Time-series forecasting based on fuzzy cognitive maps and GRU-autoencoder
SOFT COMPUTING(2023)
摘要
As a powerful soft computing tool, fuzzy cognitive maps (FCMs) have been successfully employed for time-series modeling and forecasting problems. However, both the rapid time variation and the trends are still open problems when processing univariate non-stationary time-series forecasting problems via FCM-based models. In this paper, we propose a time-series forecasting model by composing FCMs, gated recurrent unit network (GRU), and autoencoder network (AE). The model is termed GAE-FCM. Firstly, a scheme based on gated recurrent unit networks and autoencoder networks is designed to learn the potential representations and capture the long-term trend of non-stationary time series while decomposing these univariate time series into a group of multivariate feature vectors. Then, the obtained multivariate feature vectors are modeled as a fuzzy cognitive map in which quantifying its connection matrix is regarded as a convex optimization problem. Finally, the time-series trend is predicted by the optimized fuzzy cognitive map and corresponding modeling mechanism. The performance of the proposed model has been validated by comparison with several representative methods on five non-stationary time-series datasets.
更多查看译文
关键词
Fuzzy cognitive maps,Gated recurrent unit,Autoencoder,Time-series forecasting
AI 理解论文
溯源树
样例
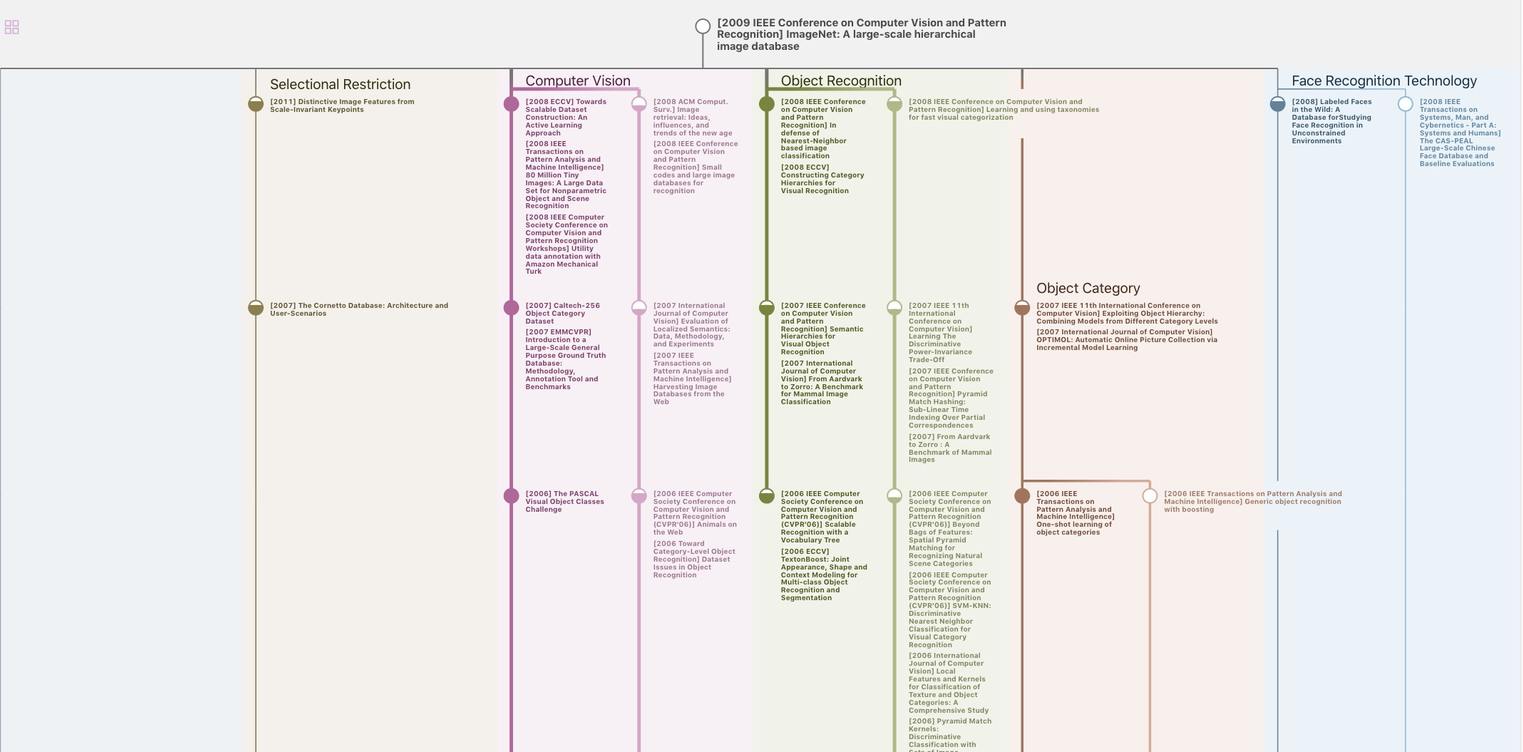
生成溯源树,研究论文发展脉络
Chat Paper
正在生成论文摘要