PARAS ^c : a parameter space-driven approach for complete association rule mining
International Journal of Data Science and Analytics(2022)
摘要
To enable efficient association rule mining, existing techniques prestore intermediate results as itemsets. However, the actual rule generation is still performed at query-time. The response time thus tends to remain unacceptably long for interactive mining, especially when rule redundancy resolution is required. Further, the widespread restriction to only support positive rules can miss important insights and lead to misleading results. For this reason, the discovery of both negative and positive rules, which can be extremely revealing, is important. Unfortunately, the generation of negative rules slows down the mining process even further. To tackle these shortcomings, we introduce the parameter space model , called PARAS^c . PARAS^c enables efficient mining of complete rules, i.e., both positive and negative rules, by precomputing and compactly maintaining the final rulesets. The PARAS^c model is based on the stable region abstractions that form the coarse granularity ruleset space for managing complete rules. Based on new insights into the redundancy relationships among complete rules, PARAS^c establishes a surprisingly compact representation of complex redundancy relationships while enabling efficient redundancy resolution for complete rules at query-time. PARAS^c supports novel classes of exploratory queries that can be answered near real time. Our experimental evaluation demonstrates that PARAS^c achieves 2–5 orders of magnitude improvement over existing techniques in rule mining.
更多查看译文
关键词
Association rule mining,Negative rule,Parameter space exploration,Rule redundancy
AI 理解论文
溯源树
样例
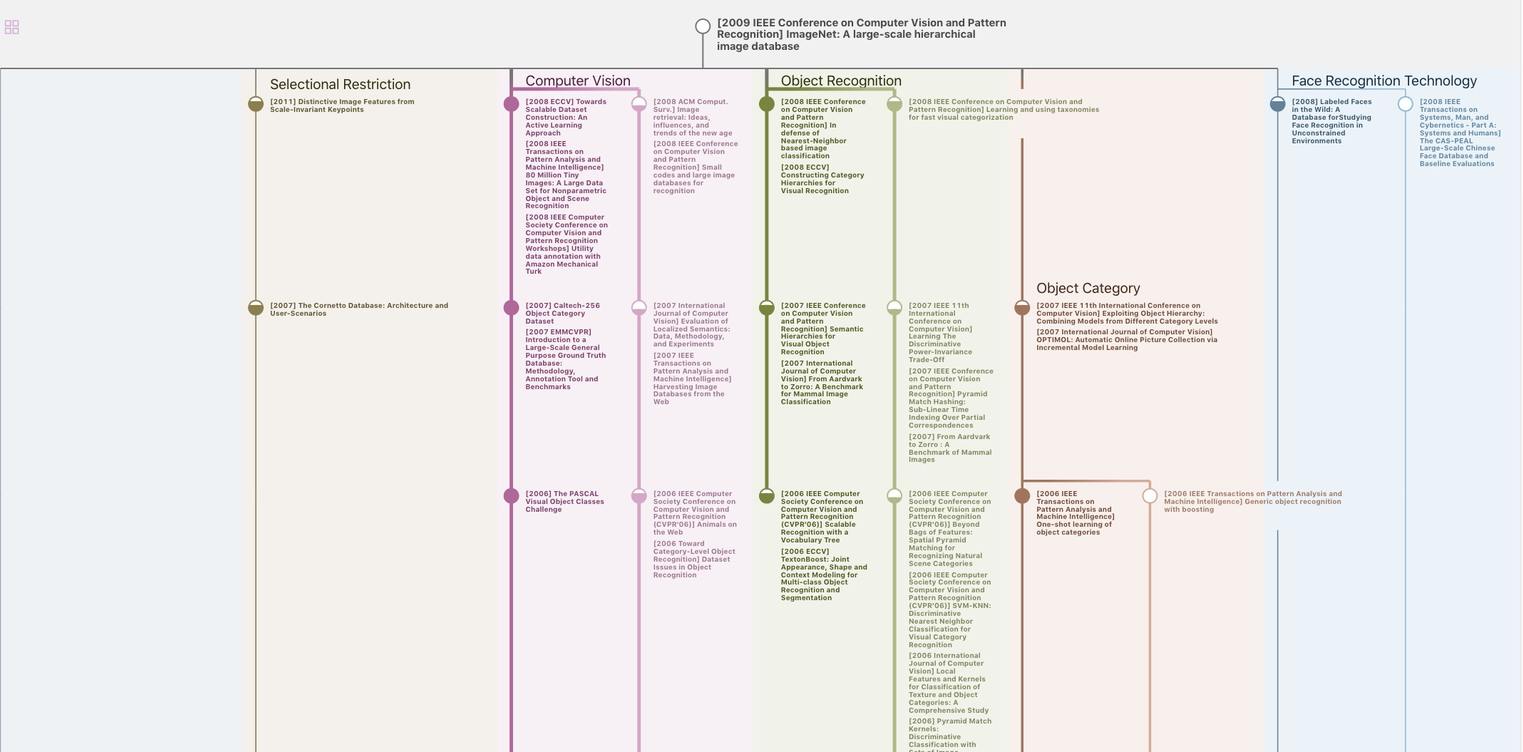
生成溯源树,研究论文发展脉络
Chat Paper
正在生成论文摘要