A machine-learning-based health diagnosis of polymer 3D-printed plates using the electromechanical impedance method
HEALTH MONITORING OF STRUCTURAL AND BIOLOGICAL SYSTEMS XVII(2023)
摘要
Additive manufacturing (AM) technology has been used for the creation of complex parts in different industries. The addition of defect detection and load sensing capabilities to these products can highly increase their values. Recently, modern industries have started incorporating AM components into their structures, including those with critical applications like aerospace and civil constructions. This requires the development of accurate and reliable methods for evaluating and monitoring the structural integrity of such components. The Electromechanical Impedance (EMI) method is frequently used to evaluate the health condition of lightweight structures based on the local structural response in the high-frequency range. This study investigates the usage of machine learning (ML) for the health-condition assessment of 3D-printed M3-X plates using EMI conductance (G) and resistance (R) data fusion. Piezoelectric wafers (PZTs) bonded to the center of the plates were used for the measurements. Drilled holes were created and repaired in multiple plates, and several EMI measurements were taken for the healthy, damaged, and repaired states of each plate. After fusing the R and G EMI measurement using a wide frequency range (1 kHz to 5 MHz), principal component analysis (PCA) was employed for feature reduction before a deep-learning approach was applied for diagnosis and damage classification. The findings demonstrate that the EMI method can be applied for the health assessment of AM polymers and is capable of differentiating between their healthy, damaged, and repaired states.
更多查看译文
关键词
Electromechanical impedance, 3D printing, data fusion, PCA, deep learning, classification
AI 理解论文
溯源树
样例
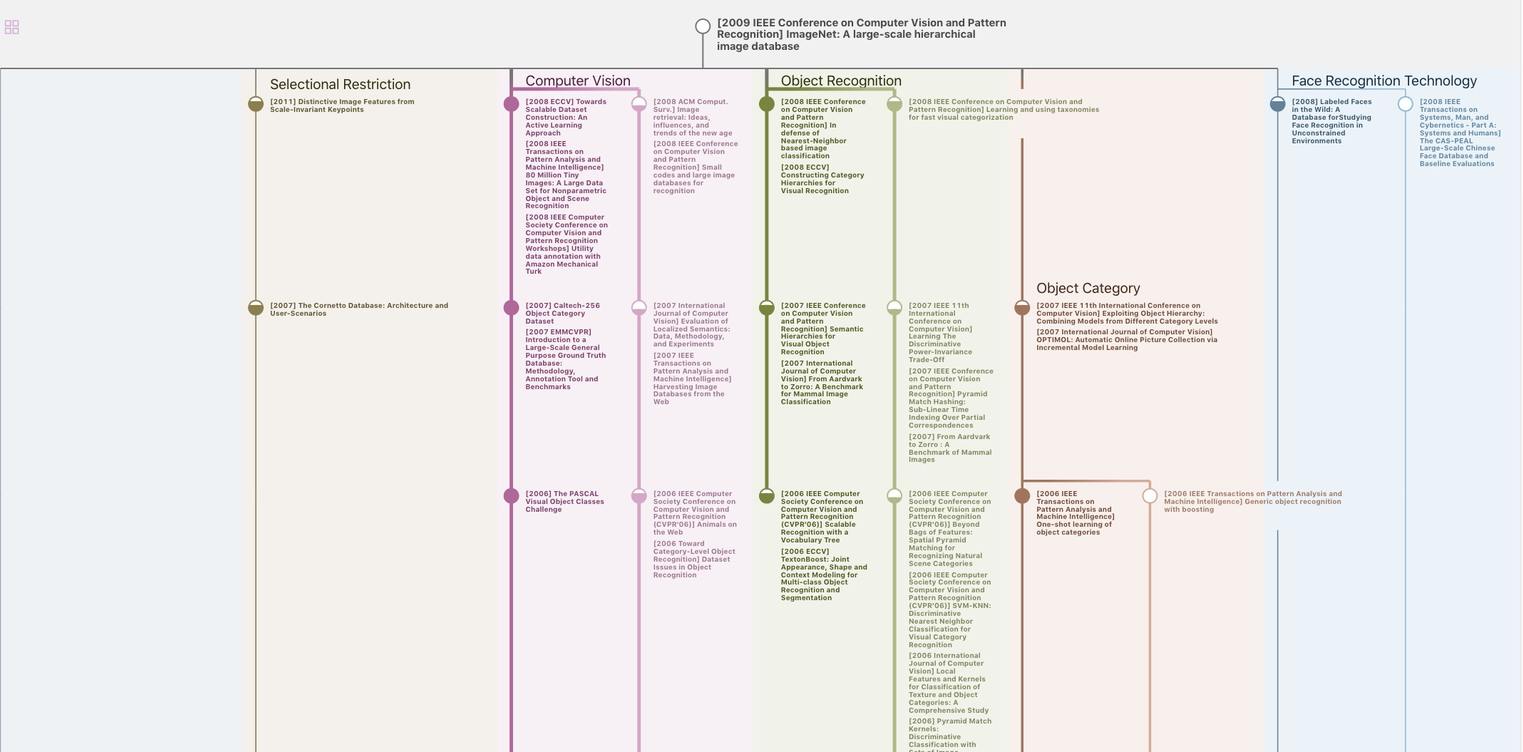
生成溯源树,研究论文发展脉络
Chat Paper
正在生成论文摘要