Data-based support for petroleum prospect evaluation
Earth Science Informatics(2020)
摘要
We consider the challenging task of evaluating the commercial viability of hydrocarbon prospects based on limited information, and in limited time. We investigate purely data-driven approaches to predicting key reservoir parameters and obtain a negative result: the information that is typically available for prospect evaluation and is suitable for data-based methods, cannot be used for the required predictions. We can show however that the same information is sufficient to produce a limited list of potentially similar well-explored reservoirs (known as analogues ) that can support the prospect evaluation work of human geoscientists. We base the proposal of analogues on similarity measures on the data available about prospects. Technically, the challenge is to define suitable similarity measures on categorical data like depositional environment or rock types. Existing data-based similarity measures for categorical data do not perform well, since they do not take geological domain knowledge into account. We propose two novel similarity measures that use domain knowledge in the form of hierarchies on categorical values. Comparative evaluation shows that the semantic-based similarity measures outperform the existing data-driven approaches and are effective in comparison to the human analogue selection.
更多查看译文
关键词
Analogues,Prediction,Similarity,Machine learning
AI 理解论文
溯源树
样例
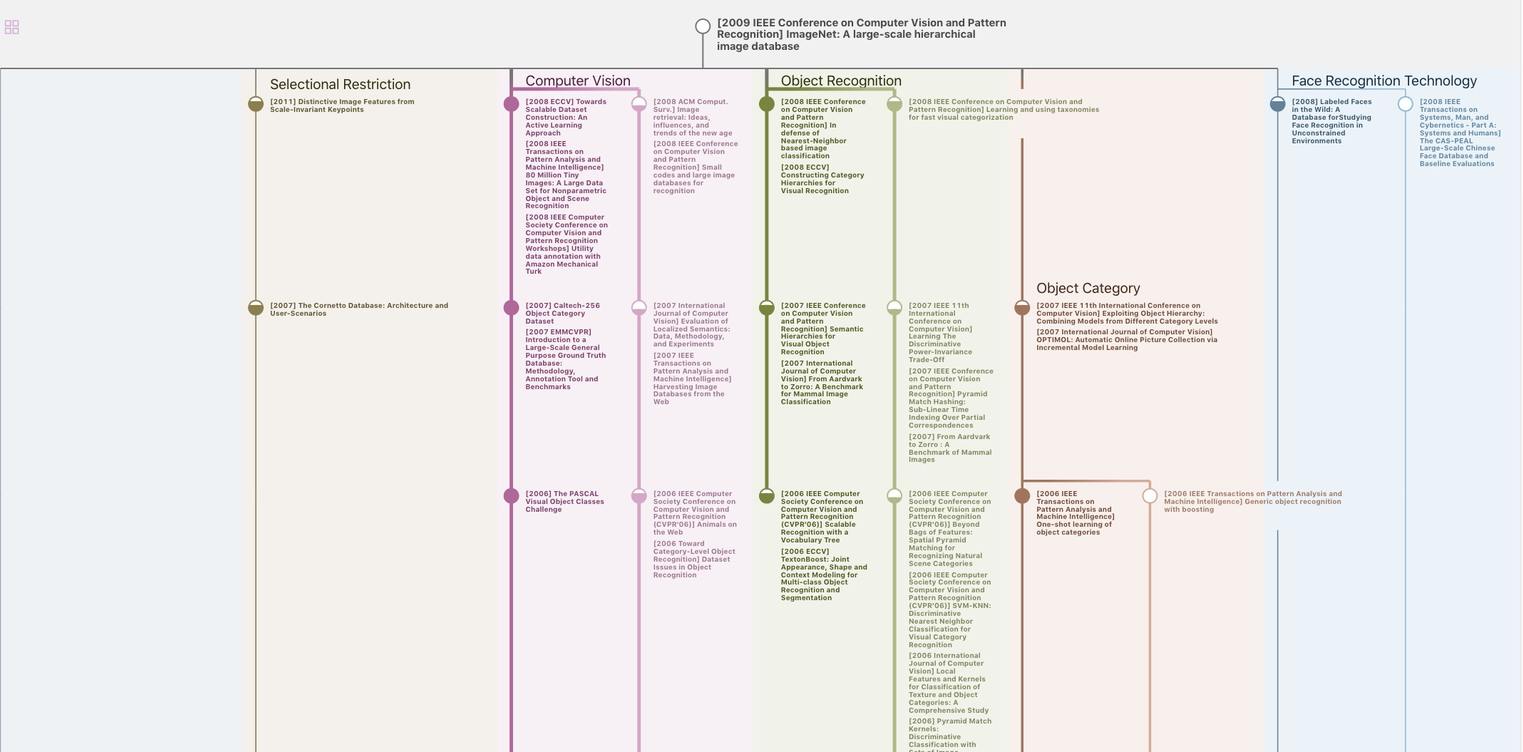
生成溯源树,研究论文发展脉络
Chat Paper
正在生成论文摘要