An Adaptive Asymmetric Loss Function for Positive Unlabeled Learning
AUTOMATIC TARGET RECOGNITION XXXIII(2023)
摘要
We introduce a new and efficient solution to the Positive and Unlabeled (PU) problem which is tailored specifically for a deep learning framework. We demonstrate the merit of this method using image classification. When only positive and unlabeled images are available for training, our custom loss function, paired with a simple linear transform of the output, results in an inductive classifier where no estimate of the class prior is required. This algorithm, known as the aaPU (Adaptive Asymmetric Positive Unlabeled) algorithm, provides near supervised classification accuracy with very low levels of labeled data on several image benchmark sets. aaPU demonstrates significant performance improvements over current state-of-the-art positive unlabeled learning algorithms.
更多查看译文
关键词
Positive Unlabeled, Semi-Supervised Learning, Deep Learning, Neural Nets, Classification
AI 理解论文
溯源树
样例
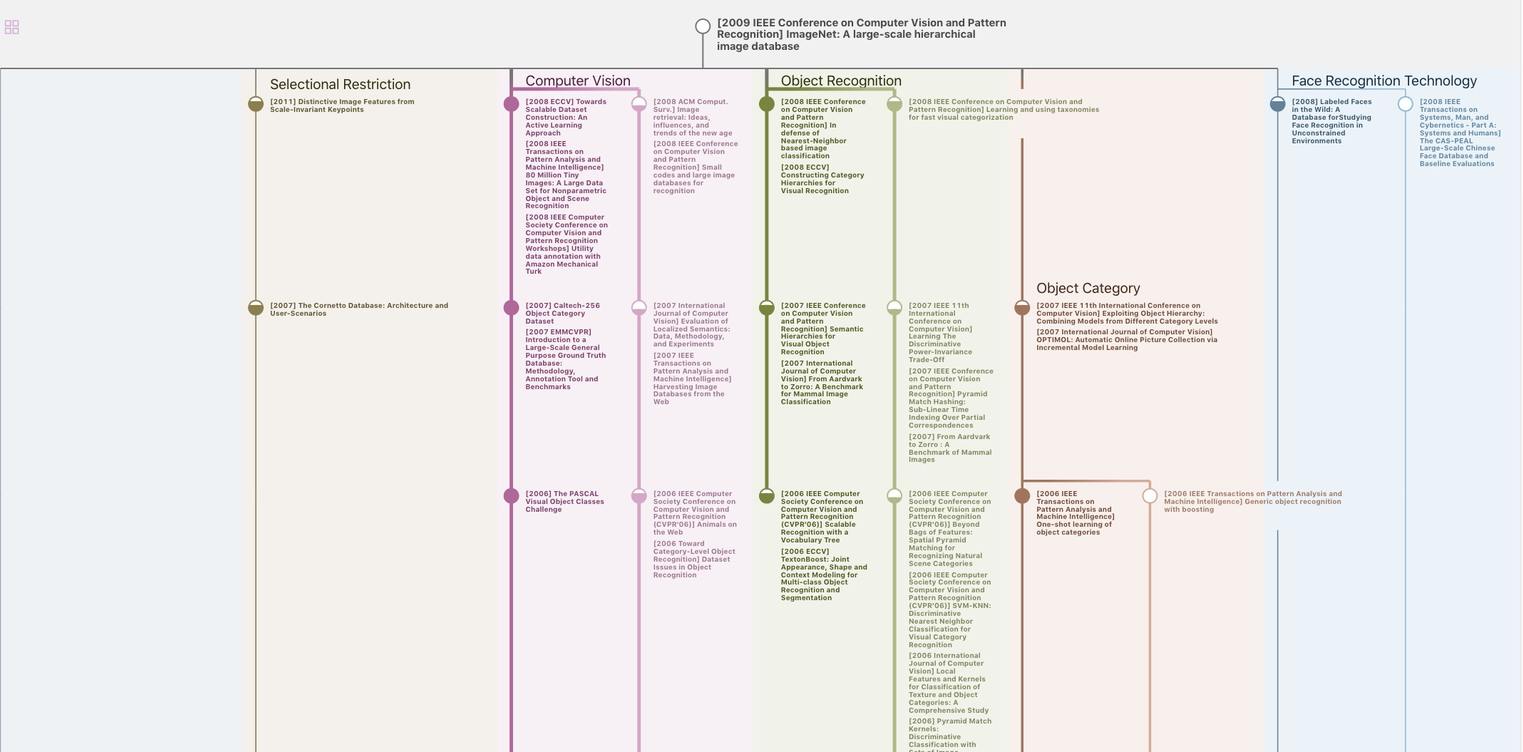
生成溯源树,研究论文发展脉络
Chat Paper
正在生成论文摘要