Fast fault detection for nonlinear interconnected systems via deterministic learning
TRANSACTIONS OF THE INSTITUTE OF MEASUREMENT AND CONTROL(2024)
摘要
In this paper, a fast fault detection scheme is developed for a class of nonlinear interconnected systems with output measurements. First, through combining an adaptive high gain observer with the deterministic learning theory, the system states and unknown dynamics are estimated simultaneously. However, large value of gain may let the estimator becomes noise sensitive. Thus, the observer structure is modified to avoid this issue. Second, by reusing the estimated knowledge which is stored in the constant radial basis function (RBF) neural networks, a bank of dynamic estimators are constructed. Then, the average L (1) norms of residuals are generated. The smallest residual principle is considered for decision-making in the detection phase. Third, the fault detection conditions and detection time are analyzed under the influence of observer gain. A simulation example is utilized to illustrate the effectiveness of this scheme.
更多查看译文
关键词
Fault detection,radial basis function neural networks,deterministic learning,adaptive high gain observer,persistent excitation condition,interconnected systems
AI 理解论文
溯源树
样例
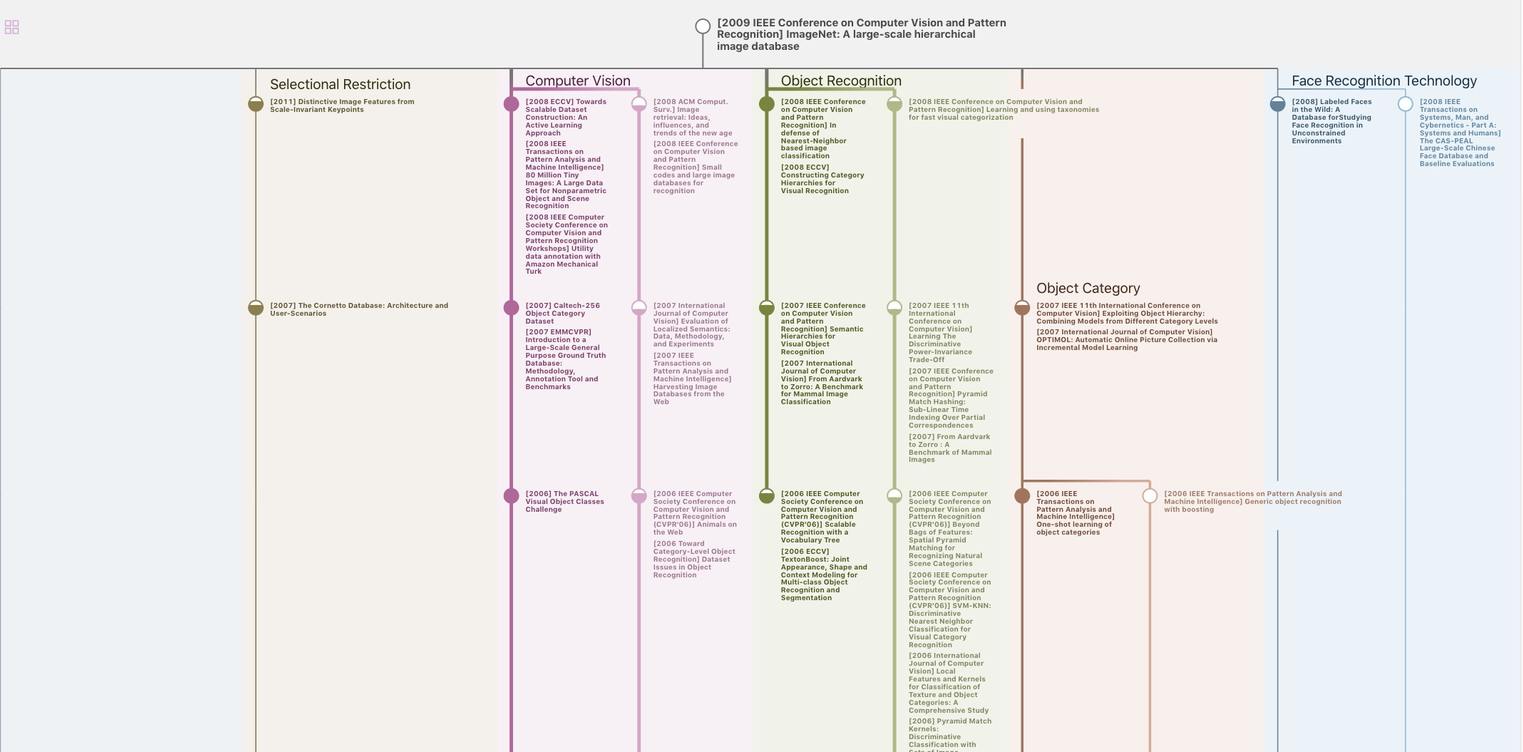
生成溯源树,研究论文发展脉络
Chat Paper
正在生成论文摘要