Multi-scale feature fusion for histopathological image categorisation in breast cancer
COMPUTER METHODS IN BIOMECHANICS AND BIOMEDICAL ENGINEERING-IMAGING AND VISUALIZATION(2023)
摘要
Histopathological images of the breast play a crucial role in diagnosing breast cancer and developing effective treatment strategies. However, the complexity of these images poses challenges for traditional convolutional neural networks, which struggle to extract all relevant features. In this paper, we present a novel classification method that addresses this limitation and enhances the accuracy of diagnosing benign and malignant breast tumors. Our approach involves data augmentation techniques, such as staining normalization, image patch generation, and spatial geometry transformation, to expand the training set. We utilize BCMNet (Breast Classification Fusion Multi-Scale Feature Network), which combines VGG16 and CBAM, to fuse spatial features, channel features, and multi-scale features of the input images. We evaluate our method on the BreakHis dataset and achieve average accuracies of 91.91%, 91.14%, 92.65%, and 87.56% for breast histopathology image classification at four different magnifications. Compared to the benchmark network VGG16 without data augmentation, our approach improves the highest accuracy by 3.69% and the average accuracy by 18.47%. Moreover, our proposed method achieves an F1 score of 95.02% and an average recognition accuracy of 92.20% at the patient level. Our approach surpasses previous breast pathology image classification methods, both single and hybrid networks, and establishes a strong theoretical foundation for computer-assisted breast cancer diagnosis.
更多查看译文
关键词
Breast cancer, histopathological images, convolutional neural network, feature fusion, data augmentation
AI 理解论文
溯源树
样例
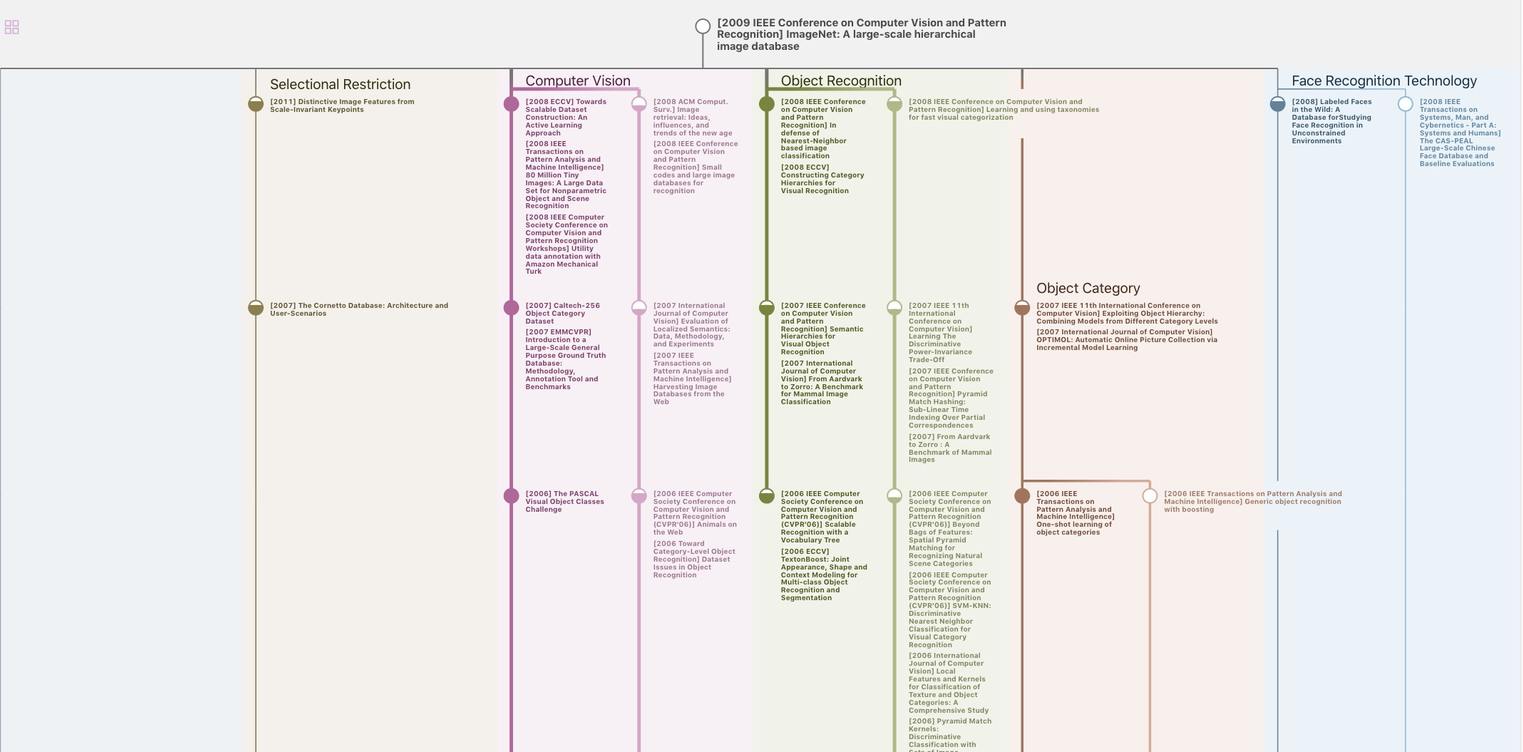
生成溯源树,研究论文发展脉络
Chat Paper
正在生成论文摘要