Development of Extractor-Classifier-Regulator integrated anomaly detection model for turning process
JOURNAL OF MANUFACTURING PROCESSES(2023)
摘要
Anomaly detection is important for safety and productivity in manufacturing systems. Together with the development in IoT, data-driven approach turns out as the most promising alternative for up-to-date anomaly detection. While applying modern A.I solutions into manufacturing for anomaly detection, however, it is often encountered that insufficient anomalous data is available for training deep-learning models. The most manufacturing systems are optimized to run at the best conditions so that anomalies rarely occur in the actual production lines. In this study, we proposed and developed an Extractor-Classifier-Regulator integrated anomaly detection model (ECR model) which combines the feature extractor, classifier and regulator in a block stacked way. This model requires the "normal" data only for training, and is capable of identifying the anomality based on the density of distribution in the extracted feature space. The framework described in the Section 2 yields that the ECR model is an end-to-end learning architecture, requires no pre-process for feature extraction and selec-tion. The performance of the proposed model was first verified in the Section 3, by use of the phase-shifted si-nusoidal waveforms generated artificially. The results suggested that our ECR model well yielded the degree of abnormality corresponding to the deviation of each variable from the "normal" datasets. The proposed model was then implemented into a turning process of Inconel 718 to detect cutting failure caused by tool wear. The experimental setup including machine tool, workpiece, tool holder, data acquisition system, time sequential signals related to the cutting process were detailed in the Section 4. After being trained by AE (Acoustic Emis-sion) or sound signals captured in the "normal" cutting stage, the proposed model was able to derive anomaly scores which well matched with the cutting stages of "normal", "transitional" and "anomalous" corresponding to the tool wear.
更多查看译文
关键词
anomaly detection model,extractor-classifier-regulator
AI 理解论文
溯源树
样例
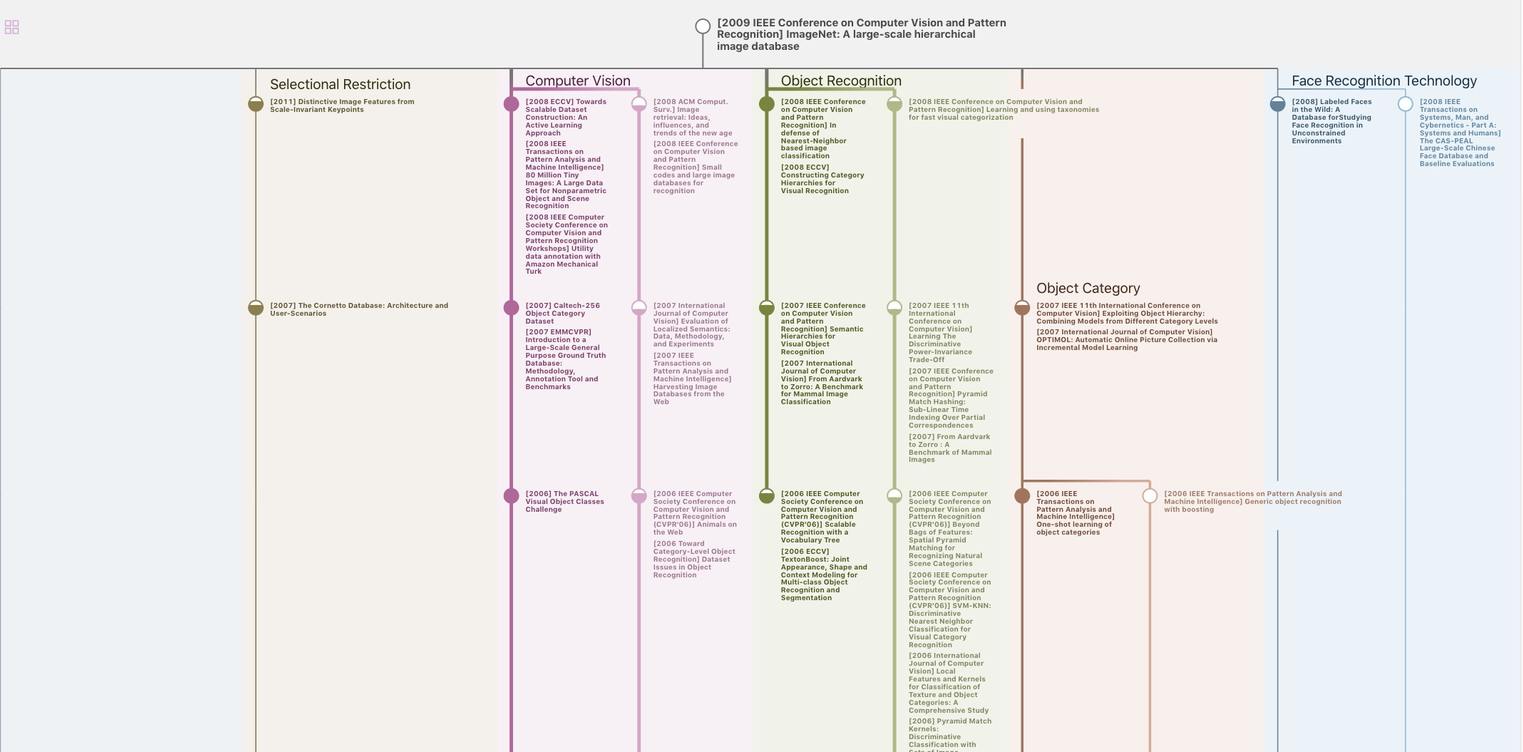
生成溯源树,研究论文发展脉络
Chat Paper
正在生成论文摘要