Shale 3D Reconstruction Method Based on Context-Aware Generative Adversarial Networks and High-Resolution Optical Flow Estimation
CHEMISTRY AND TECHNOLOGY OF FUELS AND OILS(2023)
摘要
Shale reservoirs are characterized by the presence of nano-micron pores in abundance, easy breakage due to bedding development, and difficulty in preparing true core samples. Therefore, 3D reconstruction of digital cores has become an important means of studying the microstructure features of shale. Currently, for shale matrix of dense reservoirs, existing deep learning-based reconstruction methods suffer from high costs and low accuracy, mainly due to the insufficient extraction capability of the networks for nano-micron pore features and low core resolution during reconstruction. To accurately predict the nano-micron scale pore structure of shale, we propose a shale 3D reconstruction method based on context-aware generative adversarial networks and high-resolution optical flow estimation (COFRnet-3DWGAN). This method optimizes the 3DWGAN(Wasserstein GAN) and high-resolution optical flow estimation networks by incorporating context-awareness, enhances the feature extraction capability of the networks to improve the learning degree of core nano-micron pores, and optimizes the core resolution by increasing the resolution of optical flow between core sequence images, thereby improving the accuracy of core reconstruction. The results show that, compared with WGAN, the proposed method is closer to the true core in terms of porous media morphological functions, porosity and permeability distribution, and pore structure parameters, indicating that this method has certain advantages in improving the accuracy of shale reconstruction.
更多查看译文
关键词
digital core,3D reconstruction,generative adversarial network,high-resolution optical flow estimation,context-aware
AI 理解论文
溯源树
样例
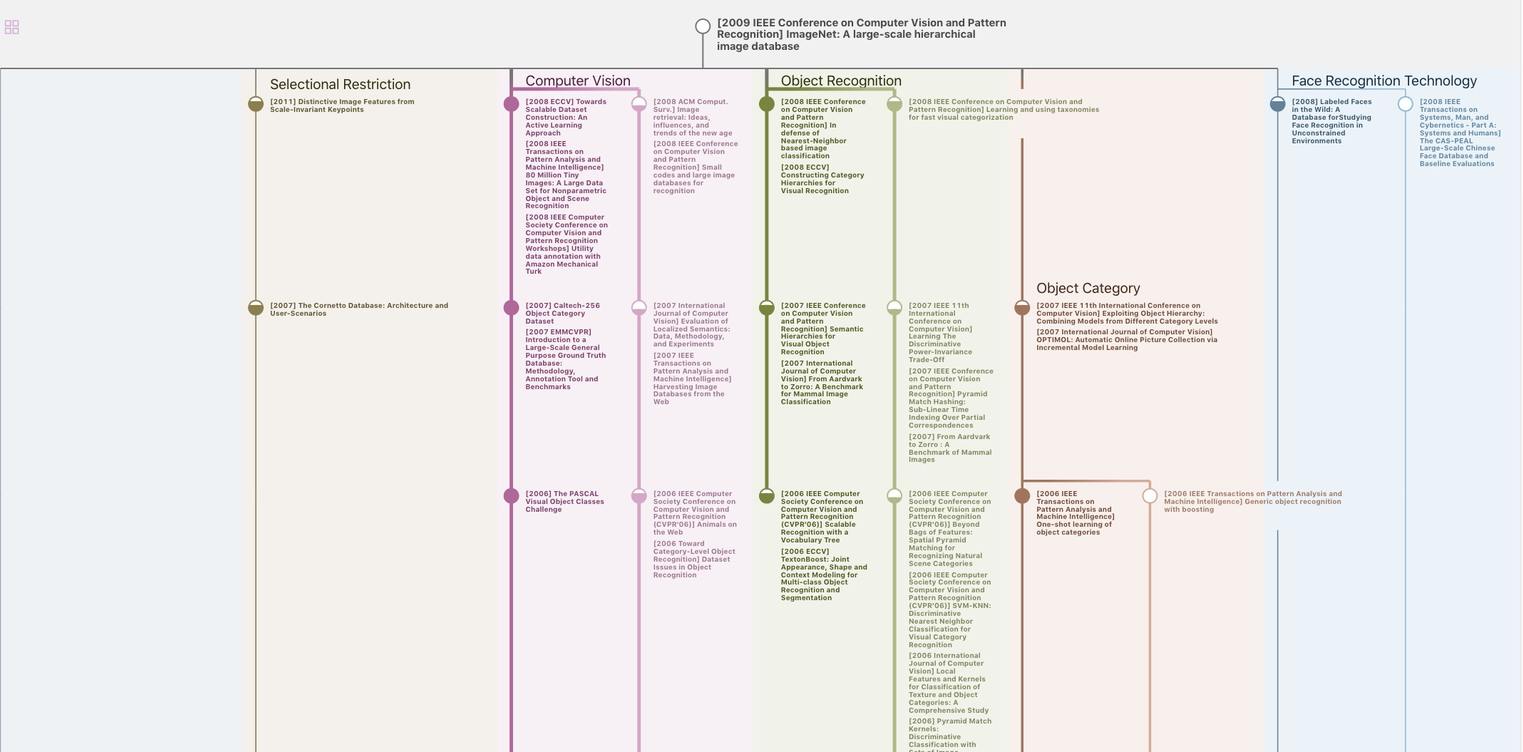
生成溯源树,研究论文发展脉络
Chat Paper
正在生成论文摘要