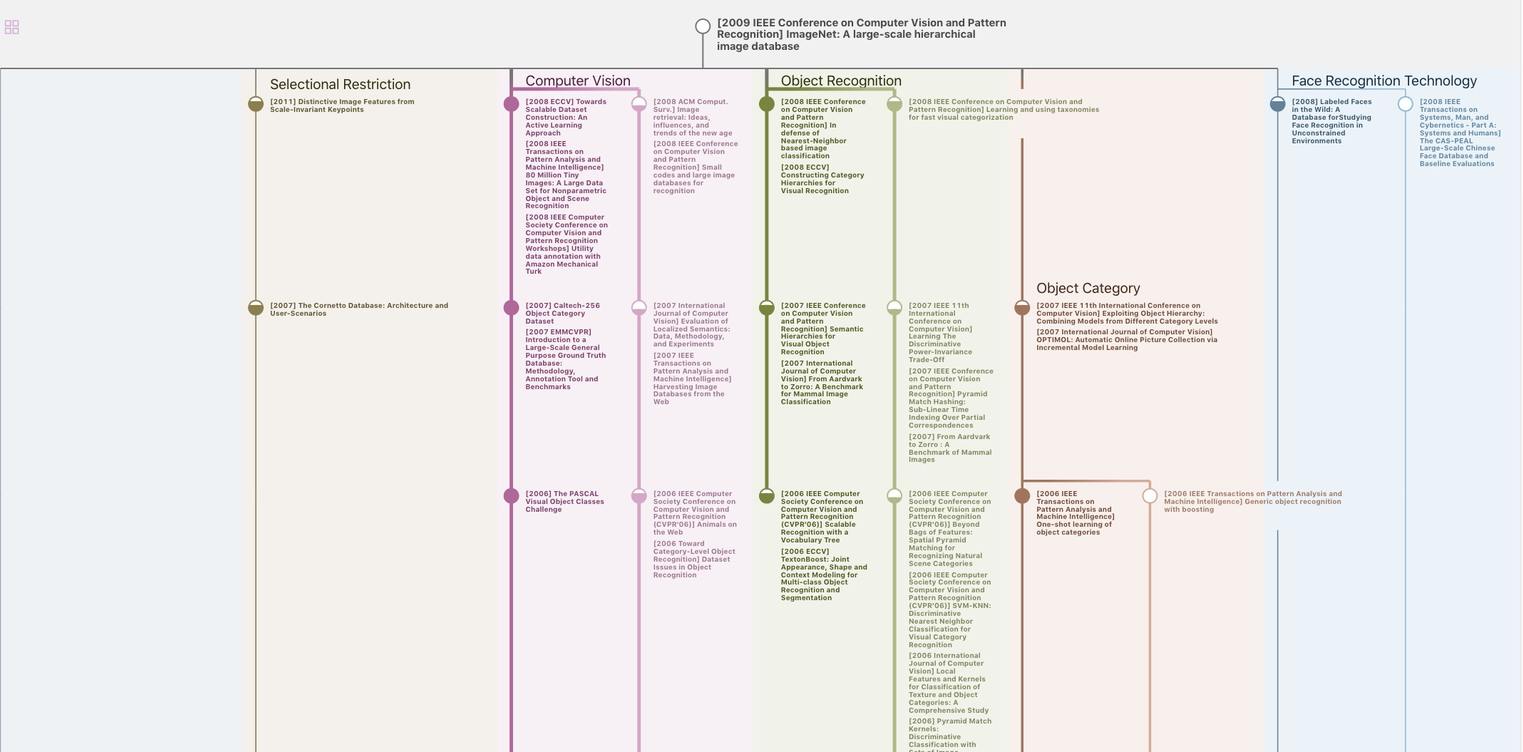
生成溯源树,研究论文发展脉络
Chat Paper
正在生成论文摘要