Inland Vessel Travel Time Prediction via a Context-Aware Deep Learning Model
JOURNAL OF MARINE SCIENCE AND ENGINEERING(2023)
摘要
Accurate vessel travel time estimation is crucial for optimizing port operations and ensuring port safety. Existing vessel travel time prediction models primarily rely on path-finding algorithms and corresponding distance/speed relationships to calculate travel time. However, these models overlook the complex nature of vessel travel time, which is influenced by multiple traffic-related factors such as collision avoidance, shortest path selection, and vessel personnel performance. The lack of consideration for these specific aspects limits the accuracy and applicability of current models. We propose a novel context-aware deep learning approach for inland vessel travel time prediction. Firstly, we introduce a complex network that captures vessel-vessel interaction contexts, providing valuable traffic environment information as an input for the deep learning model. Additionally, we employ a convolutional neural network to extract spatial trajectory information, which is then integrated with interaction contexts and indirect context information. In the vessel travel time prediction procedure, we utilize a long short-term memory network to capture the temporal dependence within consecutive channel sections' fused multiple context feature sets. Extensive experiments incorporating historical data from the Wuhan section of the Yangtze River in China demonstrate the superiority of our proposed model over classical models in predicting vessel travel time. Importantly, our model accounts for the specific traffic contexts that had previously been overlooked, leading to improved accuracy and applicability in inland vessel travel time prediction.
更多查看译文
关键词
inland river traffic, travel time prediction, complex network, deep learning network
AI 理解论文
溯源树
样例
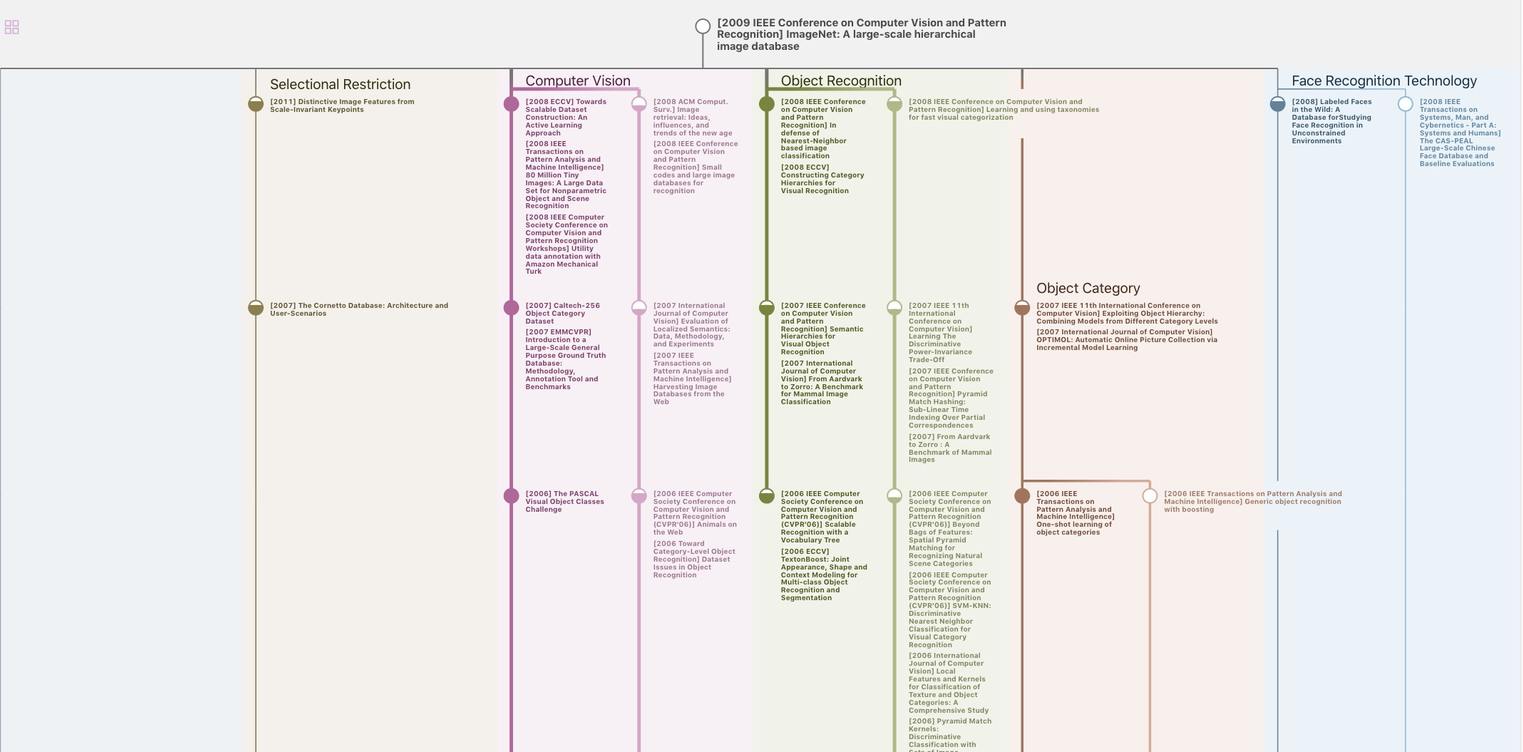
生成溯源树,研究论文发展脉络
Chat Paper
正在生成论文摘要