Conditional Independence Testing for Discrete Distributions: Beyond $\chi^2$- and $G$-tests
arXiv (Cornell University)(2023)
摘要
This paper is concerned with the problem of conditional independence testing for discrete data. In recent years, researchers have shed new light on this fundamental problem, emphasizing finite-sample optimality. The non-asymptotic viewpoint adapted in these works has led to novel conditional independence tests that enjoy certain optimality under various regimes. Despite their attractive theoretical properties, the considered tests are not necessarily practical, relying on a Poissonization trick and unspecified constants in their critical values. In this work, we attempt to bridge the gap between theory and practice by reproving optimality without Poissonization and calibrating tests using Monte Carlo permutations. Along the way, we also prove that classical asymptotic $\chi^2$- and $G$-tests are notably sub-optimal in a high-dimensional regime, which justifies the demand for new tools. Our theoretical results are complemented by experiments on both simulated and real-world datasets. Accompanying this paper is an R package UCI that implements the proposed tests.
更多查看译文
关键词
conditional independence testing,discrete distributions
AI 理解论文
溯源树
样例
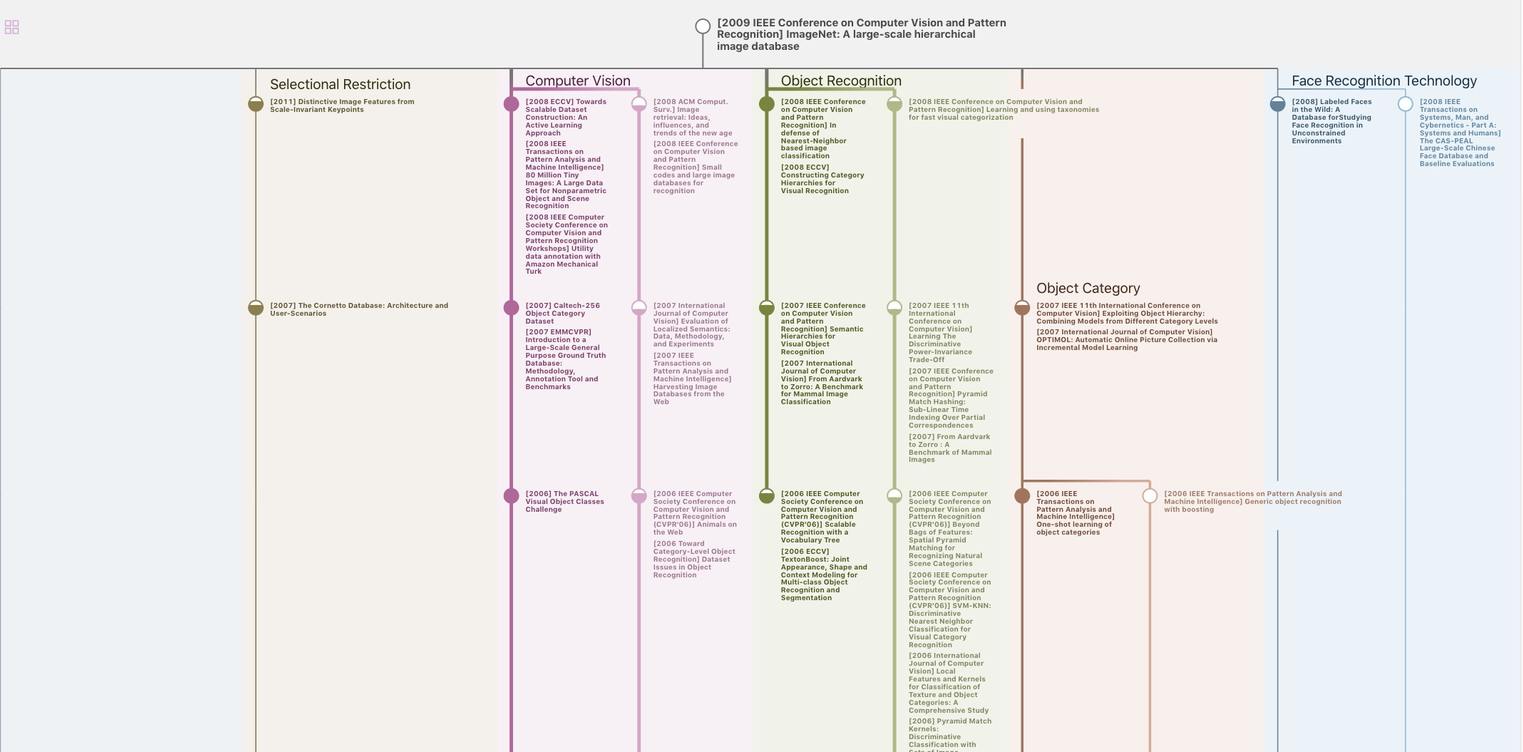
生成溯源树,研究论文发展脉络
Chat Paper
正在生成论文摘要