SMLFire1.0: a stochastic machine learning (SML) model for wildfire activity in the western United States
GEOSCIENTIFIC MODEL DEVELOPMENT(2023)
摘要
The annual area burned due to wildfires in the western United States (WUS) increased by more than 300% between 1984 and 2020. However, accounting for the non-linear, spatially heterogeneous interactions between climate, vegetation, and human predictors driving the trends in fire frequency and sizes at different spatial scales remains a challenging problem for statistical fire models. Here we introduce a novel stochastic machine learning (SML) framework, SMLFire1.0, to model observed fire frequencies and sizes in 12 km x 12 km grid cells across the WUS. This framework is implemented using mixture density networks trained on a wide suite of input predictors. The modeled WUS fire frequency matches observations at both monthly (r = 0:94) and annual (r = 0:85) timescales, as do the monthly (r = 0:90) and annual (r = 0:88) area burned. Moreover, the modeled annual time series of both fire variables exhibit strong correlations (r >= 0:6) with observations in 16 out of 18 ecoregions. Our ML model captures the interannual variability and the distinct multidecade increases in annual area burned for both forested and non-forested ecoregions. Evaluating predictor importance with Shapley additive explanations, we find that fire-month vapor pressure deficit (VPD) is the dominant driver of fire frequencies and sizes across the WUS, followed by 1000 h dead fuel moisture (FM1000), total monthly precipitation (Prec), mean daily maximum temperature (T-max), and fraction of grassland cover in a grid cell. Our findings serve as a promising use case of ML techniques for wildfire prediction in particular and extreme event modeling more broadly. They also highlight the power of ML-driven parameterizations for potential implementation in fire modules of dynamic global vegetation models (DGVMs) and earth system models (ESMs).
更多查看译文
关键词
wildfire activity,stochastic machine learning,machine learning
AI 理解论文
溯源树
样例
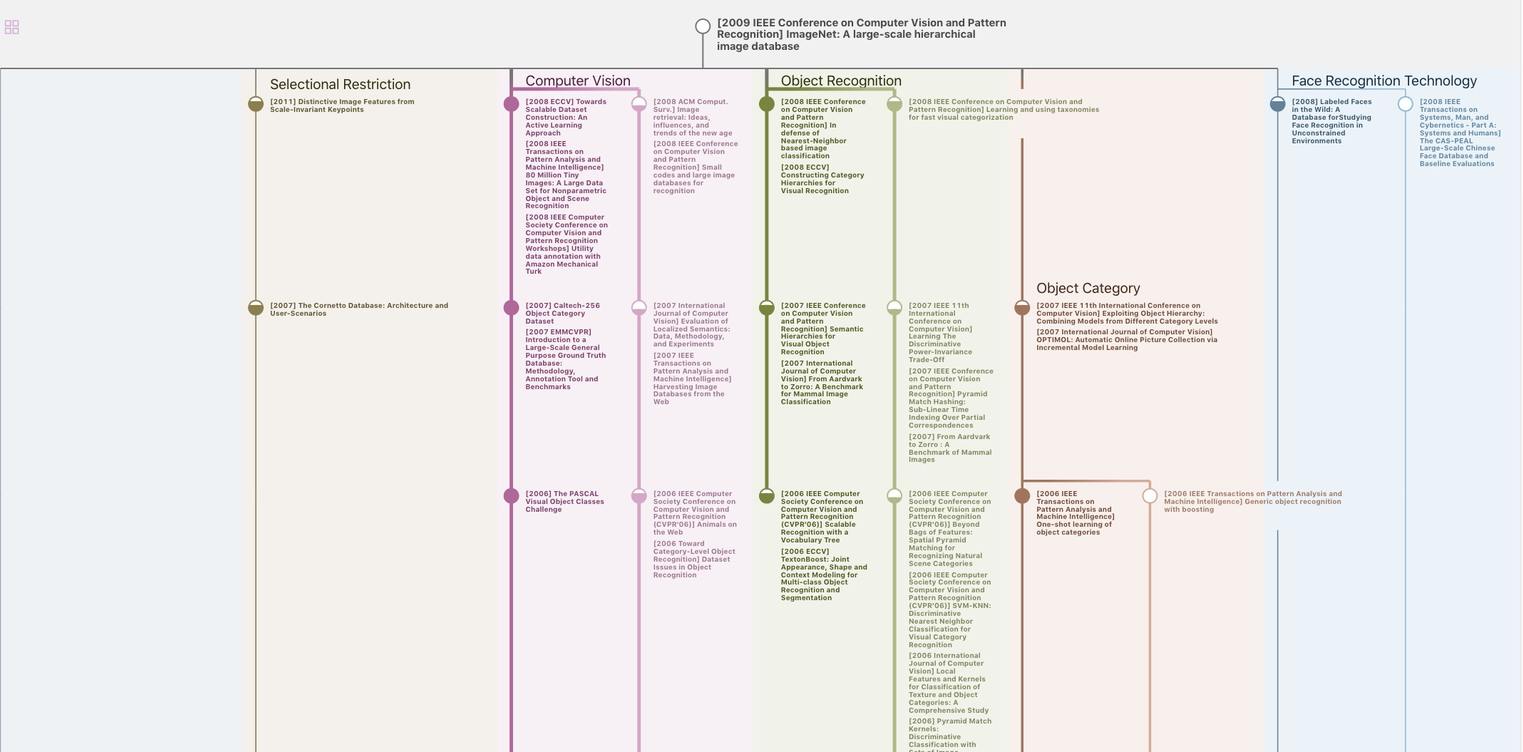
生成溯源树,研究论文发展脉络
Chat Paper
正在生成论文摘要