Community-aware graph embedding via multi-level attribute integration
KNOWLEDGE AND INFORMATION SYSTEMS(2023)
摘要
Graph embedding has been extensively studied in the literature and is widely used in various applications such as drug discovery, social network analysis, and natural language processing. However, existing approaches ignore the attribute information or are limited to learning graph representations at certain graph scales without considering the layer-wise community structure to improve embedding learning. To tackle these problems, we propose a Community-aware graph Embedding method with Multi-level attribute integration, a novel attributed graph embedding framework. It first coarsens the topological structure and attributes information alternating in a parallel strategy. Then in each coarsened layer, a self-attention mechanism is adopted to naturally integrate node attributes and obtain node embedding representations. Next, we introduce neighbor propagation at the same layer, cross-layer community propagation, and consider community information of nodes in the coarsening process to modify the embedding representation. Compared with the previous graph embedding methods, experimental results in different application scenarios on real-world datasets demonstrate the effectiveness of our proposed algorithm.
更多查看译文
关键词
Attributed graph,Graph embedding,Multi-level,Community structure
AI 理解论文
溯源树
样例
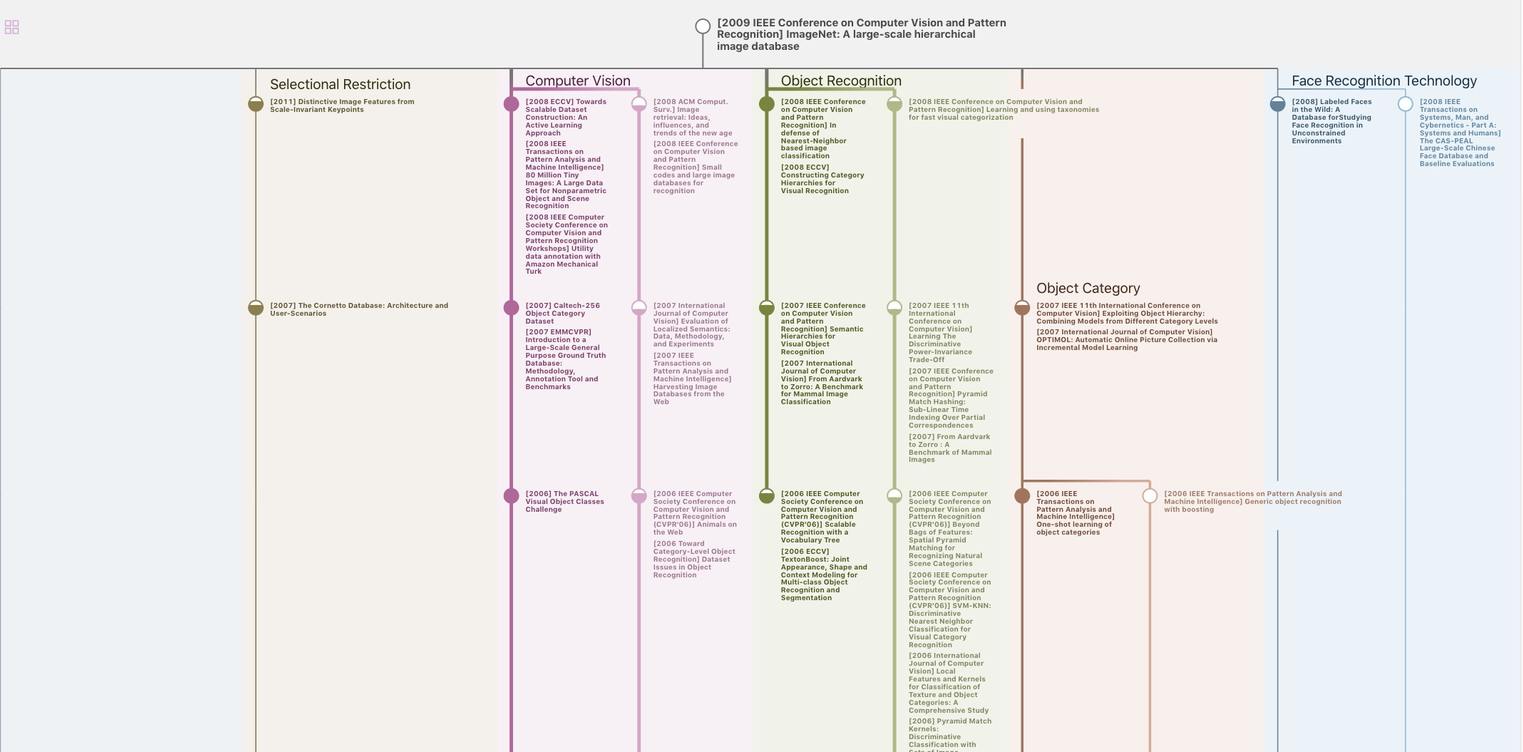
生成溯源树,研究论文发展脉络
Chat Paper
正在生成论文摘要