Deep Semantic Graph Matching for Large-scale Outdoor Point Clouds Registration
CoRR(2023)
摘要
The current point cloud registration methods are mainly based on geometric information and usually ignore the semantic information in the point clouds. In this paper, we treat the point cloud registration problem as semantic instance matching and registration task, and propose a deep semantic graph matching method for large-scale outdoor point cloud registration. Firstly, the semantic category labels of 3D point clouds are obtained by utilizing large-scale point cloud semantic segmentation network. The adjacent points with the same category labels are then clustered together by using Euclidean clustering algorithm to obtain the semantic instances. Secondly, the semantic adjacency graph is constructed based on the spatial adjacency relation of semantic instances. Three kinds of high-dimensional features including geometric shape features, semantic categorical features and spatial distribution features are learned through graph convolutional network, and enhanced based on attention mechanism. Thirdly, the semantic instance matching problem is modeled as an optimal transport problem, and solved through an optimal matching layer. Finally, according to the matched semantic instances, the geometric transformation matrix between two point clouds is first obtained by SVD algorithm and then refined by ICP algorithm. The experiments are cconducted on the KITTI Odometry dataset, and the average relative translation error and average relative rotation error of the proposed method are 6.6cm and 0.229{\deg} respectively.
更多查看译文
关键词
Large-scale point clouds,semantic adjacency graph,point clouds registration,optimal transport
AI 理解论文
溯源树
样例
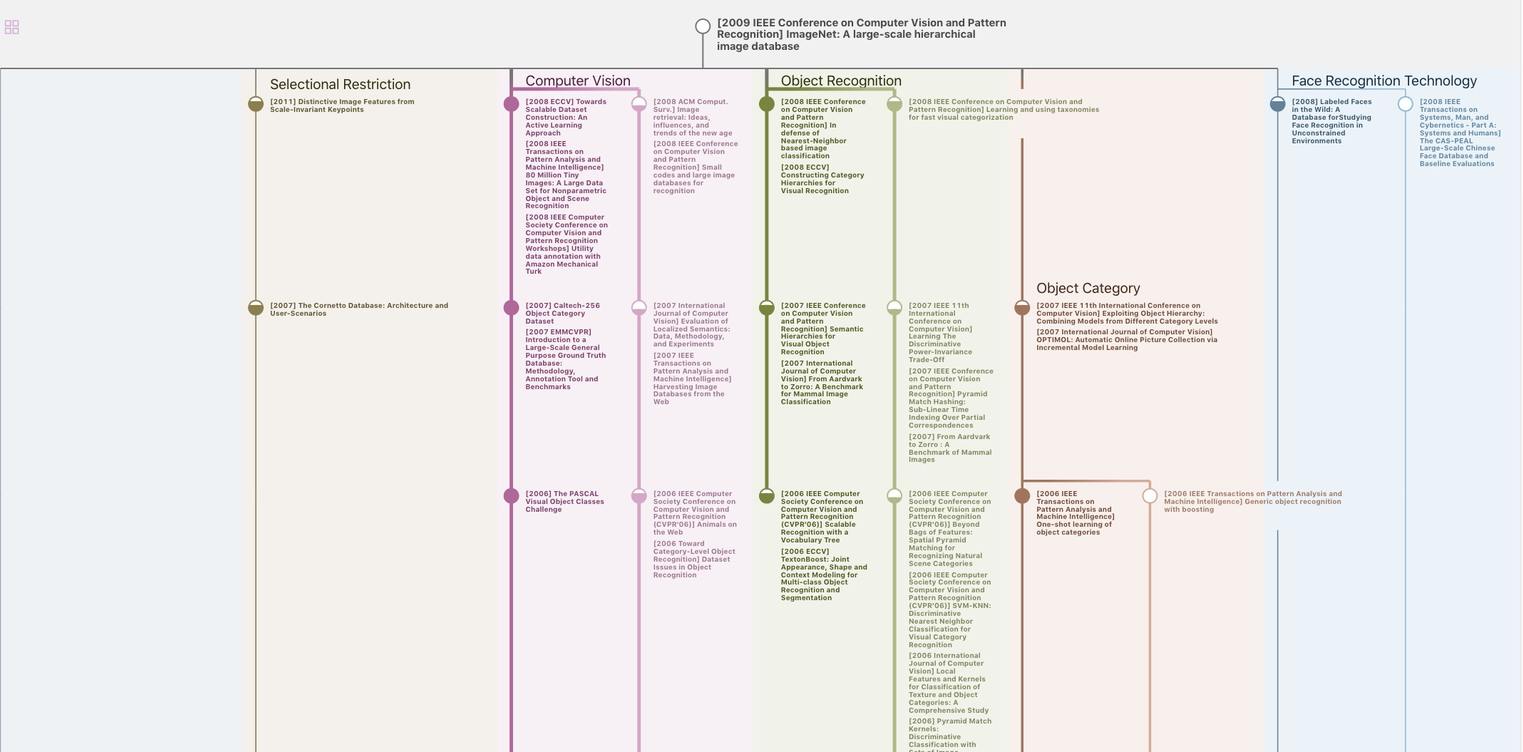
生成溯源树,研究论文发展脉络
Chat Paper
正在生成论文摘要