Efficient graph-based spectral techniques for data with few labeled samples
INTERNATIONAL JOURNAL OF DATA SCIENCE AND ANALYTICS(2023)
摘要
One of the key limitations of many existing machine learning approaches is their reliance on large labeled sets. In fact, labeled data are scarce for many applications as it is often expensive and time-consuming to obtain, and sometimes requires expert knowledge. Thus, the development of algorithms that perform well with reduced amounts of labeled data is critical. In this paper, we integrate a semi-supervised framework, a similarity graph-based setting, spectral techniques, optimization-based procedures, and specially designed forcing terms to derive two computationally tractable graph-based algorithms, which are able to obtain accurate predictions at low label rates and small amounts of labeled samples. In particular, we use a general framework involving a semi-implicit alternating scheme for the proposed techniques, and incorporate Poisson and region forcing terms. The proposed methods are very efficient, in part due to the use of spectral procedures and low-dimensional subspaces spanned by only a small number of eigenfunctions. The procedures can also be easily tailored for very large data sets by the use of certain efficient numerical solvers and can incorporate class size information, which often improves accuracy. We demonstrate the performance of our proposed methods on a variety of benchmark data sets and compare them favorably to recent existing algorithms.
更多查看译文
关键词
Data classification,Graph-based setting,Semi-supervised techniques,Spectral techniques,Low label rates,Optimization
AI 理解论文
溯源树
样例
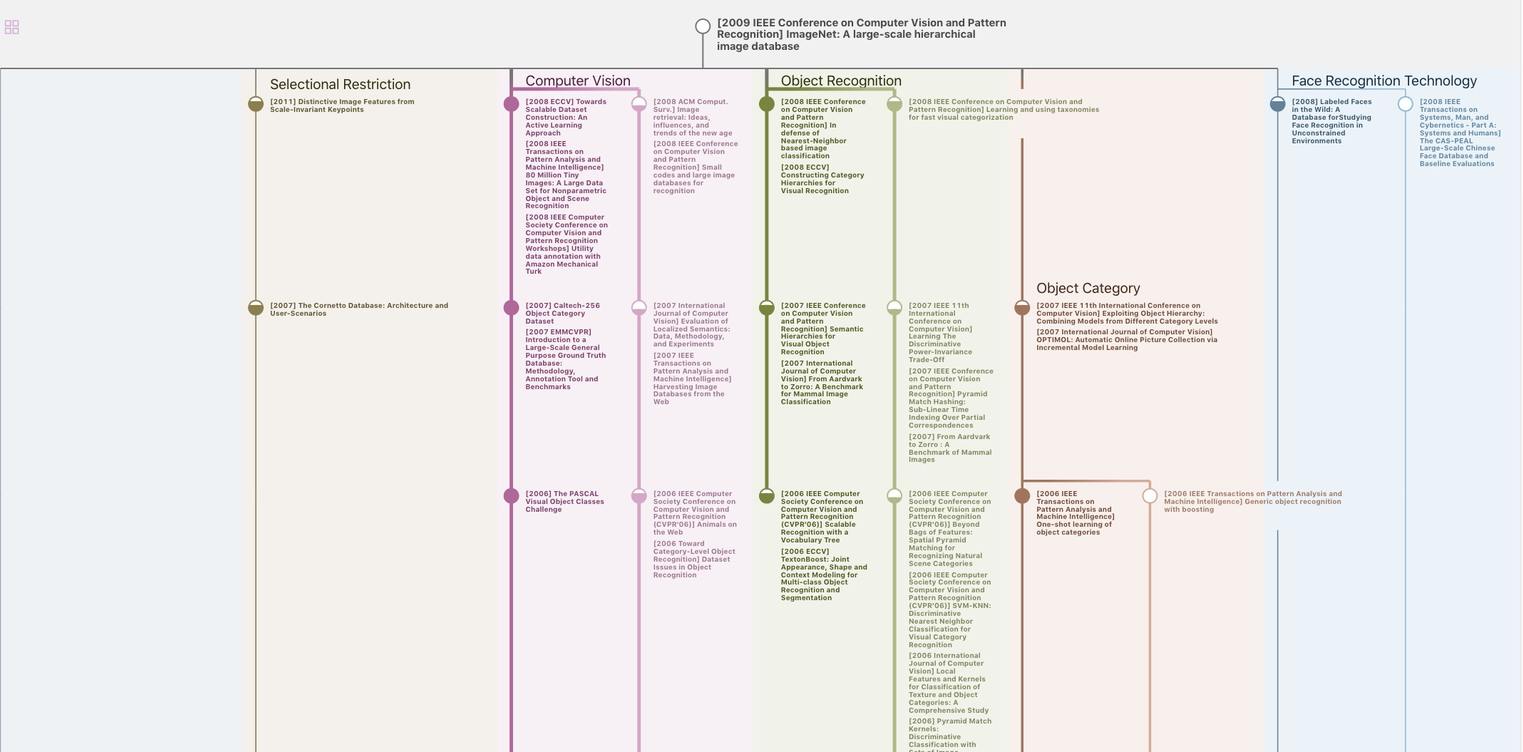
生成溯源树,研究论文发展脉络
Chat Paper
正在生成论文摘要