Study on High-Throughput Inversion Method for Anisotropic Material Parameters Based on Nanoindentation
Experimental Mechanics(2023)
摘要
Background Accurate measurements of material constitutive model parameters are of great significance for design optimization and reliability analysis. Objective In this paper, to characterize the anisotropic elastoplastic parameters of single-crystal metal materials at the nanoscale, a high-throughput inversion method of anisotropic elastoplastic constitutive parameters of single-crystal metal materials using a neural network and bicrystalline indentation load-depth curve is proposed. It addresses the limitations of indentation technology in the characterization of anisotropic material parameters. Methods A large number of finite-element simulation results were used to build a sample dataset. A neural network was used to build a mapping relationship model between the characteristics of the indentation load-depth curve and the parameters of the material elastic–plastic constitutive model. Results The parameter inversion method based on the neural network reduced the iterative optimization link, improved the parameter inversion efficiency, and realized high-throughput parameter inversion of the nonupdated intelligent material constitutive model. Conclusion The effectiveness of the method was verified by inversion experiments of anisotropic elastic–plastic parameters of a single-crystal copper material. The accuracy and universality of the method were further verified by an error analysis, demonstrating the engineering application prospects of the proposed method.
更多查看译文
关键词
Experimental mechanics,Parameter inversion,Nanoindentation,Neural networks
AI 理解论文
溯源树
样例
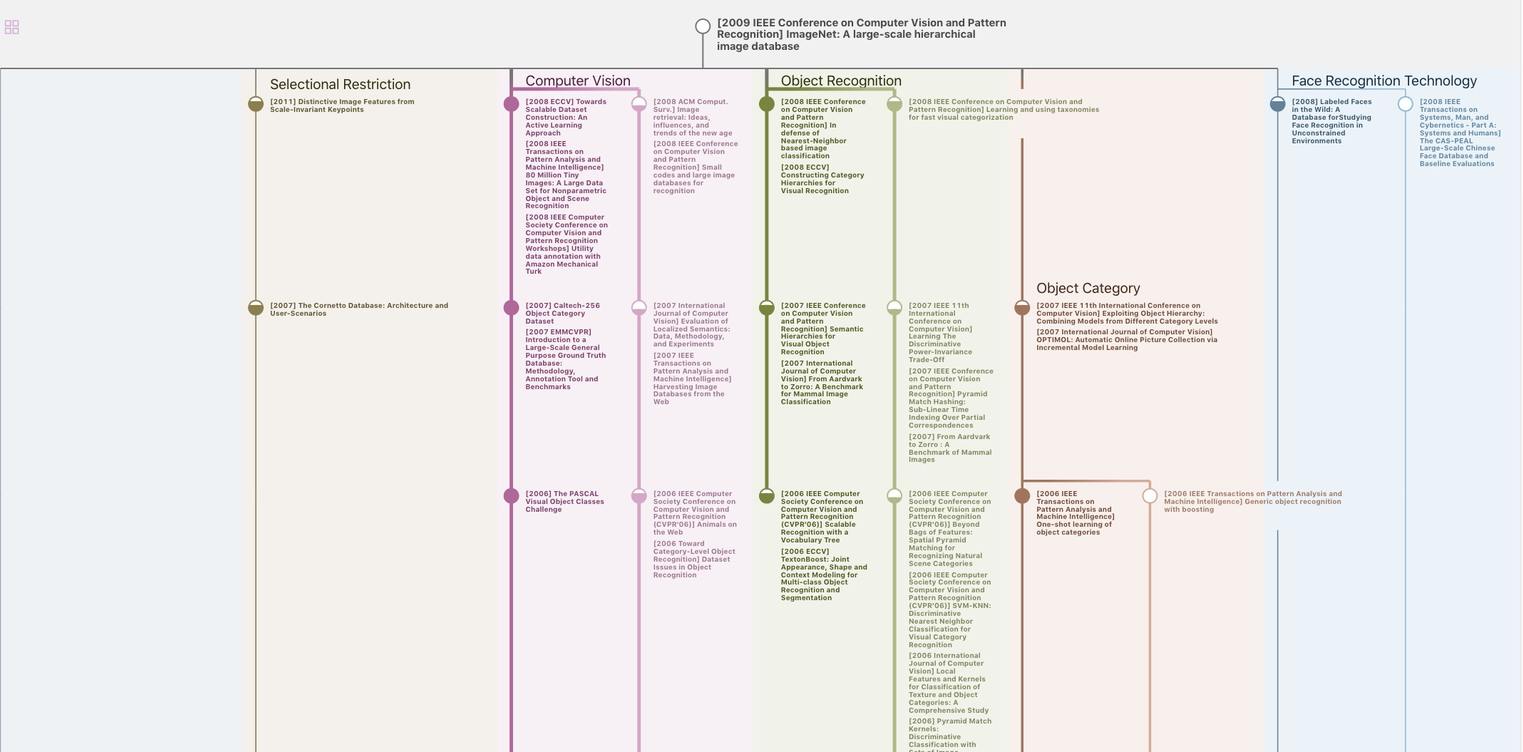
生成溯源树,研究论文发展脉络
Chat Paper
正在生成论文摘要