Integration of rotation forest and multiboost ensemble methods with forest by penalizing attributes for spatial prediction of landslide susceptible areas
STOCHASTIC ENVIRONMENTAL RESEARCH AND RISK ASSESSMENT(2023)
摘要
Landslides are among the most destructive natural hazards causing loss of life, destruction of infrastrucures and damage to properties, especially in hilly and mountaneous areas all over the world. To properly plan and manage these regions, it is crucial to accurately identify areas susceptible to landslides. In this study, we developed and applied novel hybrid machine learning models, RF-FPA and MAB-FPA, which combine forest by penalizing attribute (FPA), with two ensemble techniques, random forest (RF) and multiboost (MAB), to construct landslide susceptibility maps of Dien Bien province in Vietnam. For the development of hybrid models, we have used data of 665 landslide events and 12 landslide conditioning factors namely distance to rivers, elevations, distance to roads, normalized difference vegetation index, faults, curvature, slope, flow accumulation, stream power index, geology, topographic wetness index (TWI), and aspect. Standard statistical measures including area under the receiver operating characteristic (AUC-ROC) curve were used to evaluate predictive performance of the developed models. Results showed that both proposed novel hybrid models performed well in the correct identification of landslide susceptible areas, with RF-FPA (AUC = 0.840) slightly outperforming MAB-FPA (AUC = 0.814). The performance of the proposed hybrid models was also significantly better than that of single base classifier FPA (AUC = 0.807). In conclusion, while all the studied models performed well, the novel RF-FPA model is a more promissing tool for accurate mapping and correct prediction of landslide susceptibile areas compared to MAB-FPA amd FPA models. This approach of integrating RF with FPA can also be applied to spatially predict landslides in other areas, considering local geo-environmental conditions for proper management of landslide-prone areas.
更多查看译文
关键词
multiboost ensemble methods,rotation forest,spatial prediction,landslide
AI 理解论文
溯源树
样例
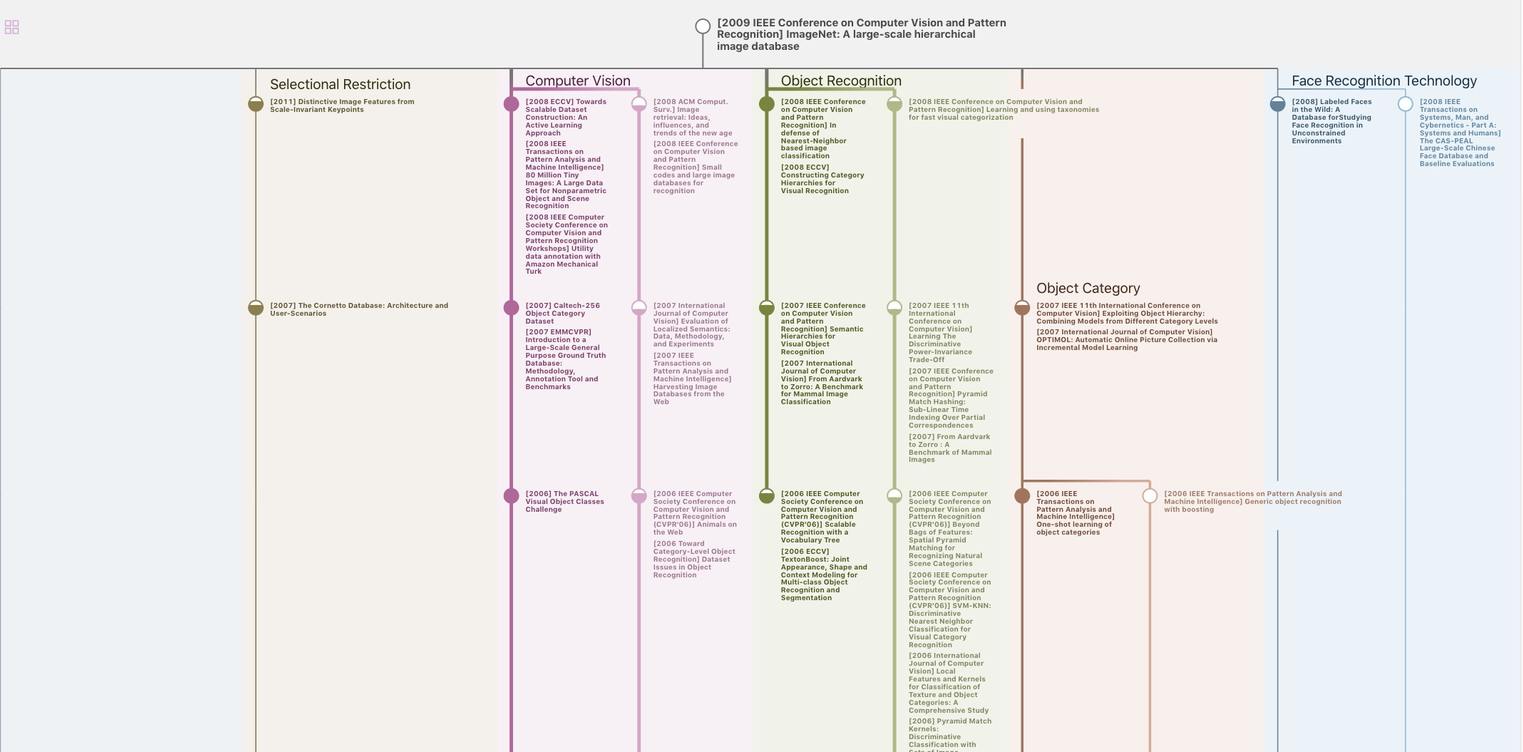
生成溯源树,研究论文发展脉络
Chat Paper
正在生成论文摘要