FAC-fed: Federated adaptation for fairness and concept drift aware stream classification
Mach. Learn.(2023)
摘要
Federated learning is an emerging collaborative learning paradigm of Machine learning involving distributed and heterogeneous clients. Enormous collections of continuously arriving heterogeneous data residing on distributed clients require federated adaptation of efficient mining algorithms to enable fair and high-quality predictions with privacy guarantees and minimal response delay. In this context, we propose a federated adaptation that mitigates discrimination embedded in the streaming data while handling concept drifts (FAC-Fed). We present a novel adaptive data augmentation method that mitigates client-side discrimination embedded in the data during optimization, resulting in an optimized and fair centralized server. Extensive experiments on a set of publicly available streaming and static datasets confirm the effectiveness of the proposed method. To the best of our knowledge, this work is the first attempt towards fairness-aware federated adaptation for stream classification, therefore, to prove the superiority of our proposed method over state-of-the-art, we compare the centralized version of our proposed method with three centralized stream classification baseline models (FABBOO, FAHT, CSMOTE). The experimental results show that our method outperforms the current methods in terms of both discrimination mitigation and predictive performance.
更多查看译文
关键词
Federated learning,Fairness,Stream classification,Deep neural network,Hedge backpropagation,Privacy
AI 理解论文
溯源树
样例
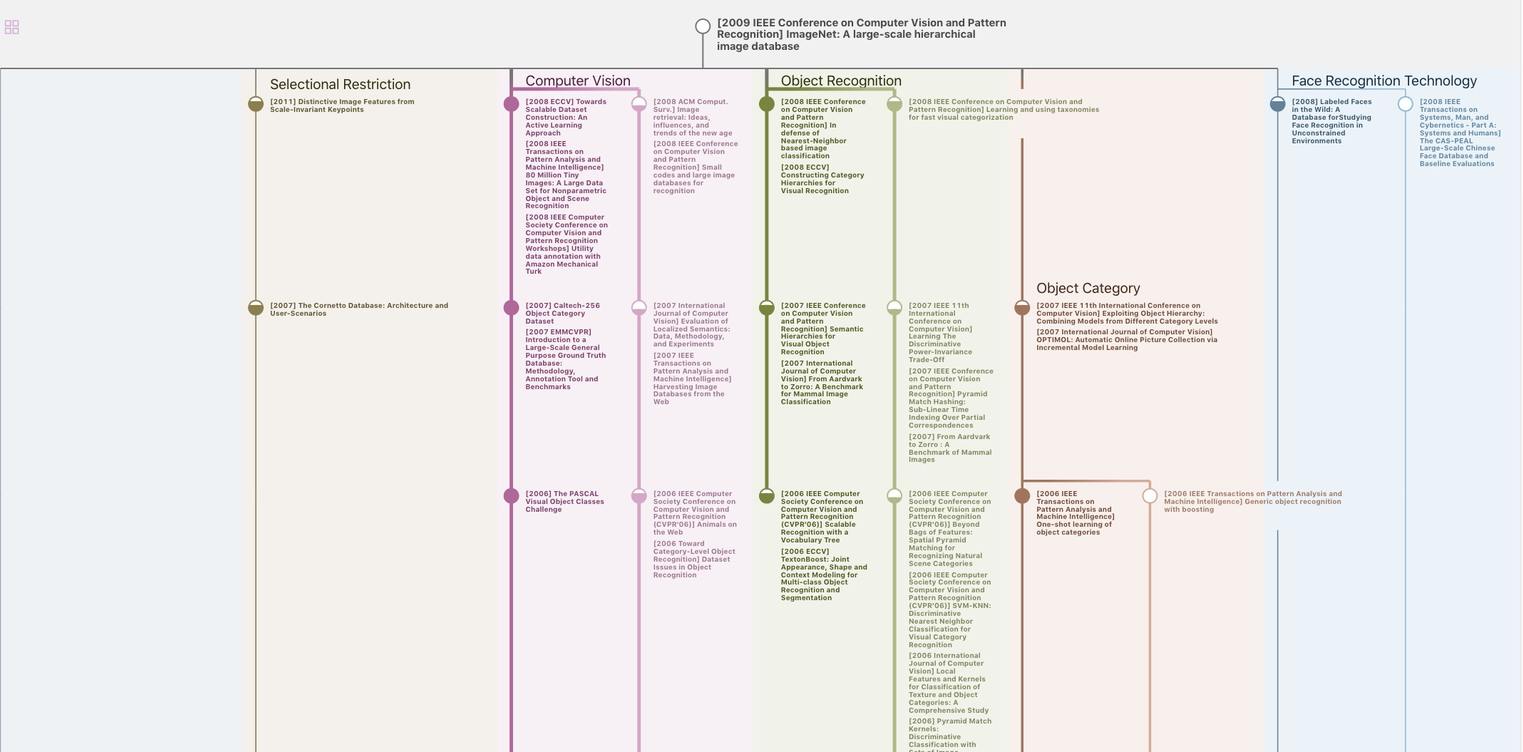
生成溯源树,研究论文发展脉络
Chat Paper
正在生成论文摘要