Teeth segmentation by optical radiographic images using VGG-16 deep learning convolution architecture with R-CNN network approach for biomedical sensing applications
OPTICAL AND QUANTUM ELECTRONICS(2023)
摘要
Today, in dentistry, computer-based techniques, such as preoperative planning and planning, and implant and surgical evaluation, are increasingly being developed. In order to achieve and implement the mentioned processes, the automatic segmentation of teeth is one of the important and primary steps. Segmentation of teeth is also used in the field of identity recognition, planning for orthodontics, and facial cosmetic surgery. Also, the separation of dental structures is very important from the anatomical and pathological point of view, which can be achieved by using segmentation. The approach of the present study to segment teeth consists of two modules, the first module is designed to identify teeth and the second module is designed to number teeth. The Faster R-CNN network is used to design the tooth recognition module, and the tooth numbering module is based on the VGG-16 convolution architecture. In this article, a database containing panoramic dental images is used to train and test the grid, and the grid is used to predict the tooth number, according to the FDI two-digit numbering system. The results of the system implementation showed that the accuracy of the proposed model in the dental diagnosis stage is 89.8% and in the tooth numbering stage is 86.5%, which indicates an improvement in the performance of the proposed system compared to previous methods. Due to the proper functioning of the system, it can be used for automated dental systems. The results of this study can help dentists to obtain more and more accurate information from radiographic images.
更多查看译文
关键词
Teeth segmentation,Panoramic radiographic images,Deep learning,Convolution neural network,VGG-16 convolution architecture,Faster R-CNN network,Biomedical sensing applications
AI 理解论文
溯源树
样例
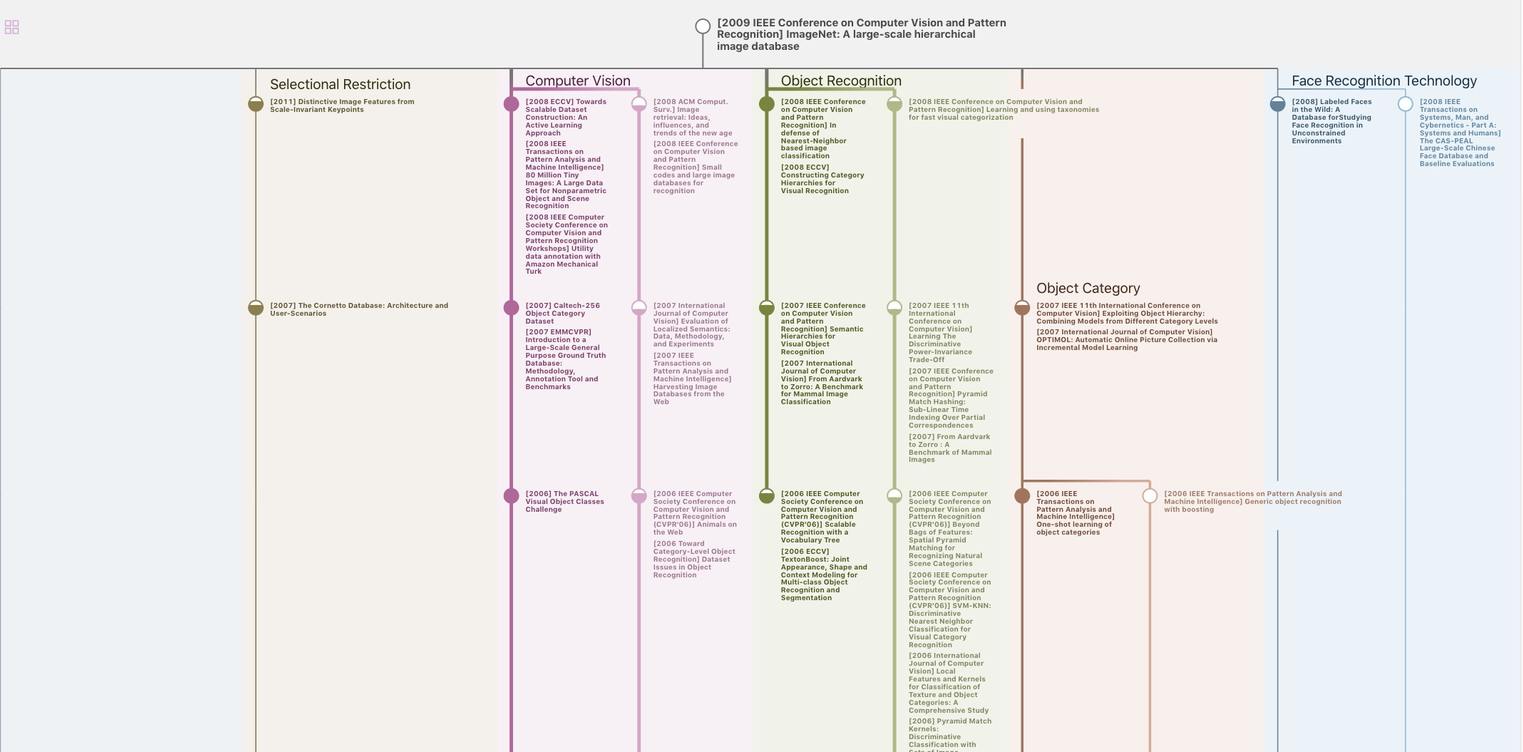
生成溯源树,研究论文发展脉络
Chat Paper
正在生成论文摘要