Mapping 30m China annual impervious surface from 1992 to 2021 via a multi-level classification approach
INTERNATIONAL JOURNAL OF REMOTE SENSING(2023)
摘要
Accurately mapping impervious surface has great significance and application for urbanization monitoring, urban sustainable planning, ecosystem protection, etc. In this study, an automatic and high-performance multi-level classification approach based on time-series similarity, spectral feature, and logical reasoning was proposed to accurately map China's annual impervious surface dataset (CAID) from 1992 to 2021 using Landsat time-series images. Firstly, the synthesized image of 30-year night-time light maximum value was used to mask the areas where humans are inactive, which will preliminarily screen out the impervious surface and mitigate the computational volume. Then, similarity measurement based on dynamic time warping was performed, respectively, for periodic index series, which were weighted by an amplitude scale factor. Furthermore, similarity measurement, spectral features, and logical reasoning of long-time-series land cover type were combined to construct a multi-level approach to mapping impervious surface. Finally, for the computational efficiency of pixel-level time-series classification, we built a distributed parallel high-performance computing framework to fully utilize local computing resources for efficiently developing CAID. Using 58,839 validation samples based on stratified random sampling, the overall accuracy and F1-score of CAID were 90.05% and 88.49%. A comparison with five existing 30 m impervious surface products further indicated that our CAID achieved better impervious surface extraction accuracy and was capable of finely delineating impervious surface landscapes. Therefore, it was concluded that our CAID is an accurate and credible product that can provide an important data base for urbanization and urban-related research in China.
更多查看译文
关键词
annual impervious surface,multi-level
AI 理解论文
溯源树
样例
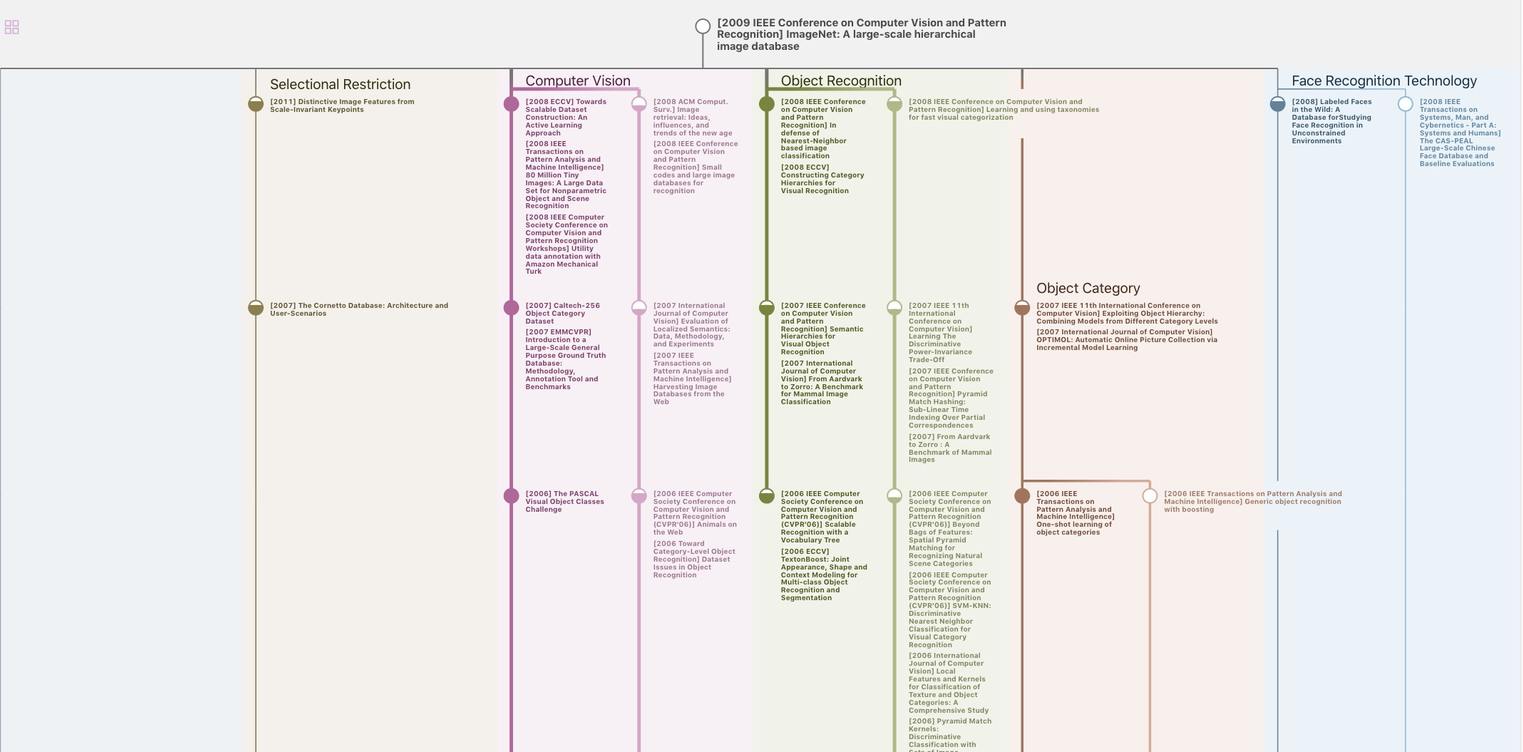
生成溯源树,研究论文发展脉络
Chat Paper
正在生成论文摘要