A computationally efficient Gibbs sampler based on data-augmentation strategy for estimating the reparameterized DINA model
COMMUNICATIONS IN STATISTICS-SIMULATION AND COMPUTATION(2023)
摘要
With the increasing demand for precise test feedback, cognitive diagnosis models (CDMs) have attracted more and more attention for fine classification of students with regard to their ability to master given skills. The aim of this paper is to use a highly effective Gibbs algorithm based on auxiliary variables (GAAV) to estimate the deterministic input noisy "and" gate (DINA) model that is widely used for cognitive diagnosis. The applicability of the algorithm to other CDMs is also discussed. Unlike the Metropolis-Hastings algorithm, this new algorithm does not require repeated adjustment of the turning parameters to achieve an appropriate acceptance probability, and it also overcomes the dependence of the traditional Gibbs sampling algorithm on the conjugate prior distribution. Four simulation studies are conducted, and a detailed analysis of fraction subtraction test data is carried out to further illustrate the proposed methodology.
更多查看译文
关键词
Bayesian inference, Cognitive diagnosis, Gibbs algorithm based on auxiliary variable, Metropolis-Hastings algorithm, Potential scale reduction factor, Reparameterized DINA model
AI 理解论文
溯源树
样例
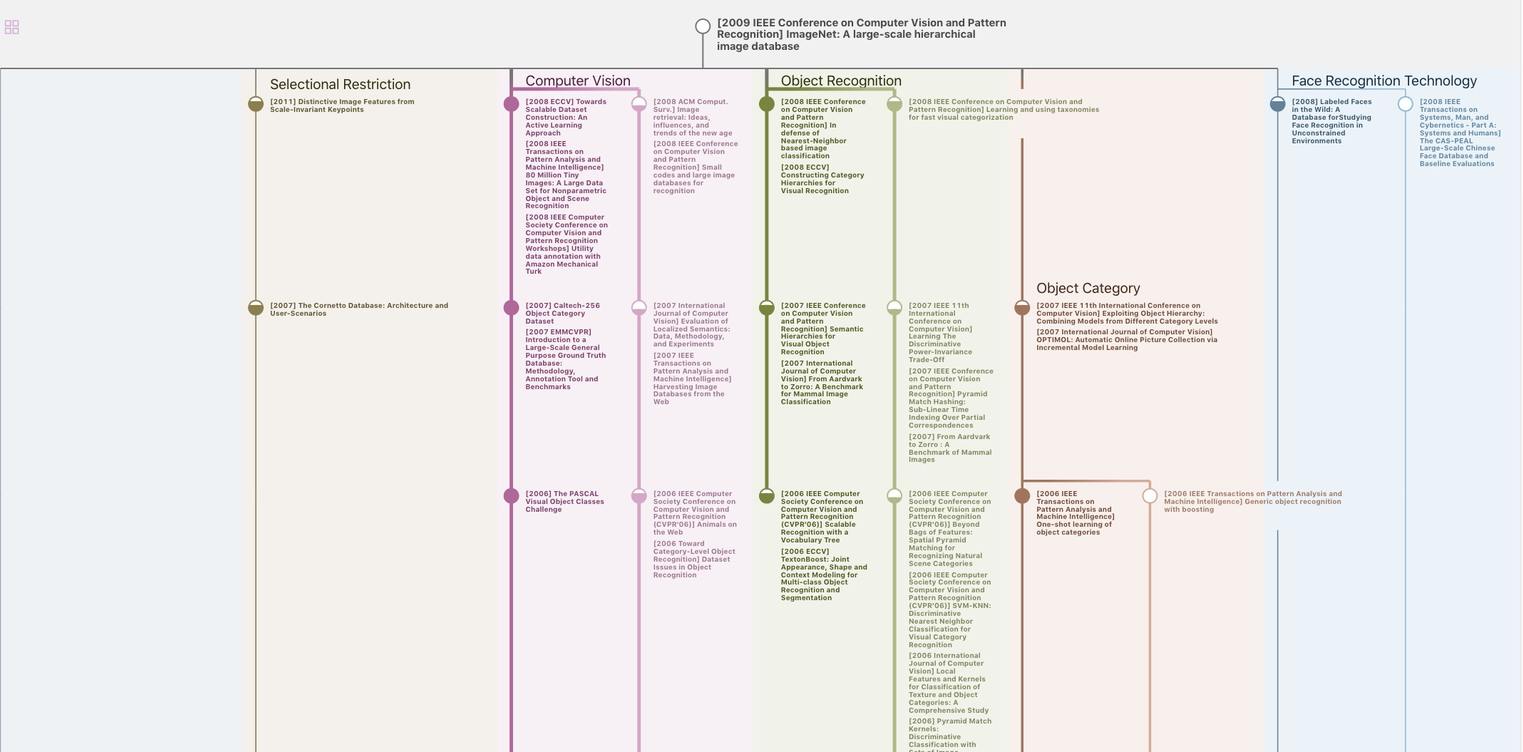
生成溯源树,研究论文发展脉络
Chat Paper
正在生成论文摘要