Customized EfficientNet for Histopathology Image Representation
2022 IEEE SYMPOSIUM SERIES ON COMPUTATIONAL INTELLIGENCE (SSCI)(2022)
摘要
In recent years, and amplified by the COVID-19 pandemic, the digitization of pathology has gained a considerable attention. Digital pathology provides crucial advantages compared to conventional light microscopy, including more efficient workflows, more accurate diagnosis and treatment planning, and easier collaboration. Despite promising progress, there are some critical challenges related to classifying images in digital pathology, such as huge input sizes (e.g., gigapixels) and expensive processing time. Most of the existing models for classification of histopathology images are very large and accordingly have many parameters to be learned/optimized. In addition, due to the large size of Whole Slide Images (WSIs), e.g., 100,000 x 100,000 pixels, models require enormous computational times to achieve reliable results. In order to address these challenges, we propose a more compact network which is customized to classify cancer subtypes with lower computation time and memory complexity. This model is based on EfficientNet topology, but it is tailored for classifying histopathology images. The utilized model is evaluated over three tumor types brain, lung, and kidney from The Cancer Genome Atlas (TCGA) public repository. Since the pre-trained EfficientNet works properly with the specific size of images, an effective approach is proposed to adjust the size of input images. The proposed model can be trained with a much smaller training set for applications such as image search that require robust and compact representations. The results show that the proposed model, compared to state-of-the-art models, i.e., KimiaNet, can classify cancer subtypes more accurately and provides superior results. In addition, the proposed model achieves memory and computational efficiency in the training phase and is a more compact deep topology compared to KimiaNet.
更多查看译文
关键词
EfficientNet, Patch Extraction, Histopathology Images, Classification, Image Search, KimiaNet, Image Representation, Deep Neural Network
AI 理解论文
溯源树
样例
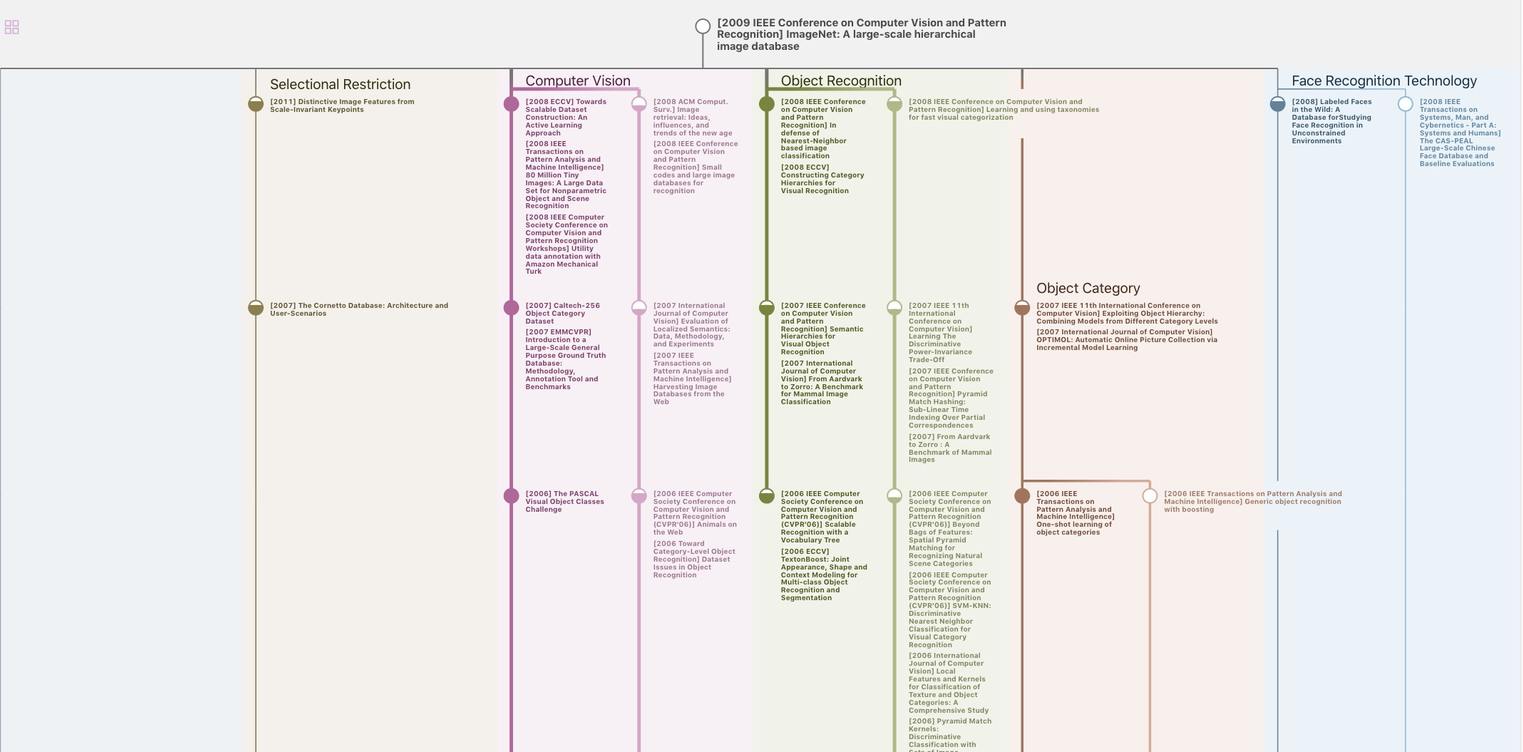
生成溯源树,研究论文发展脉络
Chat Paper
正在生成论文摘要