Empirical assessment of transformer-based neural network architecture in forecasting pollution trends
INTERNATIONAL JOURNAL OF DATA SCIENCE AND ANALYTICS(2023)
摘要
With rising pollution concerns in recent times, producing refined and accurate predictions as a part of United Nations Sustainable Development Goals 11 (sustainable cities and communities) and 13 (climate action) have gained utmost importance. As new model architectures become available, it becomes difficult for an average policymaker to evaluate various models comprehensively. One such architecture is the transformer neural network which has shown an exceptional rise in various areas such as natural language processing and computer vision. This paper explores the performance of such a transformer-based neural network (referred in the paper as PolTrans) in the domain of pollution forecasting. Experiments based on four univariate city pollution datasets (Delhi, Seoul, Skopje and Ulaanbaatar) and two multivariate datasets (Beijing PM _2.5 and Beijing PM _10 ) are performed against baselines consisting of widely used statistical, machine learning and deep learning methods. Findings show that although PolTrans performs comparatively better compared to existing deep learning methods such as bidirectional long short-term memory networks (LSTM), LSTM autoencoder, etc., for modelling pollution in cities such as Beijing, Delhi and Ulaanbaatar, in the majority of cases, the PolTrans architecture lags behind statistical and machine learning methods such as autoregressive integrated moving average (ARIMA), random forest regression, standard vector regression (SVR), etc., by a range of 1.5–15 units in terms of root mean square error.
更多查看译文
关键词
Time-series analysis, Statistical models, Deep learning, Air pollution, Pollution forecasting
AI 理解论文
溯源树
样例
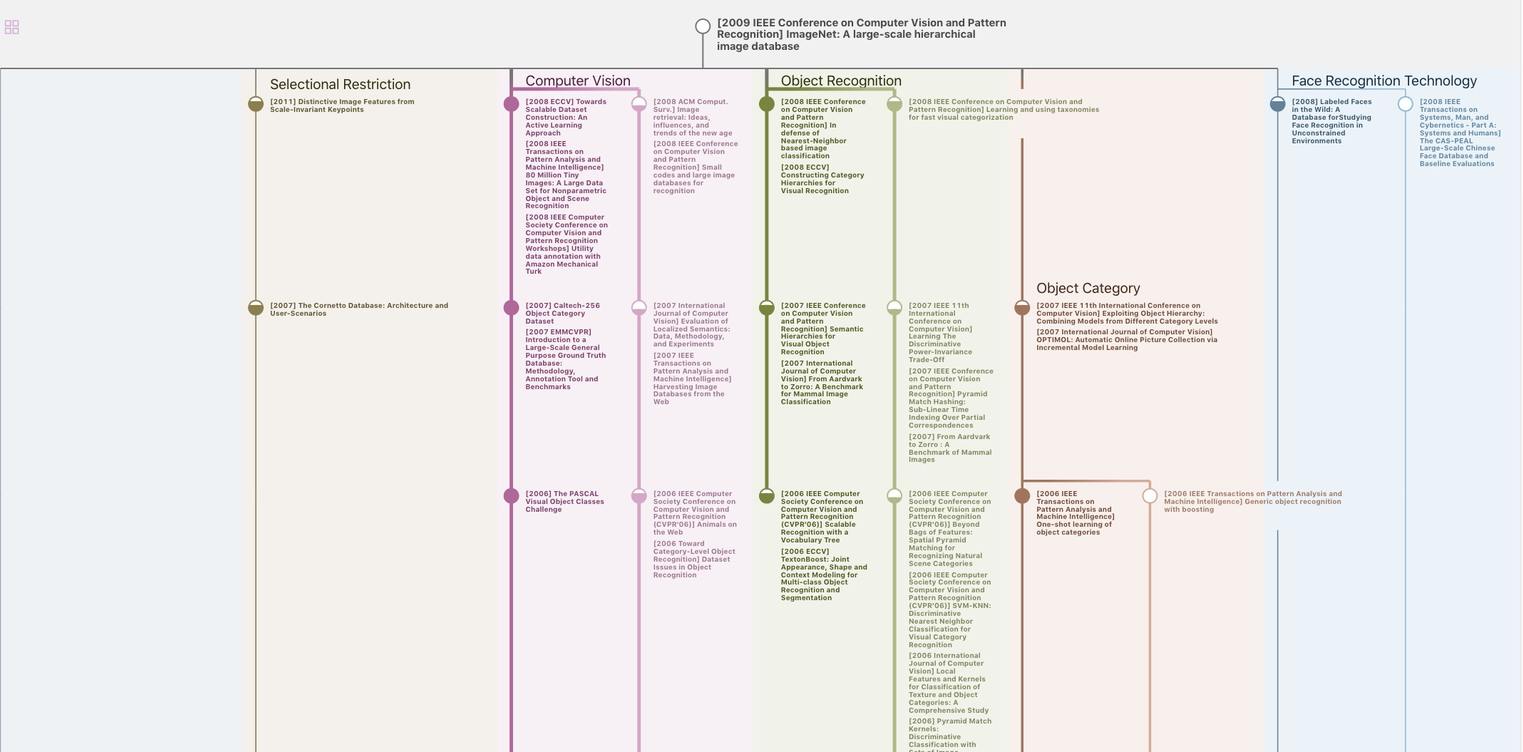
生成溯源树,研究论文发展脉络
Chat Paper
正在生成论文摘要