Fully Kernected Neural Networks
JOURNAL OF MATHEMATICS(2023)
摘要
In this paper, we apply kernel methods to deep convolutional neural network (DCNN) to improve its nonlinear ability. DCNNs have achieved significant improvement in many computer vision tasks. For an image classification task, the accuracy comes to saturation when the depth and width of network are enough and appropriate. The saturation accuracy will not rise even by increasing the depth and width. We find that improving nonlinear ability of DCNNs can break through the saturation accuracy. In a DCNN, the former layer is more inclined to extract features and the latter layer is more inclined to classify features. Therefore, we apply kernel methods at the last fully connected layer to implicitly map features to a higher-dimensional space to improve nonlinear ability so that the network achieves better linear separability. Also, we name the network as fully kernected neural networks (fully connected neural networks with kernel methods). Our experiment result shows that fully kernected neural networks achieve higher classification accuracy and faster convergence rate than baseline networks.
更多查看译文
关键词
neural networks,fully
AI 理解论文
溯源树
样例
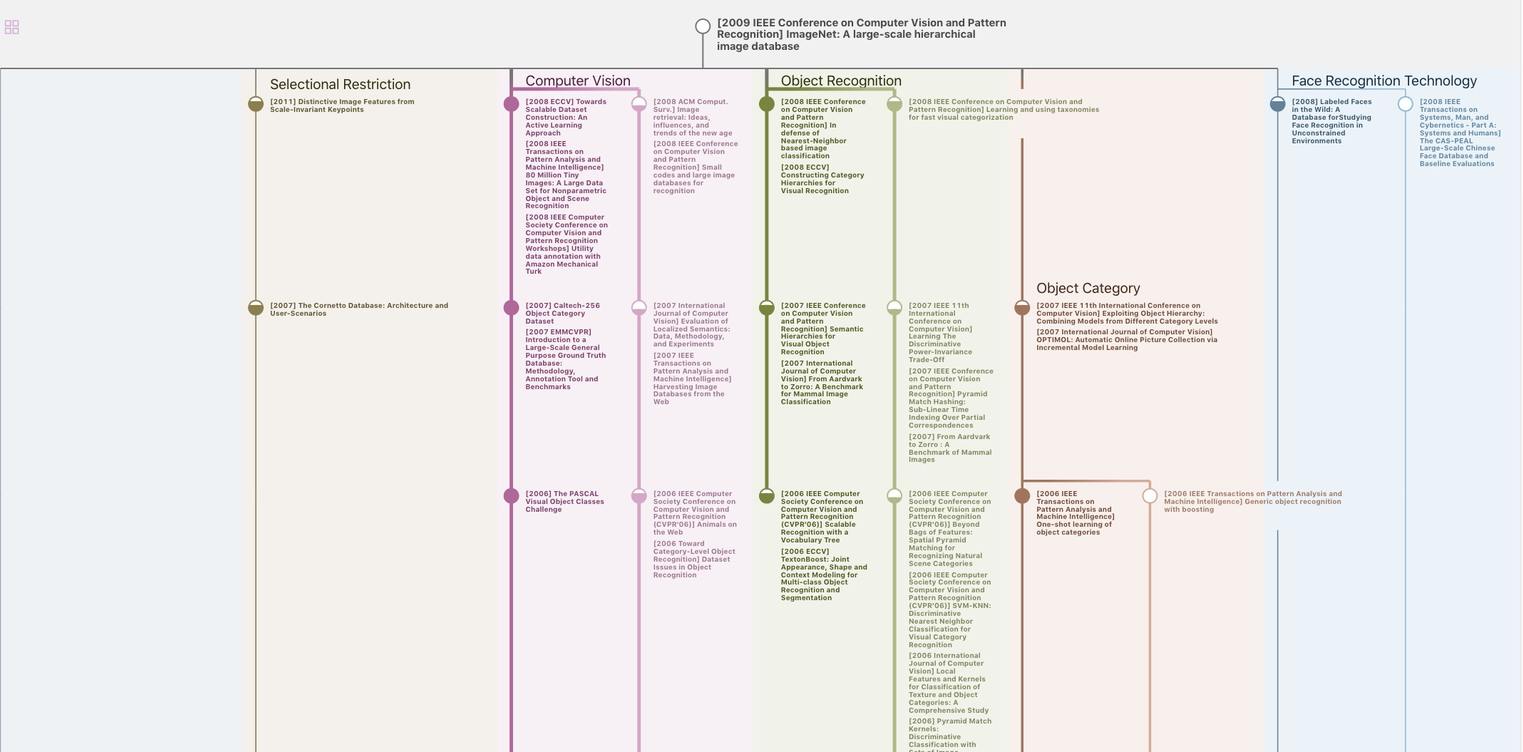
生成溯源树,研究论文发展脉络
Chat Paper
正在生成论文摘要