Adaptive self-propagation graph convolutional network for recommendation
WORLD WIDE WEB-INTERNET AND WEB INFORMATION SYSTEMS(2023)
摘要
Graph Convolutional Networks (GCNs) have received a lot of attention in recommender systems due to their powerful representation learning ability on graph data. Depending on whether to combine ego embeddings after aggregating neighbor embeddings, GCNs can be divided into two categories: without self-propagation and with self-propagation. (1) The GCNs without self-propagation bring the loss of inherent information (e.g., income, age) because of discarding ego embeddings. (2) The existing GCNs with self-propagation treat all nodes (i.e., users and items) indistinguishably, so that the distinctive and diverse characteristics of users and items are overlooked. In light of these problems existed in two types of GCNs, we propose a novel GCN model, Adaptive Self-propagation Graph Convolutional Network (ASP-GCN), to retain inherent information and distinctive characteristics of users and items simultaneously. We first conduct pilot experiments to prove that existing GCNs actually suffer from the aforementioned problems. Then, we use Gumbel-Softmax trick to generate categorical distributions for each node in each layer between two types of embeddings: neighborhood embeddings and hybrid embeddings. Neighborhood embeddings are the aggregation of neighbor embeddings and hybrid embeddings consist of ego and neighborhood embeddings. Next, user and item embeddings are updated by aggregating these two types of embeddings proportionally according to corresponding categorical distributions. After obtaining node embeddings in the last convolution layer, the Bayesian Personalized Ranking loss optimized with a similarity term is used to refine the model parameters. Comprehensive experiments are conducted on three benchmark datasets to demonstrate the effectiveness of ASP-GCN over present state-of-the-art approaches.
更多查看译文
关键词
Graph convolutional networks,Self-propagation,Gumbel-Softmax,Recommender systems
AI 理解论文
溯源树
样例
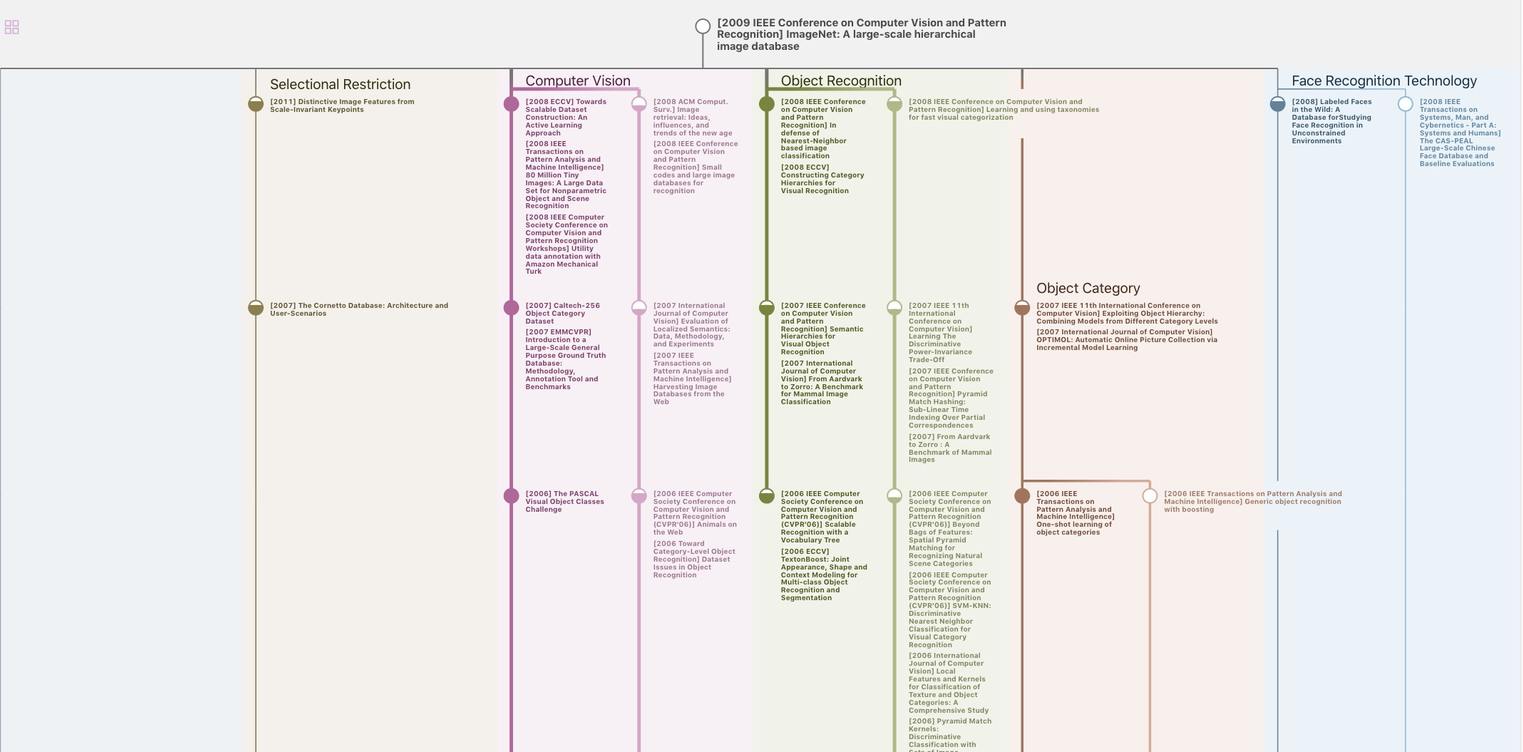
生成溯源树,研究论文发展脉络
Chat Paper
正在生成论文摘要