Monge blunts Bayes: Hardness Results for Adversarial Training
INTERNATIONAL CONFERENCE ON MACHINE LEARNING, VOL 97(2019)
摘要
The last few years have seen a staggering number of empirical studies of the robustness of neural networks in a model of adversarial perturbations of their inputs. Most rely on an adversary which carries out local modifications within prescribed balls. None however has so far questioned the broader picture: how to frame a resource-bounded adversary so that it can be severely detrimental to learning, a non-trivial problem which entails at a minimum the choice of loss and classifiers. We suggest a formal answer for losses that satisfy the minimal statistical requirement of being proper. We pin down a simple sufficient property for any given class of adversaries to be detrimental to learning, involving a central measure of "harmfulness" which generalizes the well-known class of integral probability metrics. A key feature of our result is that it holds for all proper losses, and for a popular subset of these, the optimisation of this central measure appears to be independent of the loss. When classifiers are Lipschitz - a now popular approach in adversarial training -, this optimisation resorts to optimal transport to make a low-budget compression of class marginals. Toy experiments reveal a finding recently separately observed: training against a sufficiently budgeted adversary of this kind improves generalization.
更多查看译文
AI 理解论文
溯源树
样例
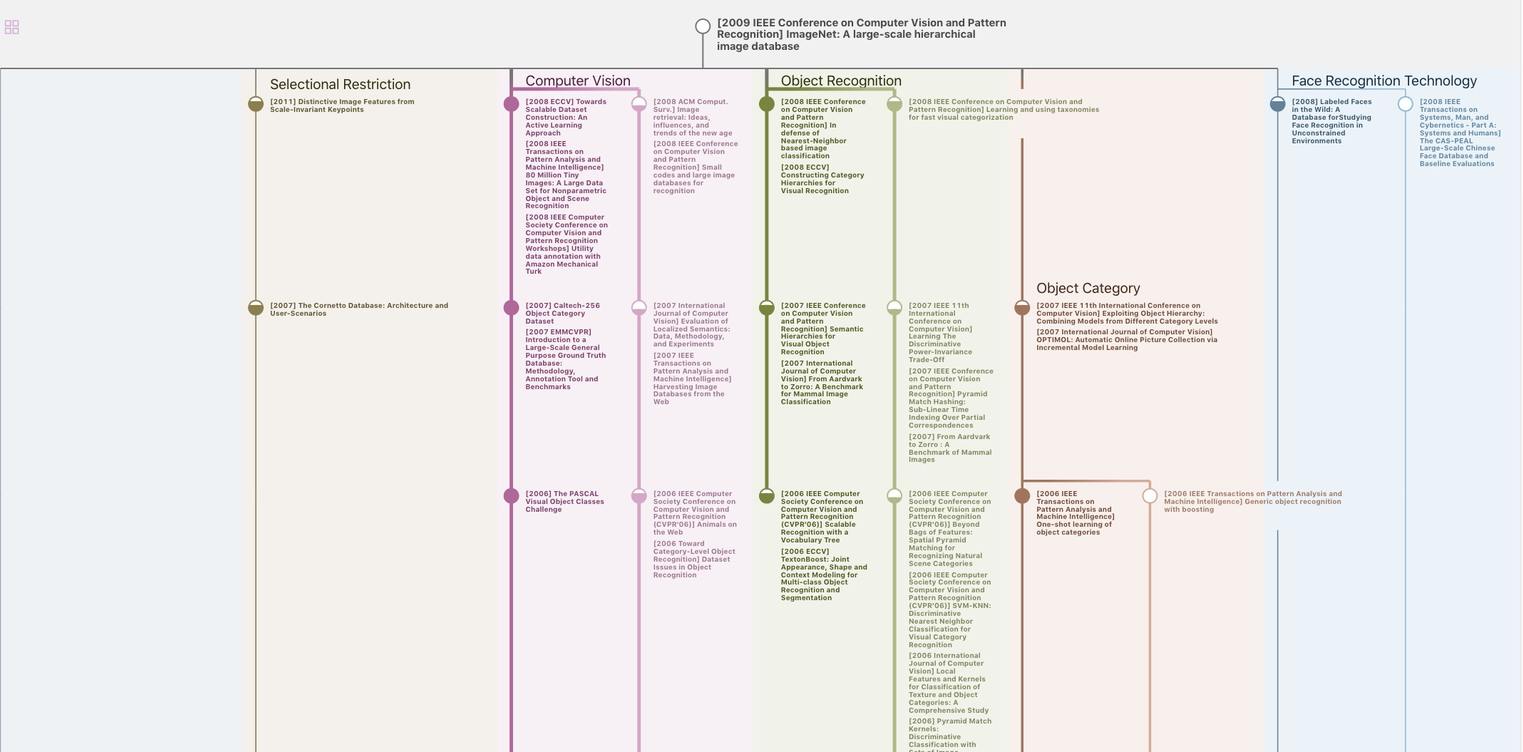
生成溯源树,研究论文发展脉络
Chat Paper
正在生成论文摘要