An Extendable Gaussian Mixture Model for Lane-Based Queue Length Estimation Based on License Plate Recognition Data
JOURNAL OF ADVANCED TRANSPORTATION(2022)
摘要
Most existing studies on queue length estimation based on license plate recognition (LPR) data require multisection LPR data. Studies based on single-section LPR data cannot ensure the accuracy and stability of the estimates when missed detections occur, which greatly limits the practicability of existing studies. Therefore, using single-section LPR data, this study proposes a lane-based queue length estimation method based on a two-dimensional Gaussian mixture model. First, the LPR data were processed to obtain the departure times and time headway of vehicles. Then, the two-dimensional Gaussian distributions of queued vehicles and nonqueued vehicles were fitted, and the expectation-maximization algorithm was adopted to solve the distribution parameters. Finally, the queuing status of each vehicle was determined, and the lane-based queue length was estimated based on the last identified queued vehicle in the cycle. The empirical results showed that the mean absolute errors (MAEs) of the proposed method were just 1.3 veh/cycle under no missed detections and 2 veh/cycle under a 20% missed detection rate, outperforming existing methods. The simulation results indicate that the proposed method can achieve accurate estimates under various traffic demands. In addition, the proposed method can be extended to real-time applications and multisection LPR systems.
更多查看译文
关键词
extendable gaussian mixture model,recognition,lane-based
AI 理解论文
溯源树
样例
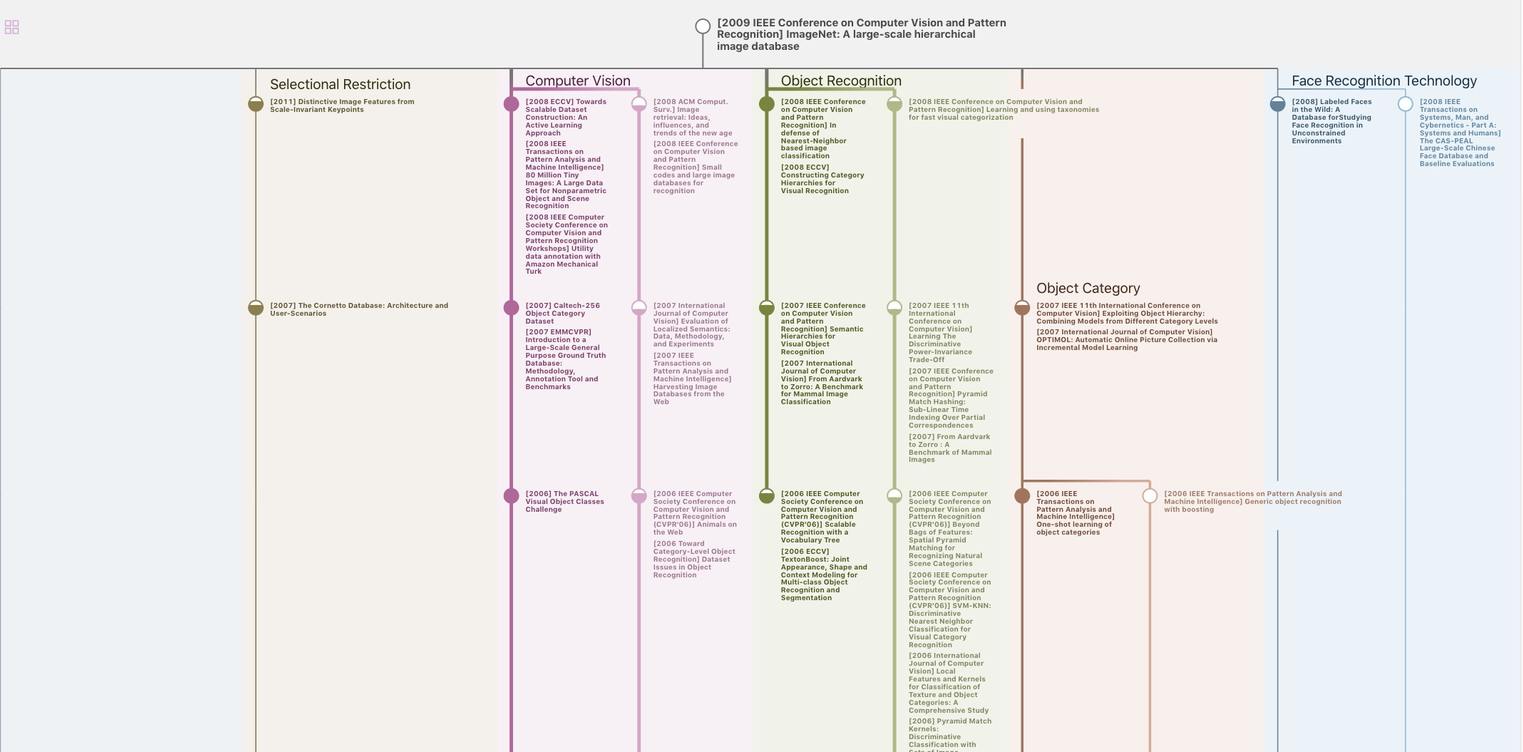
生成溯源树,研究论文发展脉络
Chat Paper
正在生成论文摘要