Real-Time Intersection Turning Movement Flow Forecasting Using a Parallel Bidirectional Long Short-Term Memory Neural Network Model
TRANSPORTATION RESEARCH RECORD(2024)
摘要
Real-time forecasting of intersection turning movements is a critical requirement for predictive traffic signal control at signalized intersections. Several traffic flow forecasting models have been developed in the past; however, most of them have focused only on the road segment-level traffic instead of the turning movement flow (TMF) at intersections. Therefore, in this paper, we propose a new TMF forecasting model, which consists of a combination of two neural network models, namely the stacked bidirectional long short-term memory and the traditional multi-layer perceptron model; this combination will enable effective learning for both short- and long-term time-varying patterns. Moreover, extensive computational experiments, using two years of turning movement counts at 22 intersections in the city of Milton, Ontario, Canada, explore the performance advantage of the proposed model in comparison with several state-of-the-art base models for forecasting accuracy, robustness, and transferability.
更多查看译文
关键词
data and data science,artificial intelligence,deep learning,machine learning (artificial intelligence),operations,ITS,traffic flow theory and characteristics
AI 理解论文
溯源树
样例
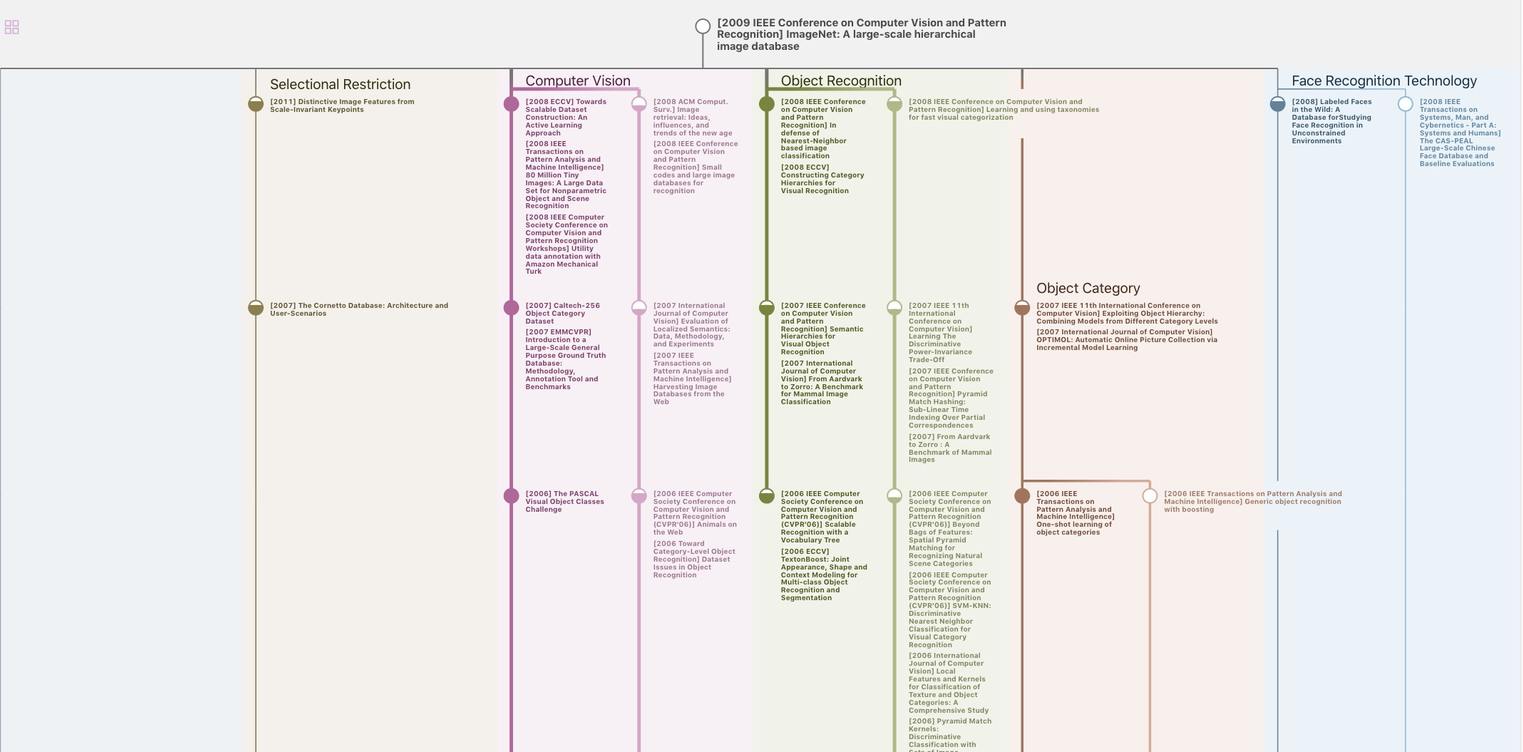
生成溯源树,研究论文发展脉络
Chat Paper
正在生成论文摘要