An optimal and modified homotopy perturbation method for strongly nonlinear differential equations
NONLINEAR DYNAMICS(2023)
摘要
Homotopy perturbation method (HPM) is one of the most popular semi-analytical methods to solve a nonlinear differential equation. However, in HPM, there is no strict rule for the choice of its linear operator, and its series solution may not always converges. In this study, firstly, we define the linear operator as an auxiliary linear operator ( ℒ_a ), in the frame of homotopy analysis method (HAM). Then we generalize this ℒ_a based on the auxiliary roots of ℒ_a=0 . Finally, using the optimization technique (on minimization of the residual error) we determine the best-fitted optimal ℒ_a for a problem. By doing this we ensure and accelerate the convergence of our semi-analytical homotopy perturbation series solution. Thereby we rename the HPM as Optimal and Modified Homotopy Perturbation Method (OMHPM). We consider three strongly nonlinear differential equations of nonlinear dynamical phenomena associated with the fluid dynamics to certify our technique. The dependencies of the form of the optimal ℒ_a and the convergence of the solution (obtained by HPM, Optimal HAM and OMHPM) on the values of parameters (involved in the scale transformation), initial/boundary conditions and artificial controlling parameters (involved in optimal HAM) are explored here. It is reported that our OMHPM is highly accurate and efficient than HPM, optimal HAM and Domain decomposition optimal HAM. Moreover, OMHPM is simple and can be applied to directly to any singular/non-singular highly nonlinear ordinary differential equations without any decomposition, special/scale transformation, linearization, artificial controlling parameters and discretization. An attempt is made to apply our optimal auxiliary linear operator onto the optimal HAM for possible fastest convergence.
更多查看译文
关键词
Optimal homotopy perturbation method,Series solution,Analytical method,Naiver–Stokes equation,Blasius equation,Optimal auxiliary linear operator
AI 理解论文
溯源树
样例
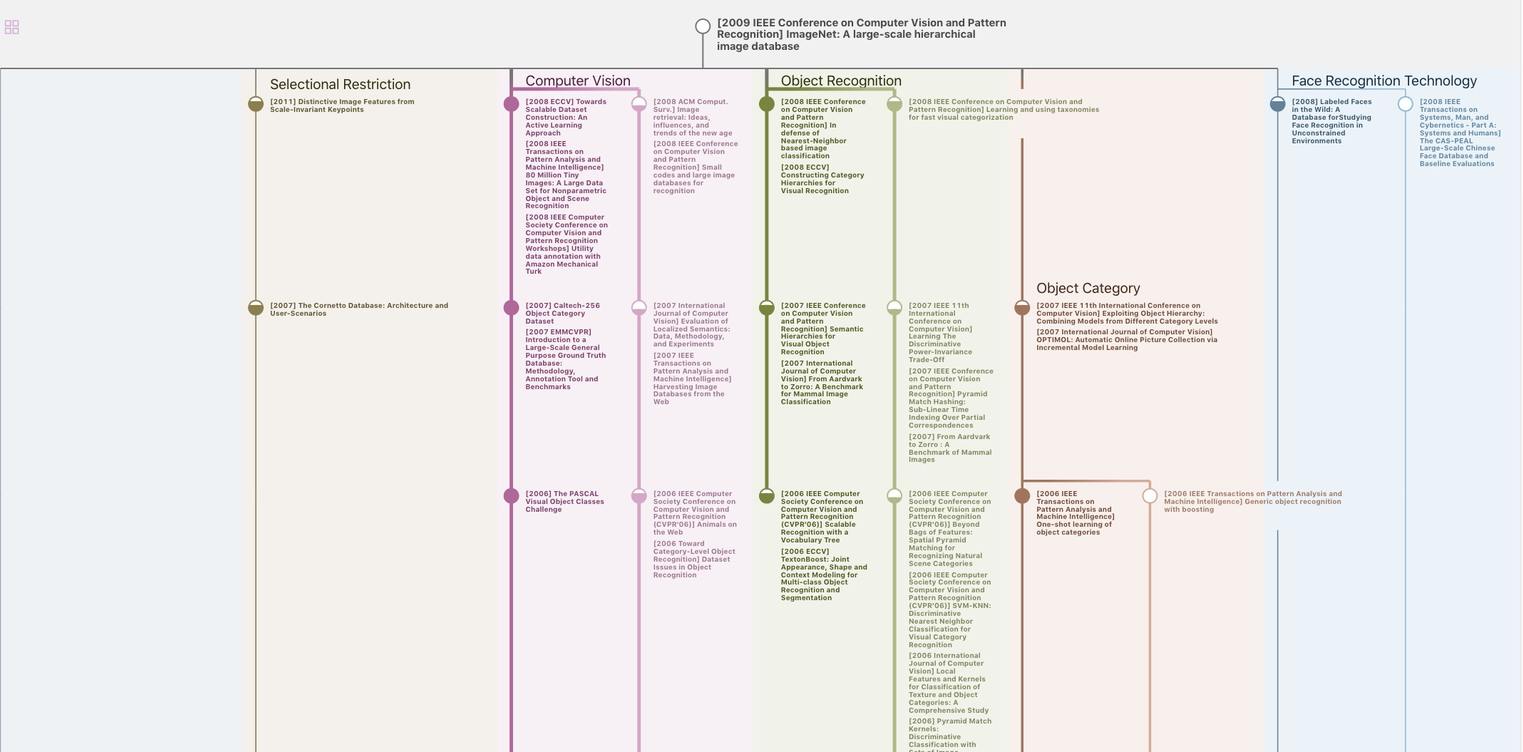
生成溯源树,研究论文发展脉络
Chat Paper
正在生成论文摘要