Designing lightweight small object detection models using attention and context
Multimedia Tools and Applications(2024)
摘要
Existing deep learning models have made some progress in improving detection accuracy for small objects, but there remains much work to be done in coordinating the practical factors involved in real object detection: accuracy, running time, model parameters and complexity. In this study, we designed multiple lightweight models and evaluated their detection performance by constructing a lightweight backbone network and embedding different combinations of attention and context modules. The results show that 1) The introduction of an attention mechanism and context information into the backbone network helps to improve the detection accuracy of the model for small objects, but the degree of improvement varies depending on the structure of the model. 2) The introduction of a context fusion network is more helpful for improving the detection performance than the introduction of an attention module. 3) The joint use of an attention mechanism and contextual information requires careful consideration of the model structure. Moreover, the designed models are lightweight. This makes the program very fast when reading and writing the parameter file. The feedforward time of the model is very short.
更多查看译文
关键词
Convolutional neural networks,Attention mechanism,Contextual information,Lightweight,Small object detection
AI 理解论文
溯源树
样例
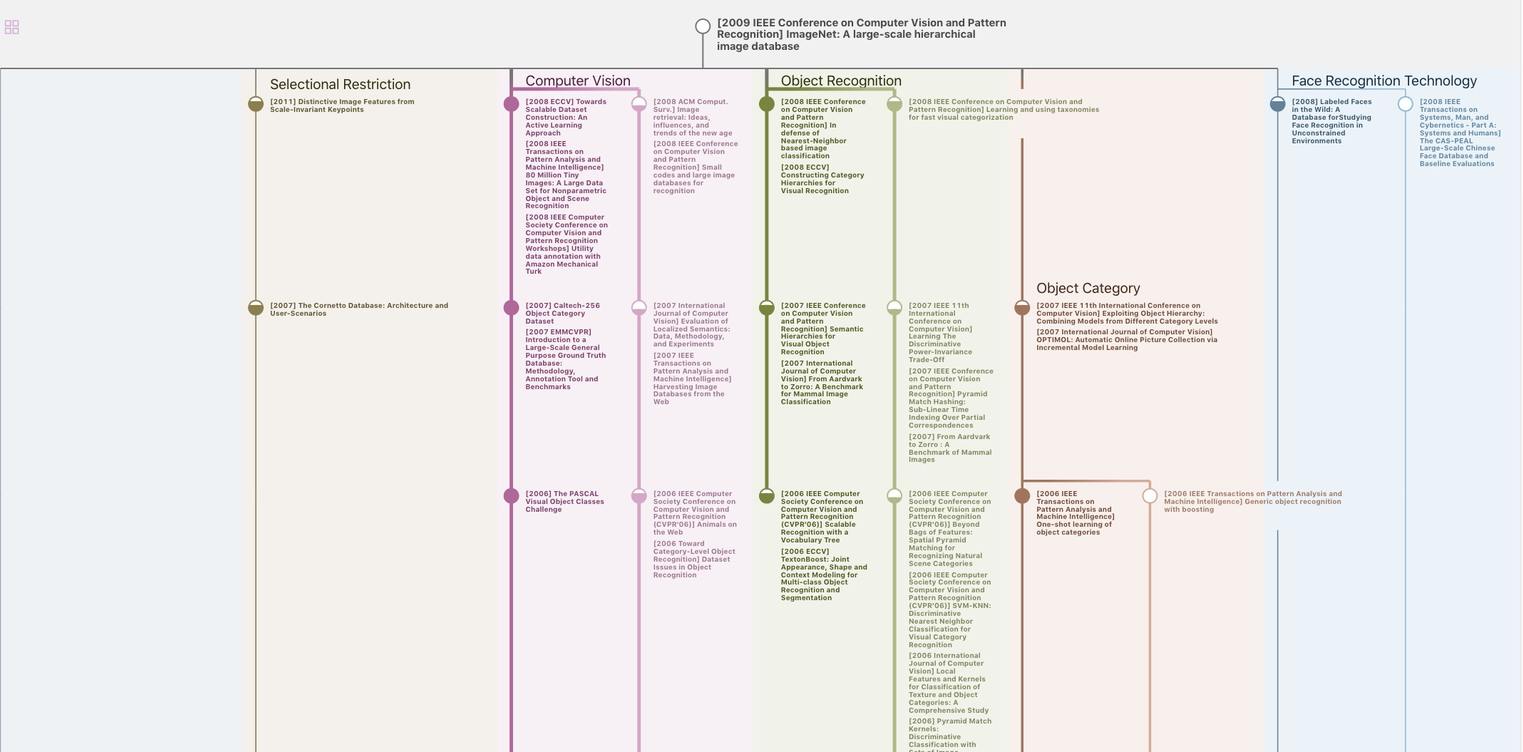
生成溯源树,研究论文发展脉络
Chat Paper
正在生成论文摘要