Virtual reference station technology for voxels without signal ray in ionospheric tomography based on machine learning
GPS SOLUTIONS(2023)
摘要
A new three-dimensional computerized ionospheric tomography model (VRS-ML-CIT) was developed in this study combining virtual reference station (VRS) and machine learning (ML) technology. Compared to the traditional VRS technology, the fitting and interpolation method generates virtual observations at each epoch. The ML technology was employed in this study to model both temporal and spatial variations of virtual observation in VRSs. Simultaneously, these ML-based VRSs are built associatively with unilluminated voxels (voxels without real observations) rather than relying solely on real reference stations, which can effectively reduce the proportion of unilluminated voxels, ensure the inversion efficiency, and improve the accuracy of virtual observations. We validate VRS-ML-CIT using observations of 153 GPS and two ionosonde stations in Europe. The results show that the test accuracy of the virtual observation is about 0.8 TECU, which offers an improvement of 40% over the previous non-machine learning VRS method. With the addition of virtual observations, the proportion of unilluminated voxels in VRS-ML-CIT declined from 30 to 12% on average. In comparison with the CIT result derived with only real observations (OBS-CIT), the error of the estimated slant total electron content in the proposed method reaches the same level for the illuminated voxels, even exceeding that of OBS-CIT in some periods. Moreover, the latitude–altitude maps and profiles of the IED from unilluminated voxels demonstrate the excellent performance of the proposed method.
更多查看译文
关键词
ionospheric tomography,virtual reference station technology,voxels,signal ray
AI 理解论文
溯源树
样例
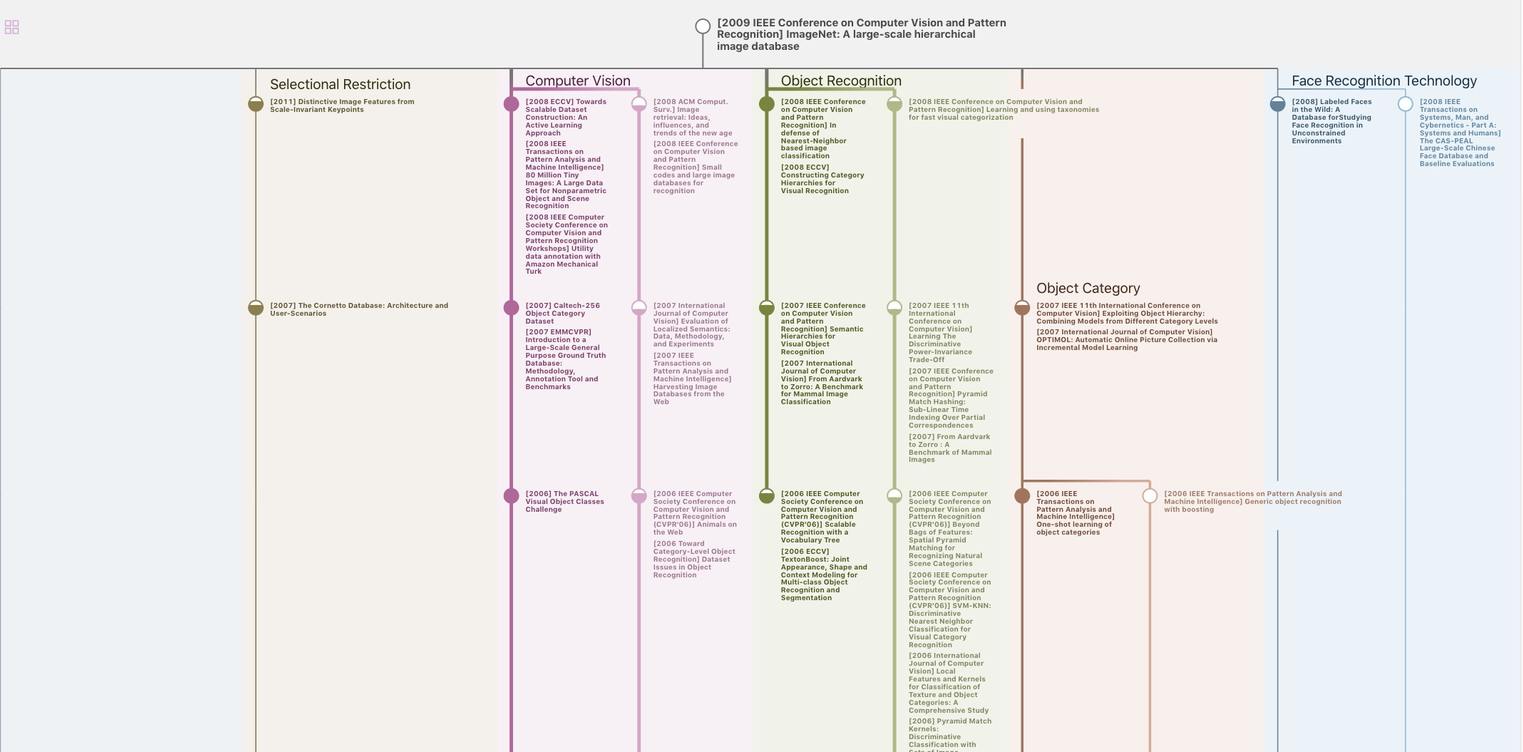
生成溯源树,研究论文发展脉络
Chat Paper
正在生成论文摘要