From resilience to Resistive Memory variability in Binarized Neural Networks to exploitation of variability in Bayesian Neural Network
2022 INTERNATIONAL CONFERENCE ON IC DESIGN AND TECHNOLOGY (ICICDT)(2022)
摘要
Resistive memory technologies are promising technologies for energy-efficient hardware implementation of intelligent systems that process information locally at the edge. However, current approaches are difficult to implement due to the intrinsic non-idealities of resistive memory. We present on the one hand binarized neural networks that are extremely resilient to errors and which operates at low energy consumption regimes and on the other hand a machine learning scheme that exploits memristor variability to implement Markov chain Monte Carlo sampling configured as a Bayesian machine learning model. Our approaches demonstrates robustness to device variability, degradation, and, based on circuit and systemlevel simulations, the total energy to perform a classification and to train models is estimated to be two order of magnitude lower than in complementary metal-oxide-semiconductor (CMOS)-based approaches.
更多查看译文
AI 理解论文
溯源树
样例
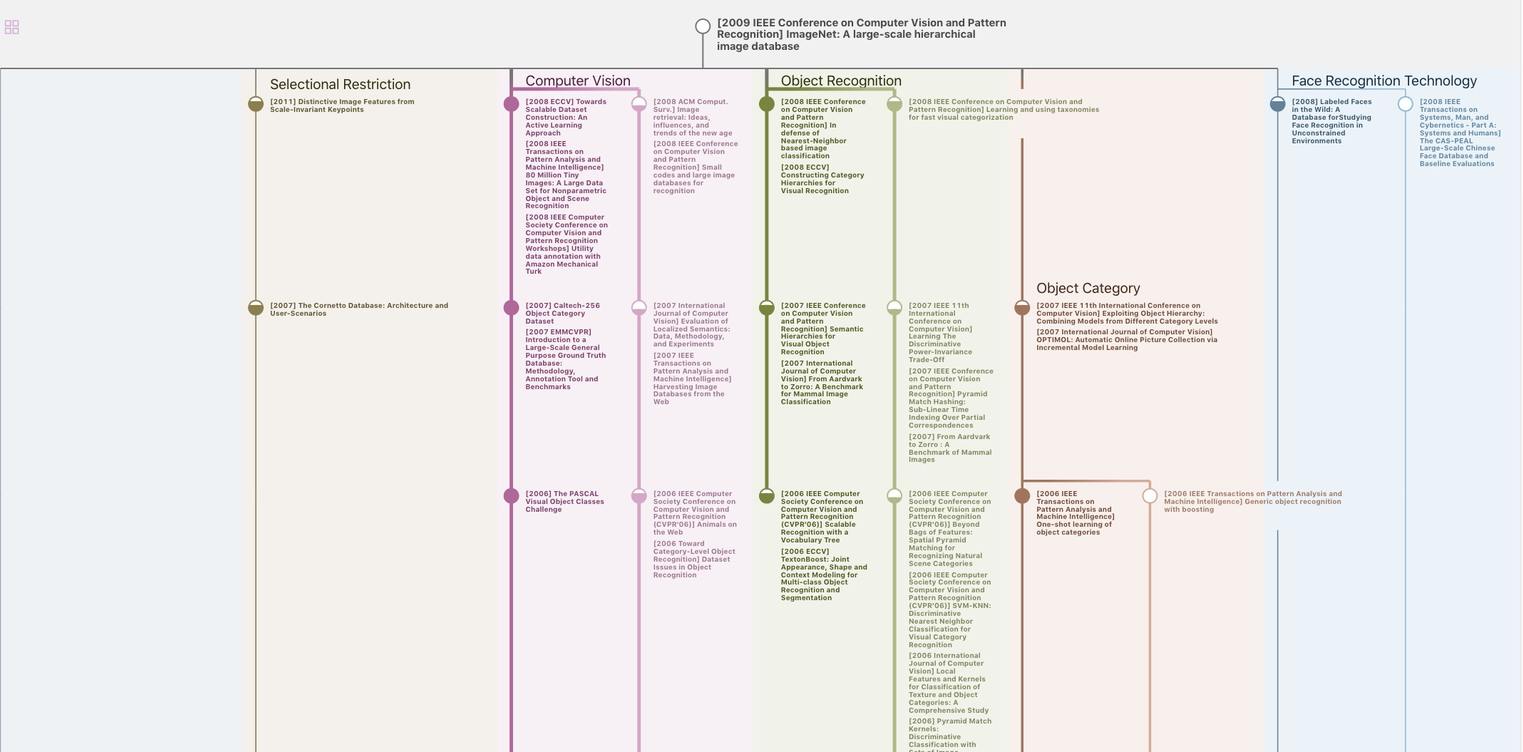
生成溯源树,研究论文发展脉络
Chat Paper
正在生成论文摘要