TGR: Neural-symbolic ontological reasoner for domain-specific knowledge graphs
APPLIED INTELLIGENCE(2023)
摘要
Ontological reasoning has great prospects in applications based on domain-specific knowledge graphs (KG). However, it is difficult for existing logic reasoners to quickly perform inference over large-scale assertional boxes (ABoxes) in domain-specific KGs with complex ontologies. To address this challenge, a novel method named the “neural-symbolic ontological reasoner” is proposed. By incorporating neural-symbolic learning into ABox reasoning, a reasoner named the TimGangReasoner (TGR) is built. The TGR synthesizes graph data using an ontology, trains an ABox reasoning network (ABRN) model, and then approximately compiles the logic reasoning process of the ontology (represented by OWL+SWRL) into neural networks (NNs). The ABRN model encodes instances into vectors and then executes parallel vector computations to accelerate ABox reasoning. Experiments conducted on three open-source complex ontologies show that the TGR can achieve high-quality approximate deductive reasoning on ABoxes. The reasoning time consumption of the TGR increases linearly with the increase in the number of assertions, providing better scalability for large-scale ABoxes. Therefore, the TGR is able to reason quickly and accurately on domain-specific KGs that have complex underlying ontologies and contain large-scale ABoxes.
更多查看译文
关键词
Domain-specific knowledge graph,Ontology,Deep learning,Neural-symbolic,Parallel reasoning,Assertional box
AI 理解论文
溯源树
样例
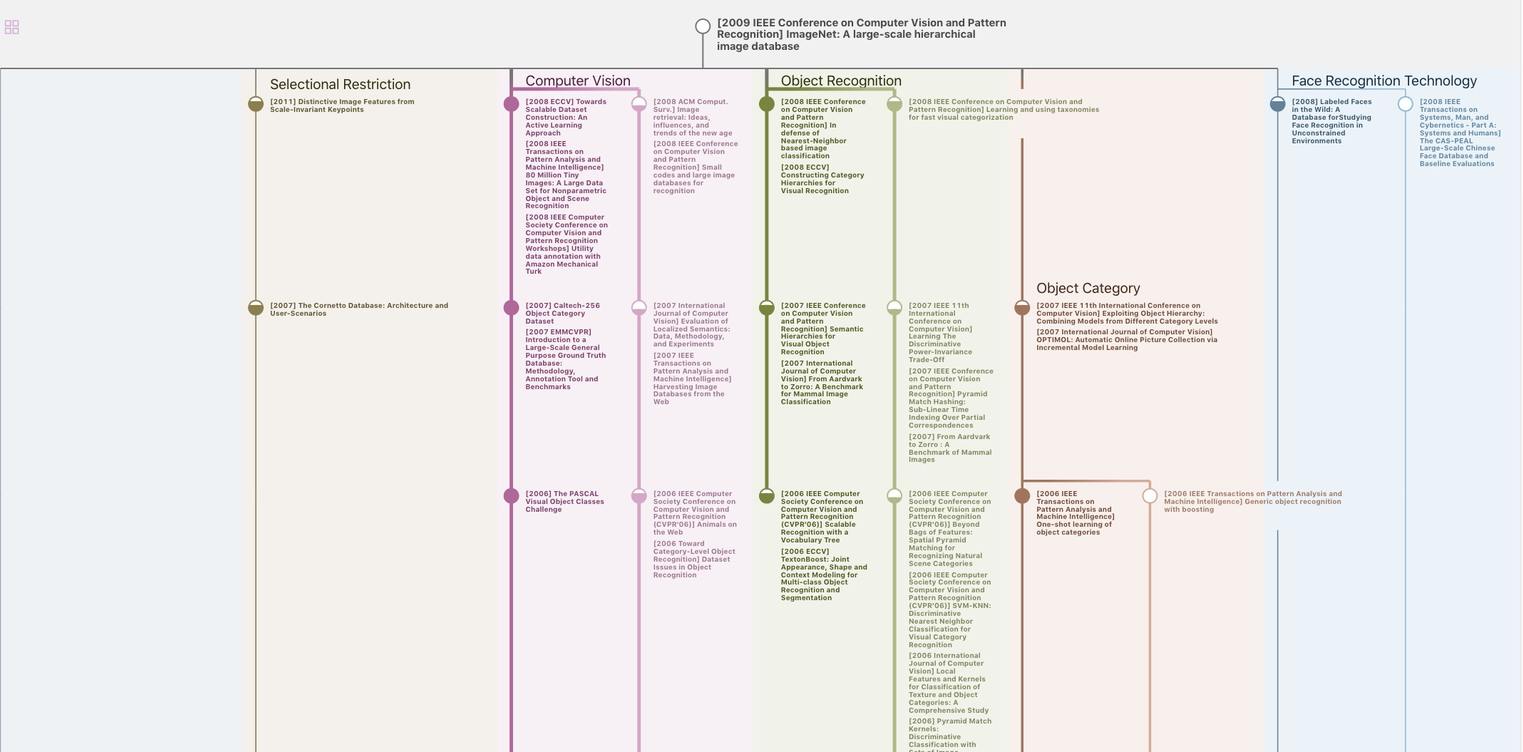
生成溯源树,研究论文发展脉络
Chat Paper
正在生成论文摘要