Optimal sample selection applied to information rich, dense data
Journal of Pharmacokinetics and Pharmacodynamics(2024)
摘要
Dense data can be classified into superdense information-poor data (type 1 dense data) and dense information-rich data (type 2 dense data). Arbitrary, random, or optimal thinning may be applied to type 1 dense data to minimise computational burden and statistical issues (such as autocorrelation). In contrast, a prospective or retrospective optimal design can be applied to type 2 dense data to maximise information gain from limited resources (capital and/or time). Here we describe a retrospective optimal selection strategy for quantification of unbound drug concentration from a discrete set of plasma samples where the total drug concentration has been measured.
更多查看译文
关键词
Dense data,Optimal design,Sample selection
AI 理解论文
溯源树
样例
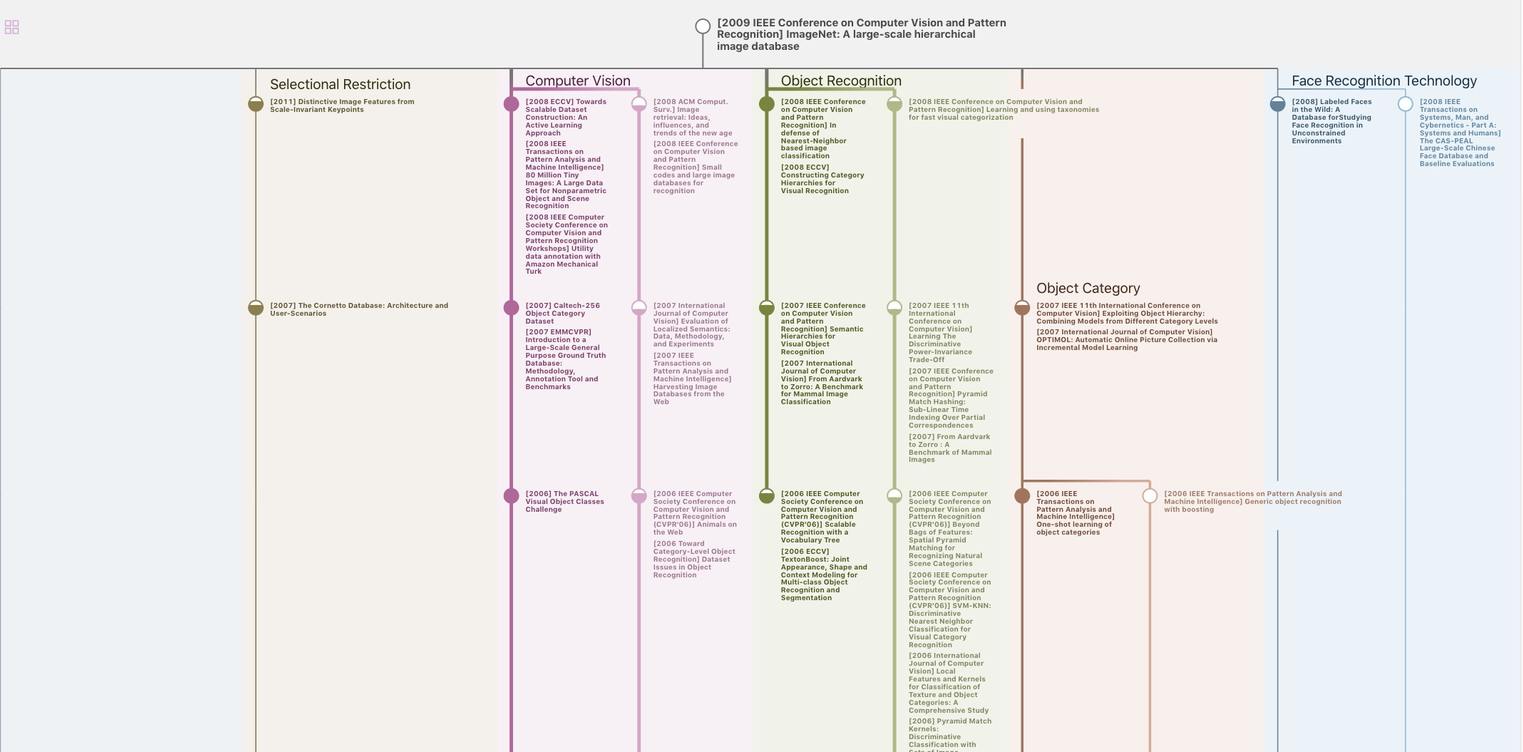
生成溯源树,研究论文发展脉络
Chat Paper
正在生成论文摘要