Injury Risk Assessment and Interpretation for Roadway Crashes Based on Pre-Crash Indicators and Machine Learning Methods
APPLIED SCIENCES-BASEL(2023)
摘要
Pre-crash injury risk (IR) assessment is essential for guiding efforts toward active vehicle safety. This work aims to conduct crash severity assessment using pre-crash information and establish the intrinsic mechanism of IR with proper interpretation methods. The impulse-momentum theory is used to propose novel a priori formulations of several severity indicators, including velocity change (& UDelta;V), energy equivalent speed (EES), crash momentum index (CMI), and crash severity index (CSI). Six IR models based on different machine learning methods were applied to a fusion dataset containing 24,082 vehicle-level samples. Prediction results indicate that the pre-crash indicators (PCIs) are more influential than the commonly used basic crash information because the average accuracy of six models can be improved by 14.35% after utilizing PCIs. Furthermore, the features' importance and their marginal effects are interpreted based on parameter estimation, Shapley additive explanation value, and partial dependence. The & UDelta;V, EES, and CMI are identified as the determinant indicators of the potential IR, and their partial distributions are significantly influenced by the crash type and impact position. Based on partial dependence probabilities, the study establishes decision thresholds for PCIs for each severity category for different impact positions, which can serve as a useful reference for developing targeted safety strategies. These results suggest that the proposed method can effectively improve pre-crash IR assessment, which can be readily transferred to safety-related modeling in an active traffic management system.
更多查看译文
关键词
injury risk assessment,a priori crash analysis,crash severity,machine learning,model interpretation
AI 理解论文
溯源树
样例
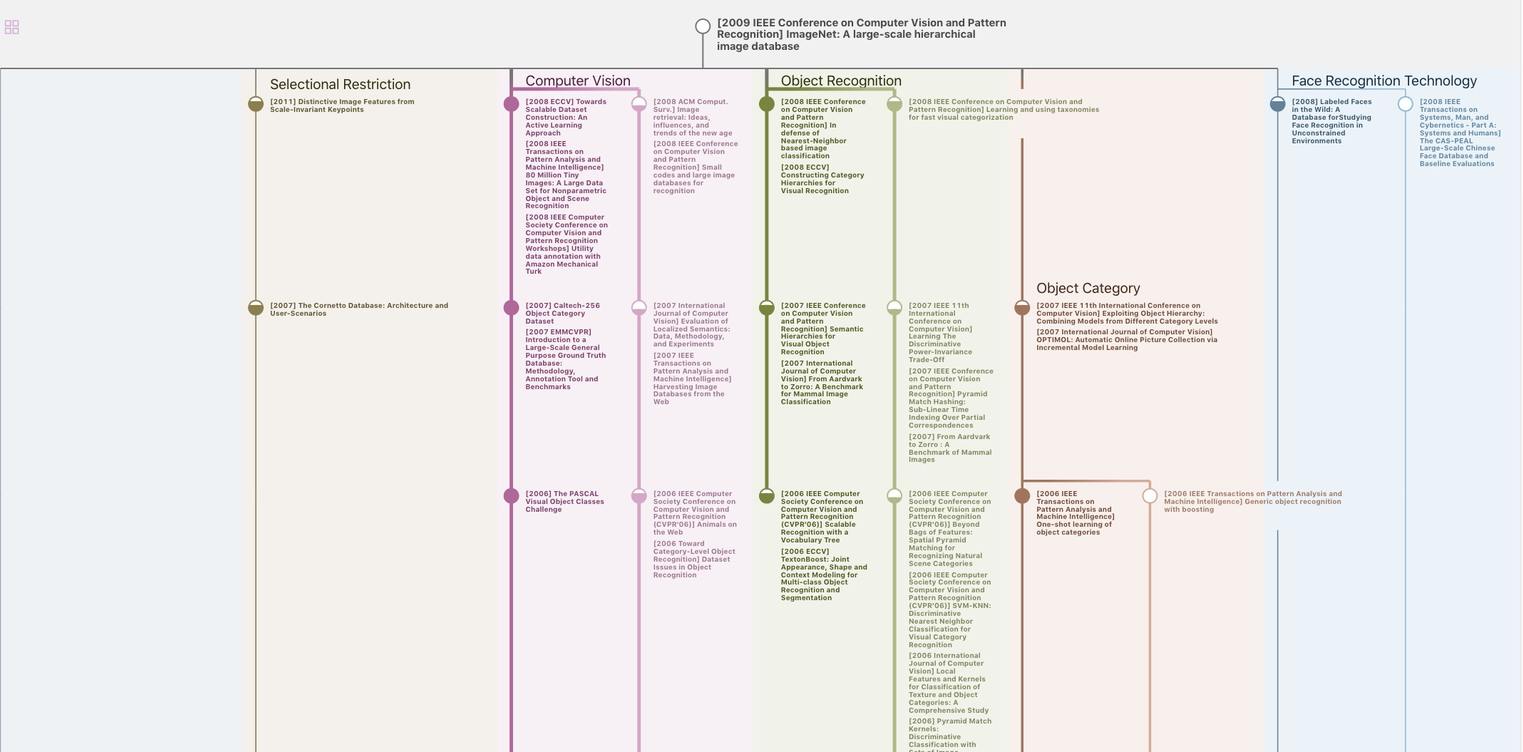
生成溯源树,研究论文发展脉络
Chat Paper
正在生成论文摘要