LEFSA: label enhancement-based feature selection with adaptive neighborhood via ant colony optimization for multilabel learning
International Journal of Machine Learning and Cybernetics(2024)
摘要
To date, multilabel learning has garnered attention increased from scholars and has a significant effect on practical applications; however, most feature selection models with classification margin cannot fully reflect the correlations between the feature and label sets. This work constructs a label enhancement-based feature selection method via ant colony optimization (ACO) on multilabel data. First, by combining the feature cosine distance and label distance of the samples, a global distance between the samples is presented, and an adjustment parameter is defined to dynamically regulate the label distance between the samples. The discriminant relation between the samples is presented to distinguish the homogeneous or heterogeneous samples of the target sample. An average classification margin-based adaptive neighborhood radius of the target sample is designed. Thus, a new adaptive fuzzy neighborhood rough set is proposed. Second, by integrating the algebraic and information viewpoints, the roughness degree is fused with the multilabel fuzzy neighborhood mutual information. The weight of each label is generated based on the label distribution of all the samples. Label enhancement-based fuzzy neighborhood mutual information can be determined to generate the final correlation of each feature and label set. Finally, Pearson correlation coefficient with an upper approximation will be applied to construct the pheromone initialization of the feature. Two metrics can be used as the heuristic information of the ACO to guide the ants to select significant features. Thus, a label enhancement-based multilabel feature subset selection methodology will be provided to obtain a superior set of features. The results from experiments confirm the capability of the proposed methodology in implementing significant classification effects on 13 datasets.
更多查看译文
关键词
Multilabel learning,Feature selection,Fuzzy neighborhood rough set,Label enhancement,Ant colony optimization
AI 理解论文
溯源树
样例
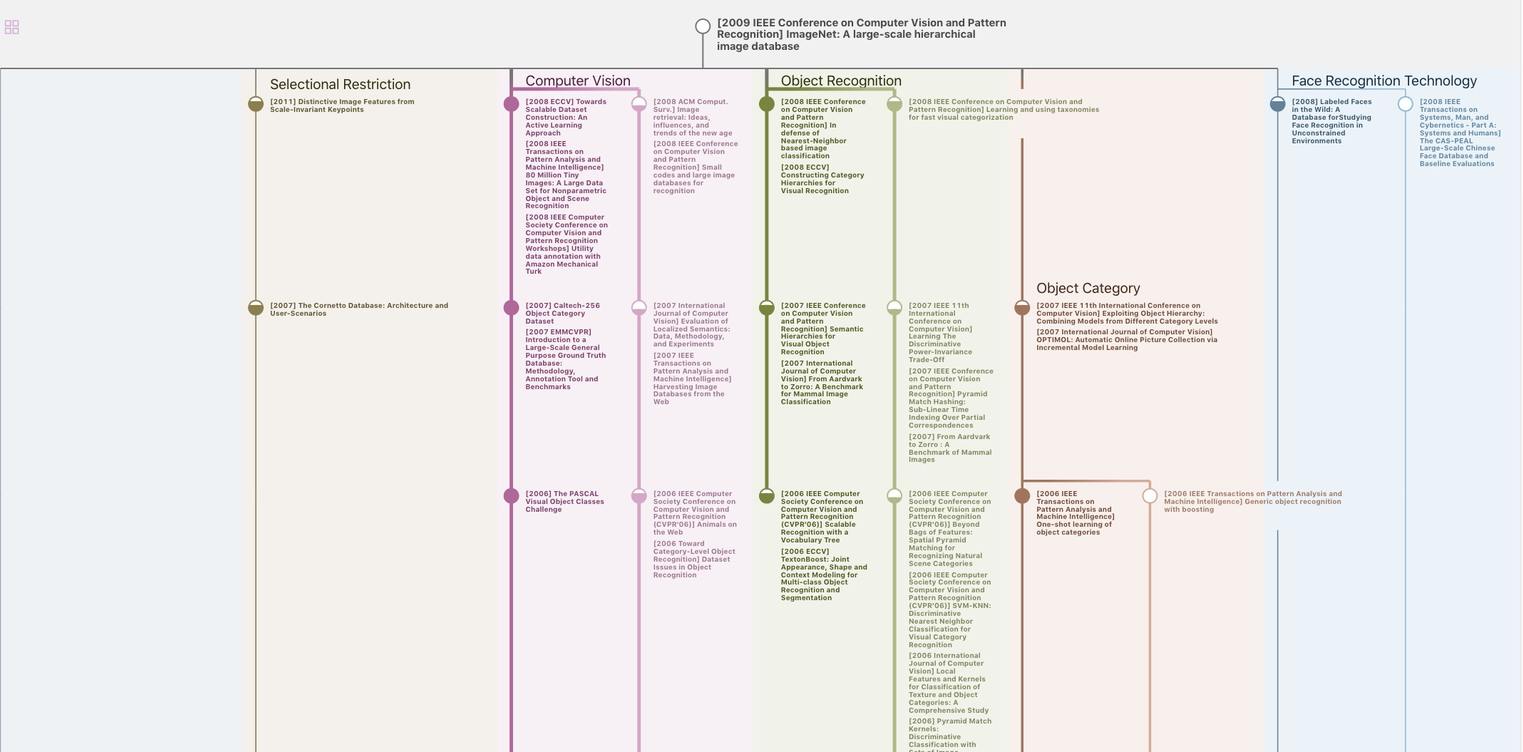
生成溯源树,研究论文发展脉络
Chat Paper
正在生成论文摘要