Hybrid Enhancement-based prototypical networks for few-shot relation classification
WORLD WIDE WEB-INTERNET AND WEB INFORMATION SYSTEMS(2023)
摘要
Few-shot relation classification is to recognize the semantic relation between an entity pair with very few samples. Prototypical network has proven to be a simple yet effective few-shot learning method for relation extraction. However, under the condition of data scarcity, the relation prototypes we achieve are usually biased compared to the real ones computed from all samples within a relation class. To alleviate this issue, we propose hybrid enhancement-based prototypical networks. In particular, our model contains three main enhancement modules: 1) a query-guided prototype enhancement module using rich interactive information between the support instances and the query instance as guidance to obtain more accurate prototype representations; 2) a query enhancement module to diminish the distribution gap between the query set and the support set; 3) a support enhancement module adopting a pseudo-label strategy to expand the scale of available data. On basis of these modules, we further design a novel prototype attention fusion mechanism to fuse information and compute discriminative relation prototypes for classification. In this way, we hope to obtain unbiased representations closer to our expected prototypes by improving the available data scale and data utilization efficiency. Extensive experimental results on the widely-used FewRel dataset demonstrate the superiority of our proposed model.
更多查看译文
关键词
Few-shot learning, Relation classification, Prototypical networks, Enhancement-based, Unbiased relation prototypes
AI 理解论文
溯源树
样例
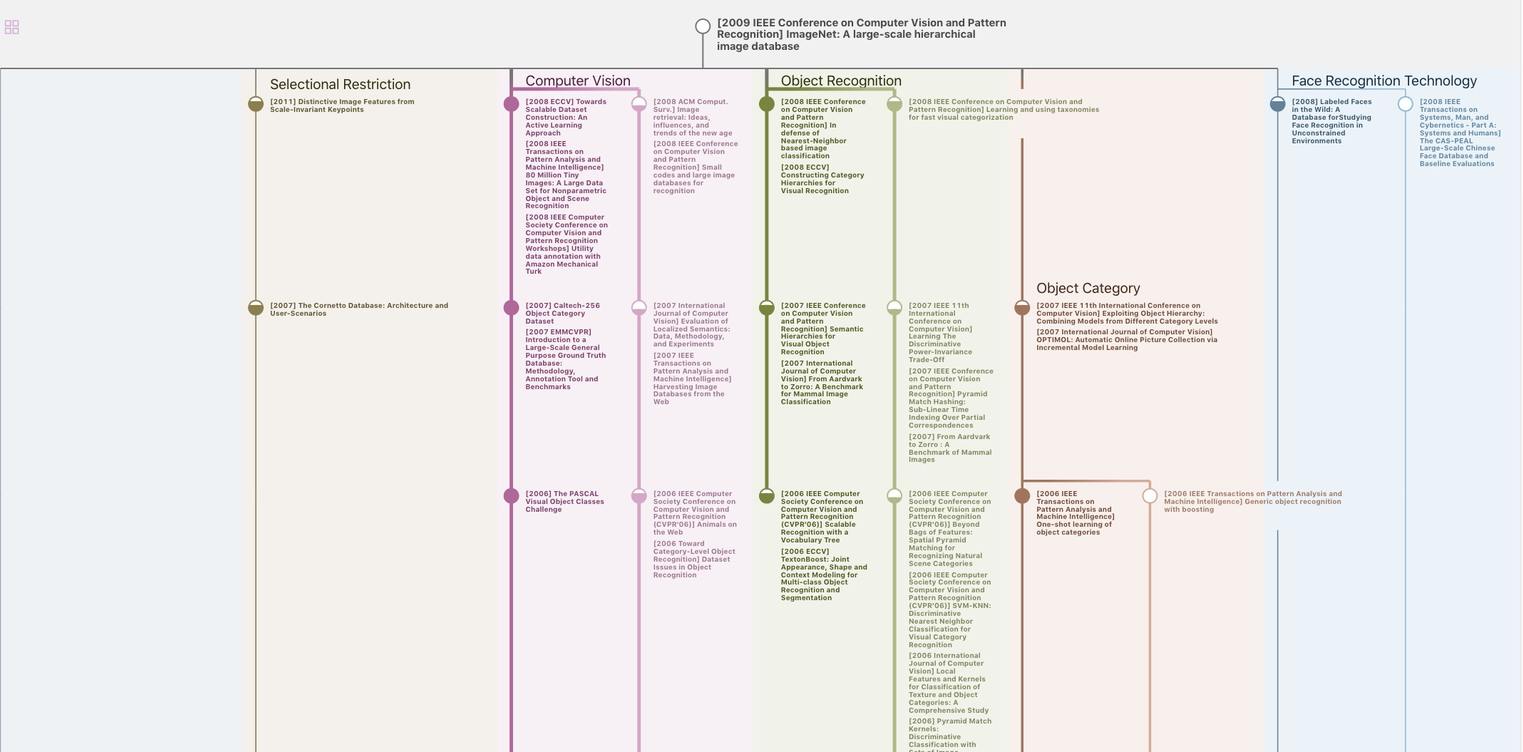
生成溯源树,研究论文发展脉络
Chat Paper
正在生成论文摘要