A novel consensus PSO-assisted trajectory unified and trust-tech methodology for DNN training and its applications
Neural Comput. Appl.(2023)
摘要
The deep neural network (DNN) relies heavily on local solvers like stochastic gradient descent (SGD). However, these methods are sensitive to initial points and hyperparameters for their local property, which affects the stability of the optimization of DNN. This paper presents a novel three-stage methodology, termed consensus particle swarm optimization-based trajectory unified optimization-assisted Trust-Tech (CPTT), for DNN training with high accuracy and robustness. The CPTT is composed of Stage I: exploration and consensus, Stage II: robustly convergence, and Stage III: search optima. We explore the effect of each stage of the CPTT methodology proposed in this paper to accomplish the following advantages: (1) high-quality local optimal solutions (LOSs) and (2) stable convergence in random initialization. The performance of CPTT has been evaluated in stages on popular classification model structures and benchmark datasets, and the performance improvement of CPTT has been evaluated in object detection models. Its optimization performance is also illustrated in a real-world application for drone-based visual inspection of electric power transmission line corridors.
更多查看译文
关键词
Deep neural network,Consensus-based PSO,Trajectory unified methodology,Trust-tech methodology,PLID
AI 理解论文
溯源树
样例
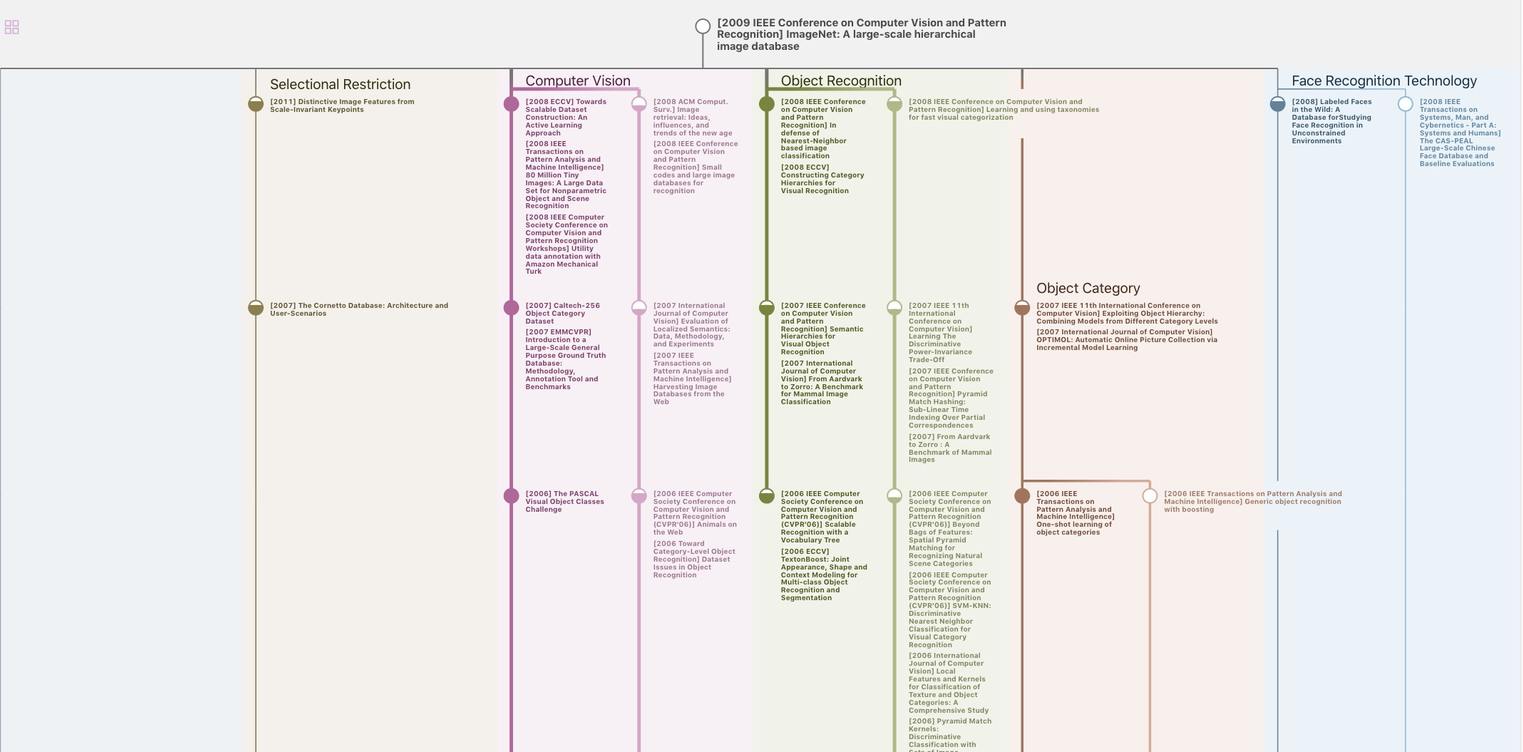
生成溯源树,研究论文发展脉络
Chat Paper
正在生成论文摘要