Spatial-temporal regularized tensor decomposition method for traffic speed data imputation
INTERNATIONAL JOURNAL OF DATA SCIENCE AND ANALYTICS(2023)
摘要
Data missing is very common in the spatial-temporal traffic data collected by various detectors, and how to accurately impute the missing values is particularly important in intelligent transportation systems. Because the method based on tensor decomposition has advantages in solving the problem of multidimensional data imputation, in this paper, we regard the missing traffic speed data imputation as a tensor decomposition problem and propose a three-process framework based on the tensor decomposition of spatial-temporal regularization, which imputes the missing traffic speed data by using the hidden spatial-temporal characteristics and underlying structure. Specifically, we first propose a high-precision initialization method based on the low-rank tensor completion model. The experimental results show that the optimal initialization of tensor decomposition has good imputation performance. Then, we design a threshold and flexibly choose the truncation rank in the truncated higher-order singular value decomposition, to get the core tensor of appropriate size and better capture the characteristics of each dimension. Finally, we apply these features and add regularization term constraints related to the time interval of one day and the location of road detectors, and the missing traffic speed data are estimated by spatial-temporal regularized Tucker decomposition (STRTD). In addition to the scenes of element-like random missing (EM) and fiber-like random missing (FM), our experiment also creates a region-like random missing (RM) by imitating the real-world loss. We have done experiments on real-world traffic speed data sets, and the results show that our STRTD model is better than the most advanced imputation model at present, even in the case of a high missing rate.
更多查看译文
关键词
Tucker decomposition imputation,Spatial–temporal traffic data,Incomplete data imputation
AI 理解论文
溯源树
样例
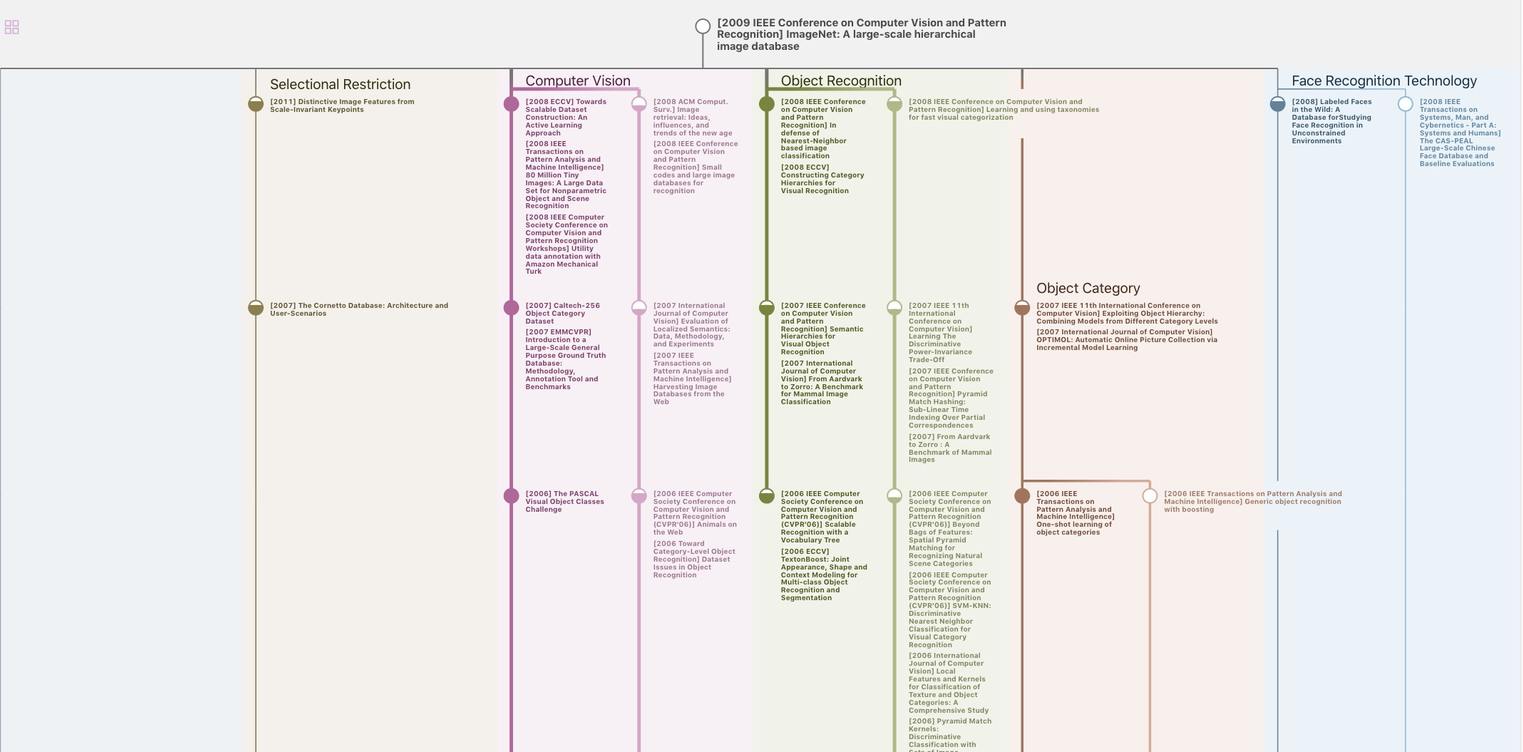
生成溯源树,研究论文发展脉络
Chat Paper
正在生成论文摘要