Spatial-Aware Multi-Directional Autoencoder For Pre-Training
COMPUTER JOURNAL(2024)
摘要
Vision Transformers for pre-trained models explore semantic context and spatial relationships for images, which heavily depend on how you select image patches. In this paper, we propose a novel Spatial-aware Multi-directional Patches Multi-cycle Autoencoder (SMPMA) for a pre-trained model that brings the following benefits: (1) Spatial-aware Multi-directional (SM) patches are created with multi-directional spatial locations, transforming a whole image autoencoder problem into a short-span image patches autoencoder problem; (2) SM patches admit a self-cycle autoencoder alignment learning for the first stage and a cross-cycle interaction learning for the second stage, which makes patches align and interact for optimization; (3) SM patches enable to explore local object features and correlation distribution of adjacent pixels by taking arbitrary sampled patches as inputs. Experimental results on four downstream tasks show that our model can achieve state-of-the-art performance over the tasks of image generation, image classification and semantic segmentation.
更多查看译文
关键词
vision transformers,multi-cycle autoencoder,vision tokens,alignment learning,interaction learning
AI 理解论文
溯源树
样例
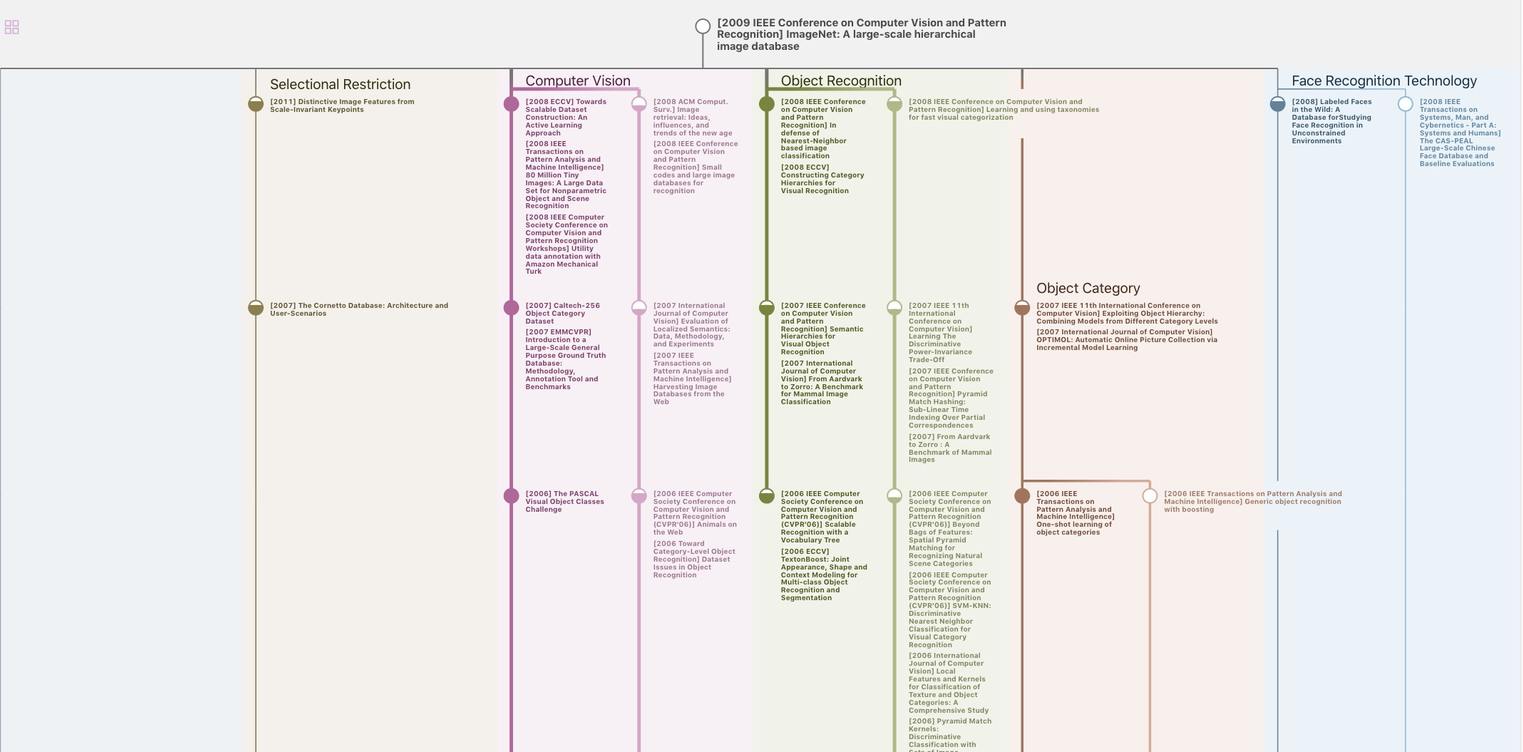
生成溯源树,研究论文发展脉络
Chat Paper
正在生成论文摘要