Collaborative on-demand dynamic deployment via deep reinforcement learning for IoV service in multi edge clouds
J. Cloud Comput.(2023)
摘要
In vehicular edge computing, the low-delay services are invoked by the vehicles from the edge clouds while the vehicles moving on the roads. Because of the insufficiency of computing capacity and storage resource for edge clouds, a single edge cloud cannot handle all the services, and thus the efficient service deployment strategy in multi edge clouds should be designed according to the service demands. Noticed that the service demands are dynamic in temporal, and the inter-relationship between services is a non-negligible factor for service deployment. In order to address the new challenges produced by these factors, a collaborative service on-demand dynamic deployment approach with deep reinforcement learning is proposed, which is named CODD-DQN. In our approach, the number of service request of each edge clouds are forecasted by a time-aware service demands prediction algorithm, and then the interacting services are discovered through the analysis of service invoking logs. On this basis, the service response time models are constructed to formulated the problem, aiming to minimize service response time with data transmission delay between services. Furthermore, a collaborative service dynamic deployment algorithm with DQN model is proposed to deploy the interacting services. Finally, the real-world dataset based experiments are conducted. The results show our approach can achieve lowest service response time than other algorithms for service deployment.
更多查看译文
关键词
deep reinforcement learning,iov service,multi edge clouds,dynamic deployment,on-demand
AI 理解论文
溯源树
样例
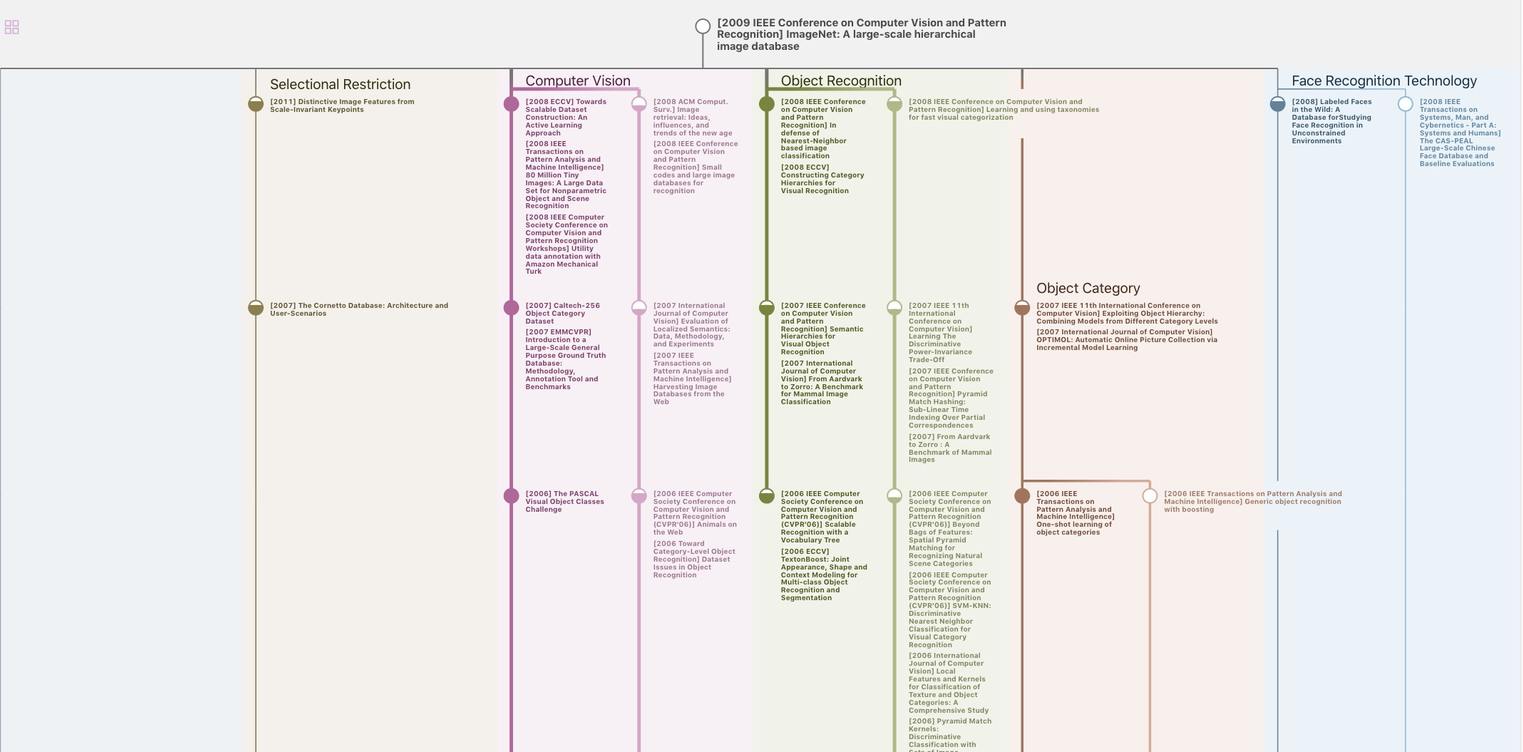
生成溯源树,研究论文发展脉络
Chat Paper
正在生成论文摘要